这段代码def slide2(y): l = y.shape[0] y = tf.reshape(y, [1, -1, 1]) input = keras.Input(shape=(l, 1)) output = Conv1D(filters=1, kernel_size=3, padding='causal', activation='linear', trainable=False, use_bias=False)(input) output = tf.stop_gradient(output) # 将 output 视为常数 model = keras.Model(inputs=input, outputs=output) weights_list = model.get_weights() weights = np.ones(3) / 3 weights_list[0] = weights.reshape((3, 1, 1)) model.set_weights(weights_list) result = model.predict(y) result = tf.reshape(result, [-1, 1]) return result用在了循环内部,出现了WARNING:tensorflow:6 out of the last 6 calls to <function Model.make_predict_function.<locals>.predict_function at 0x000001B6B7A85EE0> triggered tf.function retracing.警告
时间: 2024-03-21 20:38:54 浏览: 23
这个警告通常是由于在循环中定义 `keras.Model.predict` 函数时触发的,原因是每次调用 `predict` 函数时都需要重新追踪计算图,这会导致性能下降。为了避免这个问题,你可以尝试将 `keras.Model.predict` 函数的定义放到循环外面。
具体而言,你可以先定义一个空的模型,然后在循环内部多次调用 `model` 的 `__call__` 方法,这样就可以避免重复追踪计算图。下面是修改后的代码示例:
```
def slide2(y):
l = y.shape[0]
y = tf.reshape(y, [1, -1, 1])
input = keras.Input(shape=(l, 1))
output = Conv1D(filters=1, kernel_size=3, padding='causal', activation='linear', trainable=False, use_bias=False)(input)
output = tf.stop_gradient(output) # 将 output 视为常数
model = keras.Model(inputs=input, outputs=output)
weights_list = model.get_weights()
weights = np.ones(3) / 3
weights_list[0] = weights.reshape((3, 1, 1))
model.set_weights(weights_list)
# 在循环外定义 result
result = np.zeros((y.shape[1], 1))
for i in range(y.shape[1]):
x = y[:, :i+1, :]
y_pred = model(x)
result[i, :] = y_pred[-1, 0]
return result
```
在这个例子中,我们在循环外定义了 `result`,并在循环内部多次调用了 `model` 的 `__call__` 方法,最终得到了和原始代码相同的结果。这样就避免了重复追踪计算图的问题,也就不会再出现 `tf.function retracing` 的警告了。
相关推荐
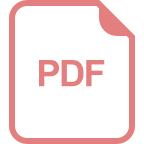
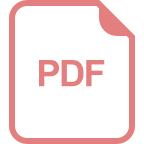
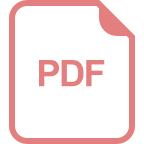














