Matlab Coordinate Axis Scale Limitation Tips: Avoid Over- or Under-Scaling for More Rational Data Presentation
发布时间: 2024-09-13 22:35:18 阅读量: 9 订阅数: 11 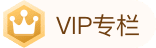
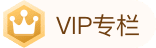
# 1. Overview of MATLAB Axis Scale Limitation
Axis scale limitation is a powerful feature in MATLAB that allows users to control the data range displayed on the axes. By limiting the axis scales, users can optimize data visualization, highlight key information, and enhance the professionalism of charts. This chapter will outline the uses, importance, and basic principles of axis scale limitation, laying the foundation for further exploration in subsequent chapters.
# 2. Theoretical Basis of Axis Scale Limitation
### 2.1 Role and Importance of Axis Scales
Axis scales are critical elements in data visualization, providing a reference framework that enables viewers to understand the range, distribution, and trends of data.
***Providing Data Range:** Axis scales define the scope of data visualization, helping viewers understand the minimum and maximum values of data.
***Displaying Data Distribution:** Scale lines and labels divide data into different intervals, allowing viewers to identify patterns of data distribution, such as normal or skewed distribution.
***Revealing Data Trends:** Scale lines and labels assist in identifying data trends, such as increases, decreases, or fluctuations.
***Enhancing Data Readability:** Clear axis scales enable viewers to quickly and accurately interpret data, improving data readability and understanding.
### 2.2 Principles and Algorithms of Axis Scale Limitation
Axis scale limitation refers to setting the minimum and maximum values of the axes to optimize data visualization. Its principles and algorithms are as follows:
***Determining the Data Range:** First, determine the minimum and maximum values of the data. This can be done using MATLAB's min and max functions or directly from the dataset.
***Setting Scale Ranges:** Based on the data range, set the minimum and maximum values for the axes. Typically, the minimum and maximum values should be slightly beyond the actual data range to provide some buffer space.
***Choosing Scale Units:** Choose appropriate scale units to make scale lines and labels clear and readable. The scale units should match the magnitude of the data.
***Setting Scale Intervals:** Determine the intervals between scale lines to ensure the number of scale lines and labels is moderate. The scale intervals should match the distribution pattern of the data.
**Code Example:**
```
% Determining the data range
data = [1, 3, 5, 7, 9];
min_value = min(data);
max_value = max(data);
% Setting scale range
axis([min_value-1, max_value+1, 0, 10]);
% Choosing scale unit
scale_unit = 1;
% Setting scale interval
scale_interval = 2;
% Setting scale lines and labels
set(gca, 'XTick', min_value:scale_interval:max_value);
set(gca, 'YTick', 0:scale_interval:10);
```
**Logical Analysis:**
This code example demonstrates the principles and algorithms of axis scale limitation.
* The `axis` function sets the minimum and maximum values for the axes, as well as the range of x-axis and y-axis scales.
* The `min` and `max` functions determine the m
0
0
相关推荐
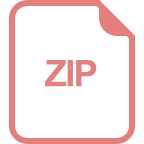
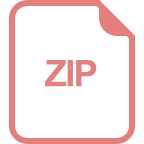
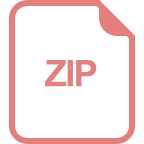





