label = label.squeeze(1)
时间: 2023-06-23 15:09:33 浏览: 149
这行代码的作用是将标签(label)的维度从(batch_size,1)压缩为(batch_size,),也就是将多余的维度去掉,使得标签可以和预测值进行比较。在深度学习中,很多时候会使用交叉熵损失函数来计算模型预测值和真实标签之间的差距,而这个损失函数要求标签的维度必须与预测值的维度相同。因此,将标签的维度从(batch_size,1)压缩为(batch_size,)可以使得标签与预测值的维度一致,从而可以直接计算损失函数。
相关问题
python代码,问题代码是label = label.squeeze().cpu().detach().numpy()
根据您提供的代码,`label`的形状是(256,256,3),您可以使用`np.squeeze()`函数将其转换为(256,256),如下所示:
```
import numpy as np
import torch
# 假设label是您的标签张量
label = torch.rand(256, 256, 3)
label = label.squeeze().cpu().detach().numpy() # 将3维去掉,转换为numpy数组
label = np.squeeze(label) # 将数组的所有维度为1的轴都去掉,只留下(256,256)
print(label.shape)
```
这段代码首先将PyTorch的张量`squeeze()`成(256,256,3)的numpy数组,然后使用`numpy.squeeze()`函数去掉所有维度为1的轴,最终得到形状为(256,256)的numpy数组。
如果仍然报错,可以提供错误信息以便我们更好地了解问题。
这段代码有什么错误def forward(self,x): num_nodes = x.size(1) # sub_graph size batch_size = x.size(0) W = torch.cat([self.W] * batch_size, dim=0) representation = torch.matmul(x, W) r_sum = torch.sum(representation, dim=-1, keepdim=False) b = torch.zeros([batch_size, num_nodes]) b = Variable(b) one = torch.ones_like(r_sum) zero = torch.zeros_like(r_sum) label = torch.clone(r_sum) label = torch.where(label == 0, one, zero) b.data.masked_fill_(label.bool(), -float('inf')) num_iterations = 3 for i in range(num_iterations): c = torch.nn.functional.softmax(b, dim=-1) weight_coeff = c.unsqueeze(dim=1) representation_global = torch.matmul(weight_coeff, representation) representation_global_all = torch.cat([representation_global] * num_nodes, dim=1) representation_similarity = torch.nn.functional.cosine_similarity(representation, representation_global_all, dim=-1) representation_similarity.data.masked_fill_(label.bool(), -float('inf')) b = representation_similarity return representation_global.squeeze(dim=1)
这段代码中存在一个错误。在for循环中,代码更新了变量b,但是在更新后没有再次进行softmax操作,导致后续的计算结果不正确。因此,需要在for循环中,在更新b后再次进行softmax操作,如下所示:
```
for i in range(num_iterations):
c = torch.nn.functional.softmax(b, dim=-1)
weight_coeff = c.unsqueeze(dim=1)
representation_global = torch.matmul(weight_coeff, representation)
representation_global_all = torch.cat([representation_global] * num_nodes, dim=1)
representation_similarity = torch.nn.functional.cosine_similarity(representation, representation_global_all, dim=-1)
representation_similarity.data.masked_fill_(label.bool(), -float('inf'))
b = torch.nn.functional.softmax(representation_similarity, dim=-1) # 进行softmax操作
```
这样修改后,该段代码的功能应该能够正常运行。
阅读全文
相关推荐
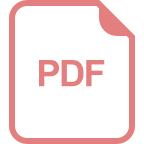
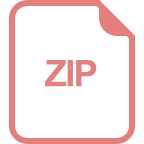
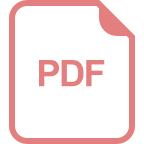













