return torch.batch_norm( RuntimeError: cuDNN error: CUDNN_STATUS_INTERNAL_ERROR
时间: 2023-07-21 14:08:56 浏览: 61
这个错误通常是由于cuDNN库引起的。cuDNN是一个用于深度学习的GPU加速库,它可能与你的PyTorch版本不兼容或者配置不正确。
你可以尝试以下解决方法:
1. 更新你的PyTorch版本:确保你正在使用最新版本的PyTorch。你可以使用以下命令更新PyTorch:
```
pip install --upgrade torch torchvision
```
2. 检查cuDNN的兼容性:确保你的cuDNN版本与你的PyTorch版本兼容。你可以在PyTorch的官方文档中找到cuDNN与PyTorch版本的兼容性表格。
3. 检查GPU驱动程序:确保你的GPU驱动程序已经正确安装并且与你的PyTorch版本兼容。你可以尝试更新或重新安装你的GPU驱动程序。
4. 检查CUDA版本:确保你的CUDA版本与你的PyTorch版本兼容。你可以在PyTorch的官方文档中找到CUDA与PyTorch版本的兼容性表格。
如果以上方法都没有解决问题,你可以尝试在PyTorch的论坛或社区中寻求帮助,或者提供更多详细的错误信息以便我们更好地帮助你解决问题。
相关问题
return torch.batch_norm( RuntimeError: CUDA error: out of memory
出现"RuntimeError: CUDA out of memory"错误是因为GPU显存内存不足。解决方法有以下几种:
1. 将batch_size改小:通过减小每个batch的大小,减少GPU显存的占用量。
2. 使用item()属性获取torch变量的标量值:在取出torch变量的标量值时,使用item()方法而不是直接输出变量本身,可以释放掉一些显存。
3. 添加代码以在测试阶段释放显存:在进行模型测试时,可以添加一些释放显存的代码,例如使用 torch.cuda.empty_cache() 来清理缓存。
如果需要同时利用其他GPU设备,可以按照以下方法进行配置:
1. 使用特定的GPU设备:可以使用 os.environ['CUDA_VISIBLE_DEVICES'] = "2" 来指定使用的GPU设备编号。
2. 使用多GPU并行处理:可以使用 torch.nn.DataParallel(model, device_ids=) 来指定多GPU并行处理时使用的设备编号。
请注意,在以上解决方法中,具体的设备编号和配置方式可能因实际情况不同而有所不同。
return torch.batch_norm( RuntimeError: Expected all tensors to be on the same device, but found at least two devices, cuda:0 and cpu! (
该报错是因为在运行中发现有至少两个设备,cuda:0和cpu上的张量。解决方法是将所有张量都放在同一个设备上运行,可以将其都改为在GPU上运算。
参考代码中没有提到具体的函数调用`return torch.batch_norm()`,所以无法针对该代码提供具体解决方法。如果你能提供更多关于这个报错的上下文信息,我可以为你提供更准确的解答。
相关推荐
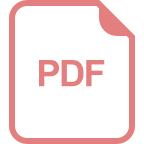
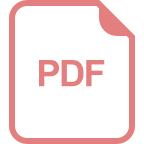
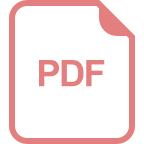













