请调用刚写的findpeak函数完成这个代码# Mean shift algorithm def meanshift(data, r): labels = np.zeros(len(data.T)) peaks = [] #聚集的类中心 label_no = 1 #当前label labels[0] = label_no # findpeak is called for the first index out of the loop peak = findpeak(data, 0, r) peakT = np.concatenate(peak, axis=0).T peaks.append(peakT) # Every data point is iterated through for idx in range(0, len(data.T)): # 遍历数据,寻找当前点的peak # 并实时关注当前peak是否会收敛到一个新的聚类(和已有peaks比较) # 若是,更新label_no,peaks,labels,继续 # 若不是,当前点就属于已有类,继续 ### YOUR CODE HERE pass ### END YOUR CODE #print(set(labels)) return labels, np.array(peaks).T
时间: 2024-02-14 14:10:14 浏览: 139
好的,现在我们需要在meanshift函数中调用findpeak函数,找到每个数据点所属的聚类的中心点(peak)。同时,我们需要检查当前点的peak是否已经存在于已有的聚类中,如果是,则将该点赋予已有的类别,否则创建一个新的类别。以下是代码实现:
```
def meanshift(data, r):
labels = np.zeros(len(data.T))
peaks = [] #聚集的类中心
label_no = 1 #当前label
labels[0] = label_no
peak = findpeak(data, 0, r)
peakT = np.concatenate(peak, axis=0).T
peaks.append(peakT)
for idx in range(1, len(data.T)):
# 寻找当前点的peak
curr_peak = findpeak(data, idx, r)
# 实时关注当前peak是否会收敛到一个新的聚类(和已有peaks比较)
found_cluster = False
for i, p in enumerate(peaks):
# 计算当前点与已有的peak之间的距离
dist = np.sqrt(np.sum((curr_peak - p)**2))
if dist <= r:
# 如果距离小于等于r,则将该点归入已有的类别中
labels[idx] = i+1
found_cluster = True
break
if not found_cluster:
# 如果距离大于r,则创建一个新的类别
label_no += 1
labels[idx] = label_no
peaks.append(np.concatenate(curr_peak, axis=0).T)
return labels, np.array(peaks).T
```
在循环中,我们首先调用findpeak函数找到当前点的peak,然后遍历所有已经存在的peak,计算当前点与它们之间的距离,如果距离小于等于r,则将该点归为已有的类别中,否则创建一个新的类别。需要注意的是,我们在labels中使用1,2,3...等正整数来表示不同的类别,而非像k-means那样使用0,1,2...等整数。
阅读全文
相关推荐









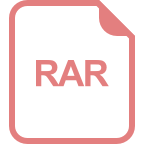






