TensorFlow 生态系统全面解读
发布时间: 2024-05-03 01:19:33 阅读量: 88 订阅数: 40 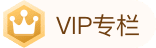
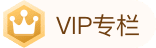
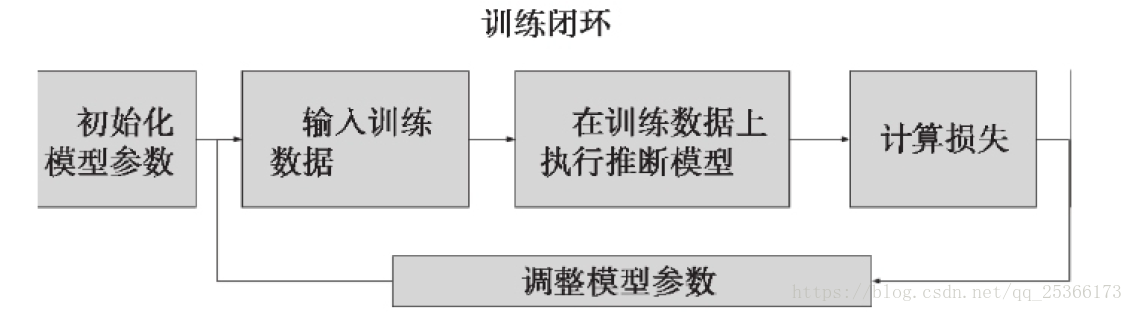
# 1. TensorFlow概述**
TensorFlow是一个开源的机器学习框架,由谷歌开发,用于构建和训练机器学习模型。它提供了一系列工具和API,使开发人员能够轻松地创建和部署复杂的神经网络模型。TensorFlow以其灵活性和可扩展性而闻名,使其成为各种机器学习任务的理想选择。
# 2.1 TensorFlow图计算模型
### 2.1.1 图结构与操作节点
TensorFlow采用图计算模型,将计算过程表示为一个有向无环图(DAG)。图中的节点代表操作,而边代表数据流。
操作节点可以是算术运算(如加法、乘法)、逻辑运算(如比较、布尔运算)或其他复杂操作(如卷积、池化)。每个操作节点都有一个或多个输入和一个或多个输出。
### 2.1.2 数据流与计算过程
数据通过图中的边在操作节点之间流动。每个操作节点执行特定的计算,并将其输出发送到后续节点。
TensorFlow图的执行过程如下:
1. **图构建:**定义图结构,包括操作节点和数据流。
2. **会话创建:**创建会话对象,用于执行图。
3. **数据馈送:**将输入数据馈送到图中。
4. **图执行:**会话执行图,计算操作节点并生成输出。
#### 代码示例
```python
import tensorflow as tf
# 定义两个常量节点
a = tf.constant(5)
b = tf.constant(3)
# 定义一个加法操作节点
c = tf.add(a, b)
# 创建会话
sess = tf.Session()
# 执行图并获取结果
result = sess.run(c)
print(result) # 输出:8
```
**逻辑分析:**
* `tf.constant` 创建常量节点,将给定值作为输入。
* `tf.add` 创建加法操作节点,将两个输入节点相加。
* `sess.run` 执行图并返回操作节点的输出。
#### 参数说明
| 参数 | 描述 |
|---|---|
| `a` | 第一个输入节点 |
| `b` | 第二个输入节点 |
| `c` | 加法操作节点 |
| `sess` | 会话对象 |
# 3. TensorFlow开发实战
### 3.1 TensorFlow模型训练
#### 3.1.1 数据预处理与加载
TensorFlow模型训练的第一步是准备和加载训练数据。数据预处理是至关重要的,它可以提高模型的性能并加快训练过程。
**数据预处理步骤:**
1. **数据清洗:**删除缺失值、异常值和重复项。
2. **数据归一化:**将数据缩放或标准化到特定范围内,以提高模型的稳定性和收敛速度。
3. **数据增强:**通过随机旋转、裁剪或翻转等技术创建新数据样本,以增加数据集的多样性。
**数据加载:**
TensorFlow提供了多种数据加载器,用于从各种来源加载数据,例如CSV文件、图像和文本。
```python
import tensorflow as tf
# 从CSV文件加载数据
dataset = tf.data.experimental.make_csv_dataset("data.csv", batch_size=32)
# 从图像目录加载图像数据
dataset = tf.keras.preprocessing.image_dataset_from_directory("images", batch_size=32)
```
#### 3.1.2 模型构建与优化
模型构建涉及定义模型的架构和超参数。TensorFlow提供了各种预构建的模型,也可以从头开始构建自定义模型。
**模型架构:**
模型架构决定了模型如何处理数据。常见的架构包括:
* **全连接神经网络:**层与层之间完全连接。
* **卷积神经网络:**用于处理图像和序列数据。
* **循环神经网络:**用于处理序列数据,例如文本和时间序列。
**超参数:**
超参数控制模型的训练过程,包括:
* **学习率:**确定模型更新权重的速度。
* **批次大小:**每次训练迭代中使用的样本数量。
* **时期数:**模型在整个数据集上训练的次数。
**优化器:**
优化器用于更新模型的权重,以最小化损失函数。常见的优化器包括:
* **梯度下降:**沿着损失函数的负梯度方向更新权重。
* **动量:**通过考虑先前梯度来加速更新。
* **RMSProp:**通过使用指数加权移动平均来自适应地调整学习率。
**损失函数:**
损失函数衡量模型预测与真实标签之间的差异。常见的损失函数包括:
* **均方误差:**用于回归任务。
* **交叉熵:**用于分类任务。
* **二元交叉熵:**用于二分类任务。
**代码示例:**
```python
# 构建一个简单的全连接神经网络
model = tf.keras.Sequential([
tf.keras.layers.Dense(128, activation='relu'),
tf.keras.layers.Dense(10, activation='softmax')
])
# 编译模型
model.compile(optimizer='adam', loss='sparse_categorical_crossentropy', metrics=['accuracy'])
# 训练模型
model.fit(dataset, epochs=10)
```
# 4. TensorFlow扩展与应用
### 4.1 TensorFlow与其他框架集成
#### 4.1.1 TensorFlow与Keras集成
Keras是一个高级神经网络API,它建立在TensorFlow之上,提供了更易于使用和简洁的接口。通过集成Keras,TensorFlow用户可以轻松构建和训练复杂的神经网络模型。
```python
# 导入必要的库
import tensorflow as tf
from keras import models, layers
# 创建一个Keras模型
model = models.Sequential()
model.add(layers.Dense(units=10, activation='relu', input_shape=(784,)))
model.add(layers.Dense(units=10, activation='softmax'))
# 编译模型
model.compile(optimizer='adam', loss='sparse_categorical_crossentropy', metrics=['accuracy'])
# 训练模型
model.fit(x_train, y_train, epochs=10)
```
**参数说明:**
* `units`: 神经元数量
* `activation`: 激活函数
* `input_shape`: 输入数据的形状
* `optimizer`: 优化器
* `loss`: 损失函数
* `metrics`: 评估指标
#### 4.1.2 TensorFlow与PyTorch集成
PyTorch是一个动态计算框架,它允许用户在训练过程中动态修改计算图。通过集成PyTorch,TensorFlow用户可以利用PyTorch的灵活性来构建更复杂的模型。
```python
# 导入必要的库
import tensorflow as tf
import torch
# 创建一个PyTorch模型
class Net(torch.nn.Module):
def __init__(self):
super(Net, self).__init__()
self.fc1 = torch.nn.Linear(784, 10)
self.fc2 = torch.nn.Linear(10, 10)
def forward(self, x):
x = self.fc1(x)
x = torch.relu(x)
x = self.fc2(x)
return x
# 创建一个TensorFlow模型
tf_model = tf.keras.models.Sequential([
tf.keras.layers.Dense(units=10, activation='relu', input_shape=(784,)),
tf.keras.layers.Dense(units=10, activation='softmax')
])
# 转换TensorFlow模型为PyTorch模型
pytorch_model = tf.keras.utils.convert_keras_to_pytorch(tf_model)
```
### 4.2 TensorFlow在不同领域的应用
#### 4.2.1 TensorFlow在计算机视觉中的应用
TensorFlow在计算机视觉领域有着广泛的应用,包括图像分类、目标检测和图像分割。
**图像分类**
```python
# 导入必要的库
import tensorflow as tf
from tensorflow.keras.datasets import mnist
# 加载MNIST数据集
(x_train, y_train), (x_test, y_test) = mnist.load_data()
# 创建一个TensorFlow模型
model = tf.keras.models.Sequential([
tf.keras.layers.Flatten(input_shape=(28, 28)),
tf.keras.layers.Dense(units=128, activation='relu'),
tf.keras.layers.Dense(units=10, activation='softmax')
])
# 编译模型
model.compile(optimizer='adam', loss='sparse_categorical_crossentropy', metrics=['accuracy'])
# 训练模型
model.fit(x_train, y_train, epochs=10)
```
**目标检测**
```python
# 导入必要的库
import tensorflow as tf
from tensorflow.keras.applications.resnet50 import ResNet50
# 创建一个ResNet50模型
base_model = ResNet50(weights='imagenet', include_top=False)
# 添加自定义层进行目标检测
model = tf.keras.Sequential([
base_model,
tf.keras.layers.GlobalAveragePooling2D(),
tf.keras.layers.Dense(units=1024, activation='relu'),
tf.keras.layers.Dense(units=4, activation='softmax')
])
# 编译模型
model.compile(optimizer='adam', loss='sparse_categorical_crossentropy', metrics=['accuracy'])
# 训练模型
model.fit(x_train, y_train, epochs=10)
```
**图像分割**
```python
# 导入必要的库
import tensorflow as tf
from tensorflow.keras.applications.vgg16 import VGG16
# 创建一个VGG16模型
base_model = VGG16(weights='imagenet', include_top=False)
# 添加自定义层进行图像分割
model = tf.keras.Sequential([
base_model,
tf.keras.layers.UpSampling2D(size=(2, 2)),
tf.keras.layers.Conv2D(filters=32, kernel_size=(3, 3), activation='relu'),
tf.keras.layers.Conv2D(filters=1, kernel_size=(1, 1), activation='sigmoid')
])
# 编译模型
model.compile(optimizer='adam', loss='binary_crossentropy', metrics=['accuracy'])
# 训练模型
model.fit(x_train, y_train, epochs=10)
```
#### 4.2.2 TensorFlow在自然语言处理中的应用
TensorFlow在自然语言处理领域也有着广泛的应用,包括文本分类、机器翻译和文本摘要。
**文本分类**
```python
# 导入必要的库
import tensorflow as tf
from tensorflow.keras.datasets import imdb
# 加载IMDB数据集
(x_train, y_train), (x_test, y_test) = imdb.load_data(num_words=10000)
# 创建一个TensorFlow模型
model = tf.keras.models.Sequential([
tf.keras.layers.Embedding(input_dim=10000, output_dim=128),
tf.keras.layers.LSTM(units=128),
tf.keras.layers.Dense(units=1, activation='sigmoid')
])
# 编译模型
model.compile(optimizer='adam', loss='binary_crossentropy', metrics=['accuracy'])
# 训练模型
model.fit(x_train, y_train, epochs=10)
```
**机器翻译**
```python
# 导入必要的库
import tensorflow as tf
from tensorflow.keras.preprocessing.text import Tokenizer
from tensorflow.keras.preprocessing.sequence import pad_sequences
# 加载数据
data = pd.read_csv('data.csv')
sentences = data['sentence'].values
labels = data['label'].values
# 分词和编码
tokenizer = Tokenizer(num_words=10000)
tokenizer.fit_on_texts(sentences)
sequences = tokenizer.texts_to_sequences(sentences)
padded = pad_sequences(sequences, maxlen=100)
# 创建一个TensorFlow模型
model = tf.keras.models.Sequential([
tf.keras.layers.Embedding(input_dim=10000, output_dim=128),
tf.keras.layers.LSTM(units=128),
tf.keras.layers.Dense(units=1, activation='sigmoid')
])
# 编译模型
model.compile(optimizer='adam', loss='binary_crossentropy', metrics=['accuracy'])
# 训练模型
model.fit(padded, labels, epochs=10)
```
**文本摘要**
```python
# 导入必要的库
import tensorflow as tf
from tensorflow.keras.preprocessing.text import Tokenizer
from tensorflow.keras.preprocessing.sequence import pad_sequences
# 加载数据
data = pd.read_csv('data.csv')
texts = data['text'].values
summaries = data['summary'].values
# 分词和编码
tokenizer = Tokenizer(num_words=10000)
tokenizer.fit_on_texts(texts)
sequences = tokenizer.texts_to_sequences(texts)
padded = pad_sequences(sequences, maxlen=100)
# 创建一个TensorFlow模型
model = tf.keras.models.Sequential([
tf.keras.layers.Embedding(input_dim=10000, output_dim=128),
tf.keras.layers.LSTM(units=128),
tf.keras.layers.Dense(units=1, activation='sigmoid')
])
# 编译模型
model.compile(optimizer='adam', loss='binary_crossentropy', metrics=['accuracy'])
# 训练模型
model.fit(padded, summaries, epochs=10)
```
# 5.1 TensorFlow社区与资源
### 5.1.1 TensorFlow官方文档与教程
TensorFlow官方文档是学习和使用TensorFlow的重要资源。它提供了全面的教程、指南和API参考,涵盖了TensorFlow的各个方面,从基础概念到高级技术。
TensorFlow教程涵盖了从入门到高级的各种主题,包括:
- TensorFlow基础
- 数据预处理和加载
- 模型构建和训练
- 模型评估和部署
- TensorFlow与其他框架集成
TensorFlow API参考提供了对TensorFlow所有函数、类和模块的详细说明。它对于理解TensorFlow的内部工作原理和使用其API进行开发至关重要。
### 5.1.2 TensorFlow社区论坛与交流
TensorFlow社区是一个充满活力的生态系统,拥有大量的论坛、讨论组和社交媒体群组。这些平台为用户提供了与其他TensorFlow开发者联系、讨论问题、分享知识和获得帮助的机会。
主要的TensorFlow社区论坛包括:
- TensorFlow社区论坛:https://discuss.tensorflow.org/
- Stack Overflow上的TensorFlow标签:https://stackoverflow.com/questions/tagged/tensorflow
- GitHub上的TensorFlow问题跟踪器:https://github.com/tensorflow/tensorflow/issues
TensorFlow社区还活跃在社交媒体上,包括Twitter、LinkedIn和YouTube。这些平台提供了与TensorFlow团队和社区成员互动的机会,了解最新消息和更新,并参与讨论。
0
0
相关推荐








