features = np.concatenate(features, axis=0)
时间: 2023-02-24 22:55:08 浏览: 194
这行代码是在使用 Numpy 库中的 np.concatenate() 函数,将一个列表的特征连接在一起。"features" 是一个 Numpy 数组,"axis=0" 表示在纵向(沿着行)方向连接特征。结果是一个更大的数组,其中包含了原来列表中的所有特征。
相关问题
sinusoids = np.concatenate((sinusoids, sinusoid_features), axis=1)
`np.concatenate()` 函数用于在给定的轴(这里是 `axis=1`)上连接两个数组。在这个上下文中,`sinusoids` 和 `sinusoid_features` 是两个沿着列(features)方向的数据集,通过调用这个函数,它们会被合并成一个新的数组,新的数组会在原来 `sinusoids` 的每一行后面添加 `sinusoid_features` 的对应行。
具体操作如下:
```python
# 假设 sinusoids 是原始特征数据
sinusoids = ... # 原始 sinusoide 数据
# sinusoid_features 是新增加的特征数据
sinusoid_features = ... # 新增特征数据
# 使用 np.concatenate 操作
concatenated_data = np.concatenate((sinusoids, sinusoid_features), axis=1)
# 结果是一个新的数组,其中原 `sinusoids` 的每一行都有新增的 `sinusoid_features` 行
```
这样做的目的是为了扩展模型输入,可能是因为要引入额外的特征来改进模型的表现。
def get_rolling_window_multistep(forecasting_length, interval_length, window_length, features, labels): output_features = np.zeros((1, features.shape[0], window_length)) output_labels = np.zeros((1, 1, forecasting_length)) if features.shape[1] != labels.shape[1]: assert 'cant process such data' else: output_features = np.zeros((1, features.shape[0], window_length)) output_labels = np.zeros((1, 1, forecasting_length)) for index in tqdm.tqdm(range(0, features.shape[1]-interval_length-window_length-forecasting_length+1), desc='data preparing'): output_features = np.concatenate((output_features, np.expand_dims(features[:, index:index+window_length], axis=0))) output_labels = np.concatenate((output_labels, np.expand_dims(labels[:, index+interval_length+window_length: index+interval_length+window_length+forecasting_length], axis=0))) output_features = output_features[1:, :, :] output_labels = output_labels[1:, :, :] return torch.from_numpy(output_features), torch.from_numpy(output_labels)什么意思
这段代码实现了一个滚动窗口的多步时间序列预测的数据处理函数。函数接收四个参数:预测长度 forecasting_length,间隔长度 interval_length,滑动窗口长度 window_length,以及特征 features 和标签 labels。函数的输出是一个元组,其中包含了处理后的特征和标签,两者都被转换成了 PyTorch 的 Tensor 格式。
该函数的主要实现步骤是:遍历特征序列,从每个时间点开始,每隔 interval_length 个时间点,取出长度为 window_length 的滑动窗口作为输入特征,同时取出该窗口后 forecasting_length 个时间点的数据作为输出标签。这样,我们就可以将时间序列分成多个滑动窗口,每个窗口都对应一个输出标签。最终,函数返回的特征和标签分别是一个三维的 Tensor,第一维表示样本数量,第二维表示时间步数(即窗口长度),第三维表示特征或标签的维度。
阅读全文
相关推荐
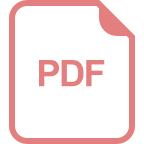
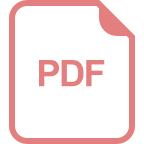
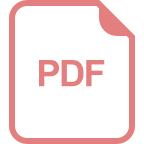













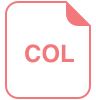