pytorch双向lstm
时间: 2023-10-01 08:10:44 浏览: 86
PyTorch中的双向LSTM(Bidirectional LSTM)是一种常用的序列模型,可以用于处理自然语言处理任务,如文本分类、情感分析等。双向LSTM结合了前向LSTM和后向LSTM,可以更好地捕捉序列中的上下文信息。
在PyTorch中,你可以使用`torch.nn.LSTM`来构建双向LSTM模型。要实现双向性,你需要将两个LSTM层分别设置为前向和后向,并将它们串联在一起。
以下是一个简单的示例代码,展示了如何在PyTorch中构建一个双向LSTM模型:
```python
import torch
import torch.nn as nn
class BiLSTM(nn.Module):
def __init__(self, input_size, hidden_size, num_layers, num_classes):
super(BiLSTM, self).__init__()
self.hidden_size = hidden_size
self.num_layers = num_layers
self.lstm = nn.LSTM(input_size, hidden_size, num_layers, batch_first=True, bidirectional=True)
self.fc = nn.Linear(hidden_size * 2, num_classes) # *2因为前向和后向各有一个隐藏状态
def forward(self, x):
h0 = torch.zeros(self.num_layers * 2, x.size(0), self.hidden_size).to(x.device) # 初始化前向和后向隐藏状态
c0 = torch.zeros(self.num_layers * 2, x.size(0), self.hidden_size).to(x.device)
out, _ = self.lstm(x, (h0, c0)) # LSTM输出为out,_表示忽略最终的隐藏状态
out = self.fc(out[:, -1, :]) # 选择最后一个时间步的输出作为预测
return out
# 示例用法
input_size = 100 # 输入特征维度
hidden_size = 128 # 隐藏层大小
num_layers = 2 # LSTM层数
num_classes = 10 # 分类类别数
model = BiLSTM(input_size, hidden_size, num_layers, num_classes)
inputs = torch.randn(32, 10, input_size) # 输入数据形状为(batch_size, sequence_length, input_size)
outputs = model(inputs)
print(outputs.shape) # 输出形状为(batch_size, num_classes)
```
在这个示例中,我们首先定义了一个`BiLSTM`类继承自`nn.Module`,并在`__init__`方法中初始化了LSTM层和全连接层。在`forward`方法中,我们使用零张量初始化了前向和后向的隐藏状态,然后通过LSTM层进行前向传播。最后,我们选择最后一个时间步的输出,并通过全连接层将其映射到预测类别。
希望对你有所帮助!如果还有其他问题,请随时提问。
阅读全文
相关推荐
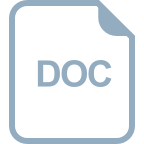
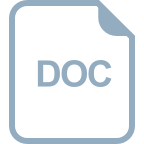
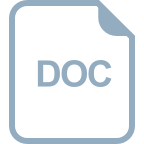




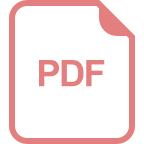
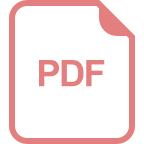
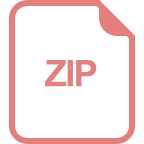
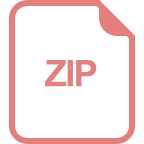
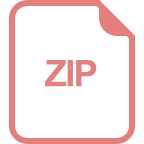
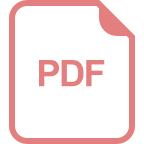





