Efficient Data Extraction and Manipulation in MATLAB: Handling MAT Files for Rapid Analysis
发布时间: 2024-09-14 07:27:32 阅读量: 32 订阅数: 33 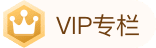
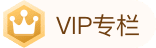
# Introduction to MATLAB MAT Files
MATLAB MAT files are a binary file format used for storing MATLAB variables and data. They allow users to save workspace data for reloading and reuse in subsequent sessions. MAT files are particularly useful for storing large datasets and sharing data.
A MAT file consists of a header part and a data part. The header contains information about the file format, version, and stored variables. The data part contains the actual variable data, stored in binary format. MAT files can be read and written using MATLAB's `load()` and `save()` functions.
# Fundamentals of Array Processing
### 2.1 Basic Concepts and Operations of Arrays
**Concept of Arrays:**
An array in MATLAB is an ordered collection of elements, each with a specific data type and position. Elements within an array can be scalars (single values), vectors (one-dimensional arrays), matrices (two-dimensional arrays), or arrays with higher dimensions.
**Creating Arrays:**
Arrays in MATLAB can be created using various methods, including:
- Direct assignment: `A = [1, 2, 3; 4, 5, 6]`
- Using built-in functions: `zeros(m, n)` creates an m x n zero matrix, `ones(m, n)` creates an m x n matrix of ones, `rand(m, n)` creates an m x n matrix of random numbers
- Importing from external files or data sources
**Accessing Arrays:**
Array elements can be accessed using indexing. An index is an integer that represents the position of the element in the array. MATLAB indexing starts at 1. For example: `A(2, 3)` accesses the element in the second row and third column of matrix A.
**Operating on Arrays:**
MATLAB provides a rich set of array operators, including:
- Arithmetic operations: addition (+), subtraction (-), multiplication (*), division (/)
- Logical operations: greater than (>), less than (<), equal to (==)
- Array concatenation: joining two arrays to form a new array
- Array merging: combining two arrays into a multidimensional array
### 2.2 Indexing and Slicing of Arrays
**Indexing:**
As mentioned, indexing is used to access specific elements within an array. MATLAB supports several types of indexing:
- Linear indexing: an integer representing the linear position of the element in the array
- Colon indexing: a colon (:), representing a range of consecutive elements from start to end
- Logical indexing: a Boolean array representing conditions for accessing elements
**Slicing:**
Slicing is a convenient way to obtain a subset of an array. The slicing syntax is as follows: `array(start:end:step)`, where:
- start: starting index
- end: ending index (not inclusive)
- step: optional step size
For example: `A(2:4, 1:3:2)` retrieves a submatrix from the 2nd to the 4th row and 1st to the 3rd column, with a step of 2 from matrix A.
### 2.3 Array Concatenation and Merging
**Array Concatenation:**
Arrays in MATLAB can be concatenated using the `[ ]` operator. The result is a new array with the elements of the two arrays arranged in sequence. For example: `[A, B]` horizontally concatenates matrix A and B to form a new matrix.
**Array Merging:**
Arrays in MATLAB can be merged using the `cat` function. The syntax for `cat` is: `cat(dimension, array1, array2, ...)`, where:
- dimension: specifies the dimension of the merge (1 for row-wise, 2 for column-wise)
- array1, array2, ...: arrays to be merged
For example: `cat(1, A, B)` vertically merges matrix A and B to form a new matrix.
# 3.1 Structure and Format of MAT Files
A MAT file is a binary format used for storing MATLAB variables. It consists of the following parts:
- **File Header:** Contains information such as the file version, data types, and the number of variables.
- **Variable Header:** For each variable, it includes variable names, data types, dimensions, and the number of elements.
- **Data Block:** Contains the actual data of the variables.
The structure of a MAT file is illustrated by the following diagram:
```mermaid
graph LR
subgraph MAT File
A[File Header] --> B[Variable Header]
B[Variable Header] --> C[Data Block]
end
```
### 3.2 Reading and Writing MAT Files with load() and save() Functions
MATLAB provides the `load()` and `save()` functions for reading and writing MAT files.
#### 3.2.1 Using the load() Function to Read MAT Files
The `load()` function is used to load variables from a MAT file. Its syntax is:
```
load(filename)
```
Here, `filename` is the name of the MAT file.
For example, the following code loads variables `x` and `y` from a MAT file named `data.mat`:
```
load('data.mat')
```
#### 3.2.2 Using the save() Function to Write to MAT Files
The `save()` function is used to save variables to a MAT file. Its syntax is:
```
save(filename, variables)
```
Here, `filename` is the name of the MAT file, and `variables` is a list of variables to be saved.
For example, the following code saves variables `x` and `y` to a MAT file named `data.mat`:
```
save('data.mat', 'x', 'y')
```
### 3.3 Data Type Conversion in MAT Files
MATLAB MAT files support a variety of data types, including:
- Numeric types (e.g., `int8`, `double`)
- String types (e.g., `char`)
- Logical types (e.g., `logical`)
- Structure types (e.g., `struct`)
- Cell array types (e.g., `cell`)
When reading and writing MAT files, MATLAB automatically converts data types to MATLAB-compatible types. For example, when loading strings from a MAT file, MATLAB converts them to `char` arrays.
If custom data types need to be stored in MAT files, `struct` or `cell` arrays can be used.
# 4. Advanced Techniques for Array Processing
### 4.1 Reshaping and Transposing Arrays
#### 4.1.1 Reshaping Arrays
The `reshape()` function in MATLAB can be used to change the shape of an array without altering its elements. The syntax is:
```matlab
B = reshape(A, m, n)
```
Where:
- `A` is the array to be reshaped.
- `m` and `n` are the desired number of rows and columns for the new array.
- `B` is the reshaped array.
For example, reshaping a 1x12 row vector into a 3x4 matrix:
```matlab
A = 1:12;
B = reshape(A, 3, 4);
```
The resulting `B` will be a 3x4 matrix:
```
B =
***
***
***
```
#### 4.1.2 Transposing Arrays
The `transpose()` function can be used to transpose an array, swapping its rows and columns. The syntax is:
```matlab
B = transpose(A)
```
Where:
- `A` is the array to be transposed.
- `B` is the transposed array.
For example, transposing a 3x4 matrix:
```matlab
A = [1 2 3 4; 5 6 7 8; 9 10 11 12];
B = transpose(A);
```
The resulting `B` will be a 4x3 matrix:
```
B =
***
***
***
***
```
### 4.2 Sorting and Filtering Arrays
#### 4.2.1 Sorting Arrays
The `sort()` function in MATLAB can be used to sort an array. The syntax is:
```matlab
B = sort(A)
```
Where:
- `A` is the array to be sorted.
- `B` is the sorted array.
By default, the `sort()` function sorts in ascending order. To sort in descending order, use `sort(A, 'descend')`.
For example, sorting a numeric array in ascending order:
```matlab
A = [3 1 2 5 4];
B = sort(A);
```
The resulting `B` will be a sorted array in ascending order:
```
B =
1 2 3 4 5
```
#### 4.2.2 Filtering Arrays
The `logical()` function in MATLAB can be used to create logical arrays where elements are either `true` or `false`. Then, logical indexing can be used to filter arrays. The syntax is:
```matlab
B = A(logical(condition))
```
Where:
- `A` is the array to be filtered.
- `condition` is a logical expression that returns an array of `true` or `false`.
- `B` is the filtered array, containing only elements that satisfy `condition`.
For example, filtering a numeric array to retain only elements greater than 3:
```matlab
A = [1 2 3 4 5 6];
B = A(logical(A > 3));
```
The resulting `B` will be an array containing only elements greater than 3:
```
B =
4 5 6
```
### 4.3 Statistical Analysis and Visualization of Arrays
#### 4.3.1 Statistical Analysis of Arrays
MATLAB provides various functions for statistical analysis of arrays, including:
* `mean()`: calculates the mean of the array.
* `median()`: calculates the median of the array.
* `std()`: calculates the standard deviation of the array.
* `max()`: calculates the maximum value in the array.
* `min()`: calculates the minimum value in the array.
For example, calculating the mean and standard deviation of a numeric array:
```matlab
A = [1 2 3 4 5 6];
mean_value = mean(A);
std_dev = std(A);
```
The `mean_value` will be 3.5, and `std_dev` will be 1.8708.
#### 4.3.2 Visualization of Arrays
The `plot()` function in MATLAB can be used to visualize arrays. The syntax is:
```matlab
plot(x, y)
```
Where:
- `x` is the horizontal axis data.
- `y` is the vertical axis data.
For example, plotting a line graph of a numeric array:
```matlab
A = [1 2 3 4 5 6];
plot(1:6, A);
```
A line graph will be generated, where the horizontal axis ranges from 1 to 6, and the vertical axis represents the values of array A.
# 5.1 Extracting Specific Data from MAT Files
In practical applications, we often need to extract specific data from MAT files for analysis or processing. MATLAB provides several methods to achieve this.
**Using the load() Function**
The `load()` function is the most commonly used method for reading MAT files. It can load an entire MAT file at once or only specific variables.
```matlab
% Load entire MAT file
data = load('data.mat');
% Load specific variables
x = load('data.mat', 'x');
```
**Using the whos() Function**
The `whos()` function displays information about all variables in a MAT file, including variable names, sizes, and types.
```matlab
whos('data.mat')
```
**Using the whos('-file') Function**
The `whos('-file')` function displays information about all variables in a MAT file, organized by file path.
```matlab
whos('-file', 'data.mat')
```
**Using the isfield() Function**
The `isfield()` function checks if a specific variable exists within a MAT file.
```matlab
if isfield('data', 'x')
% Variable x exists
else
% Variable x does not exist
end
```
**Using the getfield() Function**
The `getfield()` function extracts the value of a specific variable from a MAT file.
```matlab
x = getfield(data, 'x');
```
**Using the struct() Function**
The `struct()` function can convert variables from a MAT file into a structure.
```matlab
data_struct = struct(data);
```
By utilizing these methods, we can flexibly extract specific data from MAT files to meet various application needs.
0
0
相关推荐
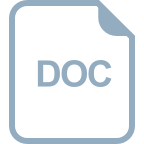
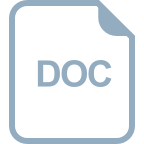
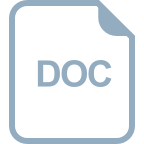
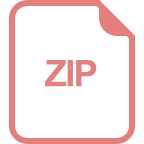
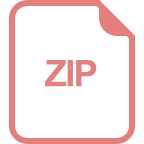
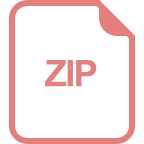
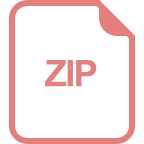
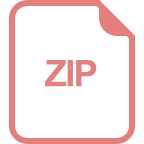