def zeros(shape, name=None):
时间: 2024-05-07 10:19:07 浏览: 3
这是一个 Python 函数的定义,用于创建一个全零的数组。参数 shape 是一个元组,指定了数组的形状。参数 name 是一个可选参数,用于指定数组的名称。
下面是示例代码:
```
import numpy as np
def zeros(shape, name=None):
return np.zeros(shape, dtype=np.float32, name=name)
```
这个函数使用了 NumPy 库中的 zeros 函数来创建全零数组,同时指定了数据类型为 32 位浮点数。如果指定了名称,则将其作为关键字参数传递给 zeros 函数。
相关问题
from scipy.sparse.linalg import eigsh, LinearOperator from scipy.sparse import isspmatrix, is_pydata_spmatrix class SVDRecommender: def init(self, k=50, ncv=None, tol=0, which='LM', v0=None, maxiter=None, return_singular_vectors=True, solver='arpack'): self.k = k self.ncv = ncv self.tol = tol self.which = which self.v0 = v0 self.maxiter = maxiter self.return_singular_vectors = return_singular_vectors self.solver = solver def svds(self, A): largest = self.which == 'LM' if not largest and self.which != 'SM': raise ValueError("which must be either 'LM' or 'SM'.") if not (isinstance(A, LinearOperator) or isspmatrix(A) or is_pydata_spmatrix(A)): A = np.asarray(A) n, m = A.shape if self.k <= 0 or self.k >= min(n, m): raise ValueError("k must be between 1 and min(A.shape), k=%d" % self.k) if isinstance(A, LinearOperator): if n > m: X_dot = A.matvec X_matmat = A.matmat XH_dot = A.rmatvec XH_mat = A.rmatmat else: X_dot = A.rmatvec X_matmat = A.rmatmat XH_dot = A.matvec XH_mat = A.matmat dtype = getattr(A, 'dtype', None) if dtype is None: dtype = A.dot(np.zeros([m, 1])).dtype else: if n > m: X_dot = X_matmat = A.dot XH_dot = XH_mat = _herm(A).dot else: XH_dot = XH_mat = A.dot X_dot = X_matmat = _herm(A).dot def matvec_XH_X(x): return XH_dot(X_dot(x)) def matmat_XH_X(x): return XH_mat(X_matmat(x)) XH_X = LinearOperator(matvec=matvec_XH_X, dtype=A.dtype, matmat=matmat_XH_X, shape=(min(A.shape), min(A.shape))) eigvals, eigvec = eigsh(XH_X, k=self.k, tol=self.tol ** 2, maxiter=self.maxiter, ncv=self.ncv, which=self.which, v0=self.v0) eigvals = np.maximum(eigvals.real, 0) t = eigvec.dtype.char.lower() factor = {'f': 1E3, 'd': 1E6} cond = factor[t] * np.finfo(t).eps cutoff = cond * np.max(eigvals) above_cutoff = (eigvals > cutoff) nlarge = above_cutoff.sum() nsmall = self.k - nlarge slarge = np.sqrt(eigvals[above_cutoff]) s = np.zeros_like(eigvals) s[:nlarge] = slarge if not self.return_singular_vectors: return np.sort(s) if n > m: vlarge = eigvec[:, above_cutoff] ularge = X_matmat(vlarge) / slarge if self.return_singular_vectors != 'vh' else None vhlarge = _herm(vlarge) else: ularge = eigvec[:, above_cutoff] vhlarge = _herm(X_matmat(ularge) / slarge) if self.return_singular_vectors != 'u' else None u = _augmented_orthonormal_cols(ularge, nsmall) if ularge is not None else None vh = _augmented_orthonormal_rows(vhlarge, nsmall) if vhlarge is not None else None indexes_sorted = np.argsort(s) s = s[indexes_sorted] if u is not None: u = u[:, indexes_sorted] if vh is not None: vh = vh[indexes_sorted] return u, s, vh def _augmented_orthonormal_cols(U, n): if U.shape[0] <= n: return U Q, R = np.linalg.qr(U) return Q[:, :n] def _augmented_orthonormal_rows(V, n): if V.shape[1] <= n: return V Q, R = np.linalg.qr(V.T) return Q[:, :n].T def _herm(x): return np.conjugate(x.T)这段代码中使用的scipy包太旧了,导致会出现报错信息为:cannot import name 'is_pydata_spmatrix' from 'scipy.sparse' (D:\Anaconda\lib\site-packages\scipy\sparse_init.py),将这段代码修改为使用最新版的scipy包
好的,将使用最新版的scipy包进行修改。首先需要将代码中的`is_pydata_spmatrix`替换为`isspmatrix_csr`。同时需要将代码开头的导入语句修改为`from scipy.sparse.linalg import eigsh, LinearOperator from scipy.sparse import isspmatrix_csr`。
class MyDense(keras.layers.Layer): def __init__(self, units, activation=None, **kwargs): super().__init__(**kwargs) self.units = units self.activation = keras.activations.get(activation) def build(self, batch_input_shape): self.kernel = self.add_weight( name="kernel", shape=[batch_input_shape[-1], self.units], initializer="glorot_normal") self.bias = self.add_weight( name="bias", shape=[self.units], initializer="zeros") super().build(batch_input_shape) # must be at the end def call(self, X): return self.activation(X @ self.kernel + self.bias) def compute_output_shape(self, batch_input_shape): return tf.TensorShape(batch_input_shape.as_list()[:-1] + [self.units]) def get_config(self): base_config = super().get_config() return {**base_config, "units": self.units, "activation": keras.activations.serialize(self.activation)}
这段代码定义了一个自定义的Keras层,名为MyDense。该层继承了Keras的Layer类,重写了__init__、build、call等方法。
在__init__方法中,定义了该层的参数units(输出维度)和激活函数activation,同时调用了父类的__init__方法。
在build方法中,定义了该层的权重kernel和偏置bias,权重的初始化方式为glorot_normal,偏置的初始化方式为zeros。同时调用了父类的build方法。
在call方法中,实现了该层的前向传播,即输入X与权重kernel相乘并加上偏置bias,再通过激活函数activation进行激活得到输出。
在compute_output_shape方法中,返回该层的输出shape。
在get_config方法中,返回该层的配置信息,包括units和activation等参数。
相关推荐
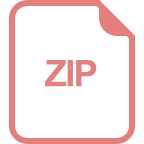
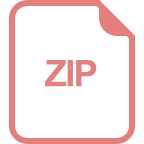
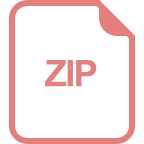












