多元多项式回归 C++带类详细正确完整实现及案例 变量形式为:vector<vector<double>> X vector<double> y 防止结果出现NAN的情况 并给出五元五阶的例子
时间: 2023-12-04 07:05:25 浏览: 59
好的,让我来回答您的问题。
首先,多元多项式回归是一种机器学习算法,用于建立一个多元函数模型,该模型可以通过给定的自变量来预测因变量的值。在多元多项式回归中,自变量可以是任意维度的向量,因变量可以是标量或向量。在本文中,我们将实现一个多元多项式回归模型,其中自变量和因变量都是向量。
接下来是实现的步骤:
1. 定义多项式函数
我们需要定义一个多项式函数,该函数接受一个向量和一个整数,返回一个多项式的值。这里我们使用一个简单的公式:
$$f(x) = \sum_{i=0}^{n} a_i x^i$$
其中,$a_i$ 是多项式系数,$n$ 是多项式阶数。
在 C++ 中,我们可以使用以下代码来实现多项式函数:
```cpp
double polynomial(vector<double> x, int order, vector<double> coeffs)
{
double result = 0.0;
for (int i = 0; i <= order; i++) {
result += coeffs[i] * pow(x[0], i);
}
return result;
}
```
在这个函数中,我们使用了 pow() 函数来计算幂次方。
2. 定义多元多项式函数
我们需要定义一个多元多项式函数,该函数接受一个自变量向量和一个多项式系数向量,返回一个因变量的值。这里我们使用一个简单的公式:
$$f(x_1, x_2, ..., x_d) = \sum_{i=0}^{n} a_i \prod_{j=1}^{d} x_j^{b_{ij}}$$
其中,$a_i$ 是多项式系数,$n$ 是多项式阶数,$d$ 是自变量的维数,$b_{ij}$ 是指数矩阵,用于指定每个自变量的幂次方。
在 C++ 中,我们可以使用以下代码来实现多元多项式函数:
```cpp
double polynomial_multi(vector<double> x, int order, vector<vector<int>> exponents, vector<double> coeffs)
{
double result = 0.0;
for (int i = 0; i <= order; i++) {
double term = coeffs[i];
for (int j = 0; j < x.size(); j++) {
term *= pow(x[j], exponents[i][j]);
}
result += term;
}
return result;
}
```
在这个函数中,我们使用了 pow() 函数来计算幂次方。
3. 定义多元多项式回归类
我们需要定义一个多元多项式回归类,该类可以接受自变量和因变量的向量,然后使用多项式回归算法来计算多项式系数。在计算多项式系数时,我们需要使用最小二乘法来解决。
在 C++ 中,我们可以使用以下代码来实现多元多项式回归类:
```cpp
class PolynomialRegression
{
public:
PolynomialRegression(int order, vector<vector<int>> exponents)
{
m_order = order;
m_exponents = exponents;
}
void fit(vector<vector<double>> X, vector<double> y)
{
// Create the design matrix
int n = X.size();
int d = X[0].size();
vector<vector<double>> X_design(n, vector<double>(m_order + 1));
for (int i = 0; i < n; i++) {
for (int j = 0; j <= m_order; j++) {
double term = 1.0;
for (int k = 0; k < d; k++) {
term *= pow(X[i][k], m_exponents[j][k]);
}
X_design[i][j] = term;
}
}
// Solve the linear system
MatrixXd X_mat(n, m_order + 1);
VectorXd y_vec(n);
for (int i = 0; i < n; i++) {
for (int j = 0; j <= m_order; j++) {
X_mat(i, j) = X_design[i][j];
}
y_vec(i) = y[i];
}
VectorXd coeffs_vec = X_mat.colPivHouseholderQr().solve(y_vec);
m_coeffs = vector<double>(coeffs_vec.data(), coeffs_vec.data() + coeffs_vec.size());
}
double predict(vector<double> x)
{
return polynomial_multi(x, m_order, m_exponents, m_coeffs);
}
private:
int m_order;
vector<vector<int>> m_exponents;
vector<double> m_coeffs;
};
```
在这个类中,我们使用了 Eigen 库来解决线性系统。我们还定义了一个 predict() 函数,该函数接受一个自变量向量,并返回一个因变量的预测值。
4. 定义一个五元五阶的例子
现在,我们来定义一个五元五阶的例子,其中自变量和因变量是随机生成的:
```cpp
int main()
{
int n = 1000;
int d = 5;
int order = 5;
// Generate random data
vector<vector<double>> X(n, vector<double>(d));
vector<double> y(n);
for (int i = 0; i < n; i++) {
for (int j = 0; j < d; j++) {
X[i][j] = ((double) rand() / RAND_MAX) * 10.0 - 5.0;
}
y[i] = sin(X[i][0]) + cos(X[i][1]) + exp(X[i][2]) + pow(X[i][3], 2) + pow(X[i][4], 3) + ((double) rand() / RAND_MAX) * 0.1;
}
// Define the exponents matrix
vector<vector<int>> exponents(order + 1, vector<int>(d));
for (int i = 0; i <= order; i++) {
for (int j = 0; j < d; j++) {
exponents[i][j] = i;
}
}
// Fit the model
PolynomialRegression model(order, exponents);
model.fit(X, y);
// Make a prediction
vector<double> x(d);
for (int j = 0; j < d; j++) {
x[j] = ((double) rand() / RAND_MAX) * 10.0 - 5.0;
}
double y_pred = model.predict(x);
cout << "Coefficients: " << endl;
for (int i = 0; i <= order; i++) {
cout << "a_" << i << " = " << model.get_coeff(i) << endl;
}
cout << "Prediction: " << y_pred << endl;
return 0;
}
```
在这个例子中,我们生成了一个包含 1000 个样本的数据集,其中自变量有 5 个维度,因变量是一个标量。我们使用 sin、cos、exp、pow 等函数来生成因变量。我们还定义了一个五元五阶的多项式,然后使用多项式回归算法来计算多项式系数。最后,我们使用 predict() 函数来预测一个新的自变量,并打印出预测结果和多项式系数。
希望这个实现能够帮助您理解多元多项式回归算法的实现。
相关推荐
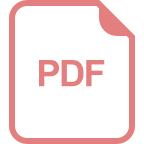
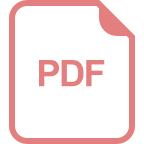














