nameIndex = np.argwhere(scoreAll == name) print(nameIndex) print('{}同学的平均成绩为{:.2f}'.format(name,np.mean(scoreNum[nameIndex[0,0]-1, 0:])))解释一下这段python代码
时间: 2024-03-07 22:51:00 浏览: 19
这段Python代码涉及到了NumPy库中的一些函数和操作。
首先,这段代码假设有一个名为`scoreAll`的NumPy数组,其中包含了所有学生的名字和成绩。其中,第一列是学生的名字,第二列是学生的成绩。假设`name`是想要查询平均成绩的学生的名字。
`nameIndex = np.argwhere(scoreAll == name)`这一行代码使用`np.argwhere()`函数在`scoreAll`数组中找到了所有等于`name`的元素(即学生名字为`name`的学生)。`argwhere()`函数返回的是一个数组,其中每个元素代表一个匹配项的索引位置。所以,`nameIndex`是一个二维数组,每个元素是一个长度为2的一维数组,代表了一个匹配项在`scoreAll`数组中的行号和列号。
接着,`print(nameIndex)`会输出所有匹配项的索引位置。
`print('{}同学的平均成绩为{:.2f}'.format(name,np.mean(scoreNum[nameIndex[0,0]-1, 0:])))`这一行代码使用了`np.mean()`函数来计算`nameIndex`对应的学生在`scoreAll`中的平均成绩。`scoreNum[nameIndex[0,0]-1, 0:]`这一部分代码取出了`scoreAll`数组中第`nameIndex`行对应的所有成绩数据。由于`nameIndex`是一个二维数组,所以要使用`nameIndex[0,0]`选取第一个匹配项的行号。由于`scoreAll`数组的第一行是学生名字,所以要将行号减去1。最后,`scoreNum[nameIndex[0,0]-1, 0:]`使用了切片语法`[0:]`,表示选取该行的所有列(即所有成绩数据)。
最后,`print()`函数输出了查询结果,其中`{}`和`{:.2f}`分别代表一个占位符,分别对应`name`和平均成绩的输出。`:.2f`表示保留2位小数的浮点数输出。
相关推荐
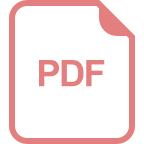
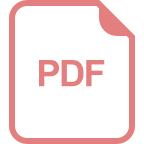














