P[i * self.ncol + j][a] = [(1, i * self.ncol + j, 0, True)]此行代码是什么意思
时间: 2024-02-15 19:43:41 浏览: 27
这行代码是将P数组中第i * self.ncol + j个状态下第a个动作的结果设置为一个包含四个元素的元组列表。其中元组的第一个元素1表示采取该动作的概率为1,第二个元素i * self.ncol + j表示采取该动作后智能体会转移到的下一个状态,第三个元素0表示执行该动作后智能体获得的奖励,第四个元素True表示执行该动作后智能体已经到达终止状态。由于智能体已经到达终止状态,因此该状态下任何动作的奖励都为0。
相关问题
import copy class CliffWalkingEnv: """ 悬崖漫步环境""" def __init__(self, ncol=9, nrow=5): self.ncol = ncol # 定义网格世界的列 self.nrow = nrow # 定义网格世界的行 # 转移矩阵 P[state][action] = [(p, next_state, reward, done)]包含下一个状态和奖励 self.P = self.createP() def createP(self): # 初始化 P = [[[] for j in range(4)] for i in range(self.nrow * self.ncol)] # 4 种动作, change[0]:上, change[1]:下, change[2]:左, change[3]:右。坐标系原点(0,0) # 定义在左上角 change = [[0, -1], [0, 1], [-1, 0], [1, 0]] for i in range(self.nrow): for j in range(self.ncol): for a in range(4): # 位置在悬崖或者目标状态, 因为无法继续交互,任何动作奖励都为 0 if i == self.nrow - 1 and j > 0: P[i * self.ncol + j][a] = [(1, i * self.ncol + j, 0, True)] continue # 其他位置 next_x = min(self.ncol - 1, max(0, j + change[a][0])) next_y = min(self.nrow - 1, max(0, i + change[a][1])) next_state = next_y * self.ncol + next_x reward = -1 done = False # 下一个位置在悬崖或者终点 if next_y == self.nrow - 1 and next_x > 0: done = True if next_x != self.ncol - 1: # 下一个位置在悬崖 reward = -100 P[i * self.ncol + j][a] = [(1, next_state, reward, done)] return P 将上述代码的每一行都进行注释并解释它在这个位置的作用
```python
import copy
class CliffWalkingEnv:
"""悬崖漫步环境"""
def __init__(self, ncol=9, nrow=5):
self.ncol = ncol # 定义网格世界的列
self.nrow = nrow # 定义网格世界的行
# 转移矩阵 P[state][action] = [(p, next_state, reward, done)]
# 包含下一个状态和奖励
self.P = self.createP()
def createP(self):
# 初始化
P = [[[] for j in range(4)] for i in range(self.nrow * self.ncol)]
# 4 种动作, change[0]:上, change[1]:下, change[2]:左, change[3]:右。坐标系原点(0,0)
# 定义在左上角
change = [[0, -1], [0, 1], [-1, 0], [1, 0]]
for i in range(self.nrow):
for j in range(self.ncol):
for a in range(4):
# 位置在悬崖或者目标状态, 因为无法继续交互,任何动作奖励都为 0
if i == self.nrow - 1 and j > 0:
P[i * self.ncol + j][a] = [(1, i * self.ncol + j, 0, True)]
continue
# 其他位置
next_x = min(self.ncol - 1, max(0, j + change[a][0]))
next_y = min(self.nrow - 1, max(0, i + change[a][1]))
next_state = next_y * self.ncol + next_x
reward = -1
done = False
# 下一个位置在悬崖或者终点
if next_y == self.nrow - 1 and next_x > 0:
done = True
if next_x != self.ncol - 1: # 下一个位置在悬崖
reward = -100
P[i * self.ncol + j][a] = [(1, next_state, reward, done)]
return P
```
代码的作用:
- `import copy`:导入 copy 模块
- `class CliffWalkingEnv:`:定义名为 CliffWalkingEnv 的类
- `def __init__(self, ncol=9, nrow=5):`:定义类的初始化函数,其中 ncol 和 nrow 分别表示网格世界的列数和行数
- `self.ncol = ncol`:将输入的 ncol 赋值给类内部的 self.ncol
- `self.nrow = nrow`:将输入的 nrow 赋值给类内部的 self.nrow
- `self.P = self.createP()`:将类的转移矩阵 P 初始化为 createP() 函数的返回值
- `def createP(self):`:定义 createP() 函数来生成转移矩阵 P
- `P = [[[] for j in range(4)] for i in range(self.nrow * self.ncol)]`:初始化 P 为空列表,其维度为 (ncol * nrow, 4),即每个状态都有四种动作
- `change = [[0, -1], [0, 1], [-1, 0], [1, 0]]`:定义 change 列表,其中每个元素表示一个动作的坐标变化,分别为上、下、左、右
- `for i in range(self.nrow):`:遍历网格世界的每一行
- `for j in range(self.ncol):`:遍历网格世界的每一列
- `for a in range(4):`:遍历四种动作
- `if i == self.nrow - 1 and j > 0:`:如果当前位置在悬崖或者终点,则任何动作奖励都为 0,此时将转移矩阵 P 的该状态和动作对应的值设为 [(1, i * self.ncol + j, 0, True)],表示只有该状态和动作概率为 1,下一个状态为当前状态,奖励为 0,终止状态为 True
- `next_x = min(self.ncol - 1, max(0, j + change[a][0]))`:计算当前动作后下一个状态的横坐标
- `next_y = min(self.nrow - 1, max(0, i + change[a][1]))`:计算当前动作后下一个状态的纵坐标
- `next_state = next_y * self.ncol + next_x`:计算下一个状态的编号
- `reward = -1`:将奖励初始化为 -1
- `done = False`:将终止状态标志初始化为 False
- `if next_y == self.nrow - 1 and next_x > 0:`:如果下一个状态是终点,则将终止状态标志设为 True
- `if next_x != self.ncol - 1:`:如果下一个状态不是悬崖,则将奖励设为 -100
- `P[i * self.ncol + j][a] = [(1, next_state, reward, done)]`:将该状态和动作对应的转移概率、下一个状态、奖励、终止状态设为 [(1, next_state, reward, done)],表示只有该状态和动作概率为 1,下一个状态为 next_state,奖励为 reward,终止状态为 done
- `return P`:返回生成的转移矩阵 P
def reset(self): # 回归初始状态,坐标轴原点在左上角 self.x = 0 self.y = self.nrow - 1 return self.y * self.ncol + self.x 中,return self.y * self.ncol + self.x是什么作用
在reset函数中,self.y * self.ncol + self.x的作用是计算智能体回归初始状态后的状态编号。
在该环境中,智能体的初始位置是在左上角,即坐标轴原点。当调用reset函数时,将智能体的横坐标self.x设置为0,纵坐标self.y设置为self.nrow - 1,即回到了初始位置。
然后,通过计算self.y * self.ncol + self.x,可以将智能体的当前位置转化为一个唯一的状态编号。这个状态编号可以用来表示智能体在环境中的状态。
最后,reset函数返回计算得到的状态编号,表示智能体回归初始状态后的状态。
相关推荐
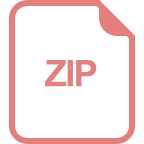
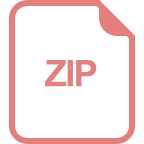













