Interior Point Method: A Modern Tool for Solving Linear Programming, Efficiently Tackling Large-Scale Problems
发布时间: 2024-09-13 13:50:41 阅读量: 33 订阅数: 19 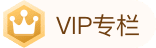
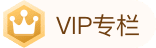
# 1. Theoretical Foundation of Interior Point Method
The interior point method is an optimization algorithm for solving linear programming problems, grounded in the theory of convex optimization. Convex optimization problems refer to optimization problems where both the objective function and constraints are convex functions. The interior point method leverages duality theory and the concept of barrier functions to transform a convex optimization problem into a series of solvable sub-problems, thus progressively approximating the optimal solution.
**Barrier functions** are functions that convert constraints into penalty terms, transforming the degree of constraint violation into a penalty value for the objective function by introducing a parameter. **Dual functions** are the objective functions of the dual problems of the original problem, closely related to the original problem's objective function.
**Central path** is a special path in the iterative process of the interior point method that connects the central point of the feasible domain and the optimal solution. The interior point method iteratively approaches the optimal solution by moving along the central path.
# 2. Implementation of the Interior Point Method Algorithm
### 2.1 Basic Principles of the Interior Point Method Algorithm
#### 2.1.1 Barrier Functions and Dual Functions
The core idea of the interior point method algorithm is to transform the original linear programming problem into a series of solvable sub-problems by constructing barrier functions and dual functions.
**Barrier Functions**
Barrier functions are penalty functions that penalize points on the boundary of the feasible domain. For a linear programming problem:
```
min f(x)
s.t. Ax ≤ b, x ≥ 0
```
The barrier function is defined as:
```
F(x, μ) = f(x) - μ ∑_{i=1}^m log(b_i - a_i^T x)
```
where μ > 0 is the barrier parameter.
**Dual Functions**
Dual functions are convex upper bounds for the original objective function. For a linear programming problem, its dual function is defined as:
```
g(y, s) = max_{x ≥ 0} [y^T x - s^T (Ax - b)]
```
where y ≥ 0 are dual variables, and s ≥ 0 are slack variables.
#### 2.1.2 Central Path and Iterative Process
The interior point method algorithm approximates the optimal solution of the problem by iteratively solving the barrier and dual functions.
**Central Path**
The central path is the set of intersection points between the barrier function and the dual function, forming a feasible path that connects the interior points of the original feasible domain and the optimal solution.
**Iterative Process**
The iterative process of the interior point method algorithm is as follows:
1. **Initialization:** Given an initial feasible solution x^0 and a dual solution (y^0, s^0), set the barrier parameter μ > 0.
2. **Iteration:**
- Solve for the central path point x^k of the barrier function F(x, μ).
- Solve for the central path point (y^k, s^k) of the dual function g(y, s).
- Update the barrier parameter μ.
3. **Convergence:** When the barrier parameter μ is sufficiently small, and x^k and (y^k, s^k) satisfy certain convergence conditions, stop the iteration.
### 2.2 Specific Steps of the Interior Point Method Algorithm
#### 2.2.1 Initialization Phase
1. Convert the linear programming problem into standard form:
```
min c^T x
s.t. Ax = b, x ≥ 0
```
2. Construct an initial feasible solution x^0 that satisfies Ax^0 = b, x^0 ≥ 0.
3. Construct an initial dual solution (y^0, s^0) that satisfies y^0 ≥ 0, s^0 ≥ 0, and y^0^T A - s^0^T = c^T.
4. Set the barrier parameter μ > 0.
#### 2.2.2 Iterative Phase
1. **Solve for the barrier function central path point x^k:**
```
min F(x, μ) = c^T x - μ ∑_{i=1}^m log(b_i - a_i^T x)
s.t. Ax = b, x ≥ 0
```
This problem can be solved using the interior point method algorithm or other optimization methods.
2. **Solve for the dual function central path point (y^k, s^k):**
```
max g(y, s) = y^T x^k - s^T (Ax^k - b)
s.t. y ≥ 0, s ≥ 0
```
This problem can be solved using the dual interior point method algorithm or other optimization methods.
3. **Update the barrier parameter μ:**
```
μ^{k+1} = θ μ^k
```
where θ ∈ (0, 1) is the damping factor.
#### 2.2.3 Convergence Conditions
The convergence conditions of the interior point method algorithm are as follows:
1. **Feasibility Conditions:**
```
||Ax^k - b|| ≤ ε
||x^k|| ≤ ε
```
2. **Duality Conditions:**
```
||c^T - y^k^T A + s^k^T|| ≤ ε
||y^k|| ≤ ε
||s^k|| ≤ ε
```
3. **Complementary Slackness Conditions:**
```
x^k_i s^k_i ≤ ε
```
Where ε > 0 is the predetermined convergence precision.
# 3.1 Steps of Solving Linear Programming Problems with Interior Point Method
#### 3.1.1 Model Transformation
The first step in solving a linear programming problem with the interior point method is to convert the problem into standard form. The standard form of a linear programming problem is as follows:
```
min cx
s.t. Ax = b
x >= 0
```
Where c is the coefficient vector of the objective function, x is the decision variable vector, A is the constraint matrix, and b is the constraint vector.
If the original linear programming problem is not in standard form, model transformation is necessary. There are two methods for model transformation:
1. **Introducing Slack Variables:** For inequality constraints, slack variables can be introduced to convert them into equality constraints.
2. **Introducing Artificial Variables:** For equality constraints, artificial variables can be introduced to convert them into inequality constraints.
#### 3.1.2 Algorithm Implementation
After converting the linear programming problem into standard form, the interior point method algorithm can be used for solving. The specific steps of the interior point method algorithm are as follows:
1. **Initialization:** Set the initial point x^0, the dual variable y^0, and the damping parameter μ^0.
2. **Iteration:**
- Solve the following system of equations:
```
(A^T y^k + μ^k I) Δx^k = -Ax^k + b
(A Δx^k)^T y^k + μ^k Δx^k = -c^T x^k
```
- Update the variables:
```
x^{k+1} = x^k + Δx^k
y^{k+1} = y^k + Δy^k
μ^{k+1} = θ μ^k
```
Where θ is the damping parameter adjustment factor, typically taken as 0.5.
3. **Convergence Judgment:**
- Check whether the following conditions are met:
```
||Ax^k - b|| < ε
||A^T y^k + μ^k I|| < ε
||c^T x^k + (A Δx^k)^T y^k + μ^k Δx^k|| < ε
```
Where ε is the convergence precision. If the above conditions are satisfied, the algorithm has converged.
#### 3.1.3 Result Analysis
After the interior point method algorithm converges, the optimal solution x^* and the dual optimal solution y^* can be obtained. The optimal solution x^* is a feasible solution to the linear programming problem and satisfies the minimum value of the objective function. The dual optimal solution y^* is a feasible solution to the dual problem and satisfies the maximum value of the dual function.
Through the analysis of the optimal solution and the dual optimal solution, the following information can be obtained:
- **Sensitivity of the Optimal Solution:** By analyzing the dual variable y^*, the impact of the objective function coefficients and constraints on the optimal solution can be determined.
- **Feasible Domain of the Dual Problem:** By analyzing the dual optimal solution y^*, the feasible domain of the dual problem can be determined, thus judging whether the original linear programming problem has a feasible solution.
- **Optimality of the Linear Programming Problem:** By comparing the objective function value and the dual function value, the optimality of the linear programming problem can be judged.
# ***parison of Interior Point Method with Other Solution Methods
### 4.1 Comparison of Interior Point Method and Simplex Method
#### 4.1.1 Differences in Algorithm Principles
Both the interior point method and the simplex method are algorithms for solving linear programming problems, but their algorithm principles are entirely different.
* The **simplex method** uses a **simplex tableau** for iteration, selecting a basic variable to leave the basis and another to enter, until a feasible solution is found.
* The **interior point method** uses **barrier functions** and **dual functions**, iteratively updating points on the central path to gradually approach the optimal solution.
#### 4.1.2 Comparison of Efficiency and Stability
In terms of efficiency, the interior point method is generally more efficient than the simplex method, especially in solving large-scale linear programming problems. This is because the interior point method produces a feasible solution in each iteration, whereas the simplex method may require multiple iterations to find a feasible solution.
In terms of stability, the interior point method is also more stable than the simplex method. The simplex method can sometimes fall into cycles, whereas the interior point method can avoid this.
### 4.2 Comparison of Interior Point Method with Other Modern Solution Methods
In addition to the simplex method, there are other modern solution methods for solving linear programming problems, such as:
***Coordinate Descent Method**
***Gradient Projection Method**
#### 4.2.1 Coordinate Descent Method
The coordinate descent method is an iterative algorithm that selects one variable at a time, fixes the others, and then updates the value of that variable to minimize the objective function. The algorithm is simple and easy to understand, but its convergence speed is slow.
#### 4.2.2 Gradient Projection Method
The gradient projection method is also an iterative algorithm that calculates the gradient of the objective function in each iteration and then projects the gradient onto the feasible domain, updating the current point. The algorithm's convergence speed is faster than the coordinate descent method, but it requires the calculation of gradients, which is computationally more intensive.
**The table below compares the advantages and disadvantages of the interior point method with other modern solution methods:**
| Solution Method | Advantages | Disadvantages |
|---|---|---|
| Interior Point Method | High efficiency, good stability | High computational effort |
| Coordinate Descent Method | Simple and easy to understand | Slow convergence speed |
| Gradient Projection Method | Fast convergence speed | High computational effort |
In practical applications, the choice of solution method should be determined based on the specific problem's scale, structure, and precision requirements.
# 5. Optimization of Interior Point Method Algorithm
The interior point method algorithm demonstrates good solution efficiency and stability in practice, but there are still areas that can be optimized, mainly focusing on convergence speed and storage space. This chapter will explore methods for optimizing the interior point method algorithm to further enhance its performance.
### 5.1 Optimization of Convergence Speed of Interior Point Method Algorithm
#### 5.1.1 Preprocessing Techniques
Preprocessing techniques can transform the original problem to some extent, ***mon preprocessing techniques include:
- **Variable Scaling:** Scale the variables to similar orders of magnitude to avoid numerical imbalance issues that could cause convergence difficulties.
- **Matrix Ordering:** Sort the constraint matrix to concentrate the non-zero elements near the diagonal, reducing the computational effort required for sparse matrix solutions.
- **Inequality Conversion:** Convert inequality constraints into equality constraints to simplify the problem structure and improve solution efficiency.
#### 5.1.2 Iterative Parameter Adjustm***
***mon iterative parameters include:
- **Step Size Parameter:** Controls the size of each iteration step; too large a step size may lead to algorithm instability, while too small a step size may slow down convergence.
- **Damping Parameter:** Used to control the curvature of the dual function; too large a damping parameter may lead to slow convergence, while too small a damping parameter may cause the algorithm to diverge.
By dynamically adjusting the iterative parameters, the convergence speed of the algorithm can be optimized. For example, in the early stages of the algorithm, larger step sizes and damping parameters can be used to accelerate convergence; in the later stages, smaller step sizes and damping parameters can be used to improve convergence precision.
### 5.2 Optimization of Storage Space of Interior Point Method Algorithm
#### 5.2.1 Sparse Matrix Storage Techniques
The interior point method algorithm involves a large number of sparse matrix operations, ***mon sparse matrix storage techniques include:
- **Compressed Row Storage (CRS):** Stores each row's non-zero elements and column indices in a continuous array.
- **Compressed Column Storage (CCS):** Stores each column's non-zero elements and row indices in a continuous array.
- **Hash Table Storage:** Stores the non-zero elements and their row and column indices of the sparse matrix in a hash table.
#### 5.2.2 Matrix Decomposition Techniques
By decomposing sparse matrices, storage space can be reduced, ***mon matrix decomposition techniques include:
- **LU Decomposition:** Decomposes the sparse matrix into a product of a lower triangular matrix and an upper triangular matrix, facilitating the solution of linear equations.
- **QR Decomposition:** Decomposes the sparse matrix into a product of an orthogonal matrix and an upper triangular matrix, used for solving least squares problems.
- **Singular Value Decomposition (SVD):** Decomposes the sparse matrix into a product of three matrices, used for dimensionality reduction and data analysis.
By employing appropriate matrix decomposition techniques, the sparse matrix can be stored in a more compact form, thus optimizing the algorithm's storage space.
# 6. Future Development Trends of Interior Point Method
### 6.1 Theoretical Improvements of Interior Point Method Algorithm
#### 6.1.1 Adaptive Algorithms
Traditional interior point method algorithms use a fixed step size strategy, meaning the same step size parameter is used in each iteration. However, in practical applications, the scale and structure of problems can vary greatly, necessitating adaptive algorithms that automatically adjust step size parameters based on problem characteristics. Adaptive algorithms can improve the convergence speed and stability of the algorithm.
#### 6.1.2 Robust Algorithms
The interior point method algorithm may perform poorly when dealing with uncertainties and noisy data. Robust algorithms aim to increase the tolerance of the algorithm to disturbances, allowing it to remain effective in the presence of uncertainties or noise. Robust algorithms can employ various techniques, such as parameter perturbation and random projection.
### 6.2 Practical Application of Interior Point Method Algorithm
#### 6.2.1 Distributed Computing
With the advent of the big data era, the scale of problems that need to be solved is growing. Distributed computing can break down large-scale problems into multiple sub-problems and solve them in parallel on different computing nodes. The interior point method algorithm can be easily parallelized, making it suitable for distributed computing environments.
#### 6.2.2 Cloud Computing
Cloud computing provides a model for on-demand access to computing resources. The interior point method algorithm can be deployed on cloud platforms, leveraging the elasticity, scalability, and cost-effectiveness of cloud computing. With cloud computing, users can dynamically allocate and release computing resources according to their needs, thus reducing computing costs.
0
0
相关推荐
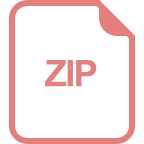
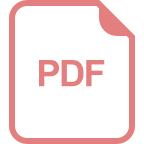
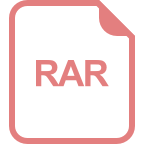
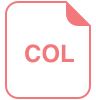
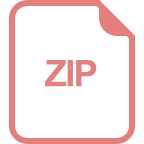
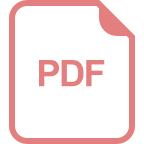
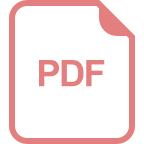
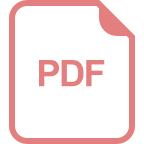
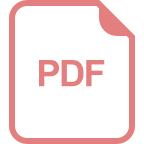