共轭法的python 实现
时间: 2023-07-11 09:35:00 浏览: 105
共轭梯度法(Conjugate Gradient Method)是一种用于解决大规模线性方程组的迭代法,常用于优化问题中的求解。下面是一个简单的Python实现:
```python
import numpy as np
def conjugate_gradient(A, b, x0, tol=1e-10, max_iter=None):
"""
Conjugate Gradient Method for solving Ax = b
:param A: a square, positive definite matrix
:param b: a vector
:param x0: initial guess for solution
:param tol: tolerance for convergence
:param max_iter: maximum number of iterations
:return: solution x
"""
x = x0.copy()
r = b - np.dot(A, x)
p = r.copy()
if max_iter is None:
max_iter = len(b)
for i in range(max_iter):
Ap = np.dot(A, p)
alpha = np.dot(r, r) / np.dot(p, Ap)
x += alpha * p
r_new = r - alpha * Ap
if np.linalg.norm(r_new) < tol:
break
beta = np.dot(r_new, r_new) / np.dot(r, r)
p = r_new + beta * p
r = r_new
return x
```
其中,参数A是一个正定矩阵,b是一个向量,x0是解的初始猜测,tol是迭代收敛的容差,max_iter是最大迭代次数。函数返回解向量x。
该代码实现了标准的共轭梯度法,如果需要使用其他变种的共轭梯度法,可以在该基础上进行修改。
阅读全文
相关推荐
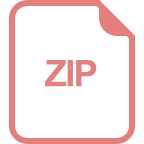


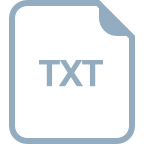








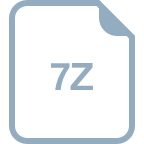