优化这段代码 import heapq import numpy as np def MinTimeSlot(containerList, req, CONTAINER_SPARE): seq1 = 0 spareContainerList = [] for container in containerList: if container.appId == req.appId and container.state == CONTAINER_SPARE: seq1 += 1 heapq.heappush(spareContainerList, (-1 * container.killedTime, seq1, container)) return spareContainerList
时间: 2023-12-17 12:04:31 浏览: 37
这段代码的优化方案需要结合具体使用场景和数据规模来考虑。以下是几个可能的优化方案:
1. 使用列表推导式替代循环,可以增加代码的可读性和执行效率:
```
seq1 = 0
spareContainerList = [(-1 * container.killedTime, i, container) for i, container in enumerate(containerList) if container.appId == req.appId and container.state == CONTAINER_SPARE]
heapq.heapify(spareContainerList)
return spareContainerList
```
2. 如果列表中的元素数量很大,可以考虑使用生成器表达式和堆排序算法来节省内存和提高执行速度:
```
def MinTimeSlot(containerList, req, CONTAINER_SPARE):
spareContainerList = ((-1 * container.killedTime, i, container) for i, container in enumerate(containerList) if container.appId == req.appId and container.state == CONTAINER_SPARE)
return heapq.nsmallest(len(spareContainerList), spareContainerList)
```
3. 如果该函数需要频繁调用,可以将已经筛选出来的容器列表缓存起来,避免重复计算:
```
class ContainerCache:
def __init__(self, containerList):
self.containerDict = {}
for container in containerList:
if container.appId not in self.containerDict:
self.containerDict[container.appId] = []
if container.state == CONTAINER_SPARE:
self.containerDict[container.appId].append(container)
def get_spare_containers(self, appId):
return self.containerDict.get(appId, [])
containerCache = ContainerCache(containerList)
def MinTimeSlot(containerList, req, CONTAINER_SPARE, containerCache):
seq1 = 0
spareContainerList = []
for container in containerCache.get_spare_containers(req.appId):
seq1 += 1
heapq.heappush(spareContainerList, (-1 * container.killedTime, seq1, container))
return spareContainerList
```
相关推荐
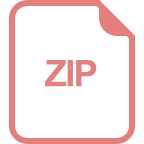
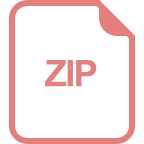
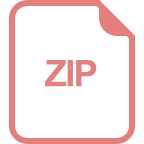















