上述代码中m = self.model[-1],请问m.m是什么
时间: 2024-04-18 15:25:28 浏览: 12
在上述代码中,m = self.model[-1]表示将self.model中的最后一个元素赋值给变量m。根据你提供的上下文,m可能是一个模型(model)的实例或模型中的某个模块。
根据代码中的语法,m.m可能是m模型中的一个属性或者子模块。具体的含义需要根据模型的定义和上下文来确定。你可以查看模型定义的代码,或者提供更多关于模型的信息,以便我能够给出更准确的回答。
相关问题
帮我看看这段代码报错原因: Traceback (most recent call last): File "/home/bder73002/hpy/ConvNextV2_Demo/train+.py", line 274, in <module> train_loss, train_acc = train(model_ft, DEVICE, train_loader, optimizer, epoch,model_ema) File "/home/bder73002/hpy/ConvNextV2_Demo/train+.py", line 48, in train loss = torch.nan_to_num(criterion_train(output, targets)) # 计算loss File "/home/bder73002/anaconda3/envs/python3.9.2/lib/python3.9/site-packages/torch/nn/modules/module.py", line 889, in _call_impl result = self.forward(*input, **kwargs) File "/home/bder73002/hpy/ConvNextV2_Demo/models/losses.py", line 38, in forward index.scatter_(1, target.data.view(-1, 1).type(torch.LongTensor), 1) RuntimeError: Expected index [128, 1] to be smaller than self [16, 8] apart from dimension 1 部分代码如下:cls_num_list = np.zeros(classes) for , label in train_loader.dataset: cls_num_list[label] += 1 criterion_train = LDAMLoss(cls_num_list=cls_num_list, max_m=0.5, s=30) class LDAMLoss(nn.Module): def __init__(self, cls_num_list, max_m=0.5, weight=None, s=30): super(LDAMLoss, self).__init__() m_list = 1.0 / np.sqrt(np.sqrt(cls_num_list)) m_list = m_list * (max_m / np.max(m_list)) m_list = torch.cuda.FloatTensor(m_list) self.m_list = m_list assert s > 0 self.s = s self.weight = weight def forward(self, x, target): index = torch.zeros_like(x, dtype=torch.uint8) # index.scatter_(1, target.data.view(-1, 1), 1) index.scatter_(1, target.data.view(-1, 1).type(torch.LongTensor), 1) index_float = index.type(torch.cuda.FloatTensor) batch_m = torch.matmul(self.m_list[None, :], index_float.transpose(0,1)) batch_m = batch_m.view((-1, 1)) x_m = x - batch_m output = torch.where(index, x_m, x) return F.cross_entropy(self.s*output, target, weight=self.weight)
这个错误是由于 `index` 张量的大小与 `output` 张量的大小不匹配导致的。在你的代码中,`index` 张量的大小是 `[batch_size, classes]`,即每个样本的预测标签的 one-hot 编码,而 `output` 张量的大小是 `[batch_size, features]`,即每个样本的特征向量的大小。因此,如果在 `index.scatter_()` 操作中使用了一个大小为 `[batch_size, 1]` 的张量,则会导致上述错误。
要解决这个问题,你可以将 `index` 的大小更改为 `[batch_size, num_classes]`,其中 `num_classes` 是分类数量。你可以在 `LDAMLoss` 的 `__init__` 方法中将 `num_classes` 作为参数并存储在实例变量中,然后在 `forward` 方法中使用它来创建 `index` 张量。例如:
```
class LDAMLoss(nn.Module):
def __init__(self, cls_num_list, max_m=0.5, weight=None, s=30, num_classes=10):
super(LDAMLoss, self).__init__()
self.num_classes = num_classes
m_list = 1.0 / np.sqrt(np.sqrt(cls_num_list))
m_list = m_list * (max_m / np.max(m_list))
m_list = torch.cuda.FloatTensor(m_list)
self.m_list = m_list
assert s > 0
self.s = s
self.weight = weight
def forward(self, x, target):
index = torch.zeros_like(x, dtype=torch.uint8)
index.scatter_(1, target.data.view(-1, 1).type(torch.LongTensor), 1)
index = index[:, :self.num_classes] # 取前 num_classes 列
index_float = index.type(torch.cuda.FloatTensor)
batch_m = torch.matmul(self.m_list[None, :], index_float.transpose(0,1))
batch_m = batch_m.view((-1, 1))
x_m = x - batch_m
output = torch.where(index, x_m, x)
return F.cross_entropy(self.s*output, target, weight=self.weight)
```
然后在使用 `LDAMLoss` 时,你需要将 `num_classes` 参数传递给它。例如:
```
num_classes = len(train_loader.dataset.classes)
criterion_train = LDAMLoss(cls_num_list=cls_num_list, max_m=0.5, s=30, num_classes=num_classes)
```
上述211行附近的代码如下,请具体指出问题 def build_targets(self, p, targets): # Build targets for compute_loss(), input targets(image,class,x,y,w,h) na, nt = self.na, targets.shape[0] # number of anchors, targets tcls, tbox, indices, anch = [], [], [], [] gain = torch.ones(7, device=targets.device) # normalized to gridspace gain ai = torch.arange(na, device=targets.device).float().view(na, 1).repeat(1, nt) # same as .repeat_interleave(nt) targets = torch.cat((targets.repeat(na, 1, 1), ai[:, :, None]), 2) # append anchor indices g = 0.5 # bias off = torch.tensor([[0, 0], [1, 0], [0, 1], [-1, 0], [0, -1], # j,k,l,m # [1, 1], [1, -1], [-1, 1], [-1, -1], # jk,jm,lk,lm ], device=targets.device).float() * g # offsets for i in range(self.nl): anchors = self.anchors[i] gain[2:6] = torch.tensor(p[i].shape)[[3, 2, 3, 2]] # xyxy gain # Match targets to anchors t = targets * gain if nt: # Matches r = t[:, :, 4:6] / anchors[:, None] # wh ratio j = torch.max(r, 1. / r).max(2)[0] < self.hyp['anchor_t'] # compare # j = wh_iou(anchors, t[:, 4:6]) > model.hyp['iou_t'] # iou(3,n)=wh_iou(anchors(3,2), gwh(n,2)) t = t[j] # filter # Offsets gxy = t[:, 2:4] # grid xy gxi = gain[[2, 3]] - gxy # inverse j, k = ((gxy % 1. < g) & (gxy > 1.)).T l, m = ((gxi % 1. < g) & (gxi > 1.)).T j = torch.stack((torch.ones_like(j), j, k, l, m)) t = t.repeat((5, 1, 1))[j] offsets = (torch.zeros_like(gxy)[None] + off[:, None])[j] else: t = targets[0] offsets = 0 # Define b, c = t[:, :2].long().T # image, class gxy = t[:, 2:4] # grid xy gwh = t[:, 4:6] # grid wh gij = (gxy - offsets).long() gi, gj = gij.T # grid xy indices # Append a = t[:, 6].long() # anchor indices indices.append((b, a, gj.clamp_(0, gain[3] - 1), gi.clamp_(0, gain[2] - 1))) # image, anchor, grid indices tbox.append(torch.cat((gxy - gij, gwh), 1)) # box anch.append(anchors[a]) # anchors tcls.append(c) # class return tcls, tbox, indices, anch
根据你提供的代码,第 211 行出现错误的地方是在 indices.append((b, a, gj.clamp_(0, gain[3] - 1), gi.clamp_(0, gain[2] - 1))) 这一行。
问题出现在使用 clamp_() 函数时,尝试将 Float 类型的 gj 和 gi 转换为 __int64 类型。由于 clamp_() 函数会直接修改张量的值,而且 __int64 类型不能容纳 Float 类型的值,因此会导致类型转换错误。
要解决这个问题,你可以使用 clamp() 函数而不是 clamp_() 函数,这样就可以返回一个新的张量,而不会修改原始张量的值。修改代码如下:
```python
indices.append((b, a, gj.clamp(0, int(gain[3]) - 1), gi.clamp(0, int(gain[2]) - 1))) # image, anchor, grid indices
```
这样修改后,应该就能解决 RuntimeError: result type Float can't be cast to the desired output type __int64 的问题了。
相关推荐
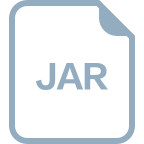
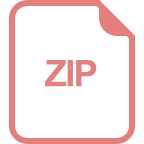
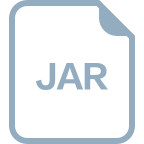












