报错原因pca = PCA(n_components=3) X_pca = pca.fit_transform(X) from pyecharts import Scatter3D import os #导入系统库是为了打开生成的html文件 scatter3D = Scatter3D("PCA特征分布") scatter3D.add("", X_pca) # 生成本地 HTML 文件 scatter3D.render("render3.html") # 打开生成的本地 HTML 文件 os.system("render3.html")
时间: 2024-03-29 07:37:10 浏览: 197
根据你提供的代码和错误提示,可能出现报错的原因有以下几种可能:
1. 缺少必要的库或者依赖项。你需要确保已经正确安装了scikit-learn和pyecharts库,并且安装了这些库所依赖的其他库。
2. 数据中存在缺失值NaN。PCA类的fit_transform()方法默认会对数据中的缺失值进行均值估计并填充缺失值。但是,如果数据中存在大量的缺失值,可能会导致PCA计算失败。因此,你需要检查数据中是否存在缺失值,如果存在缺失值,可以考虑删除或填充缺失值。
3. 数据的格式不正确。PCA类的fit_transform()方法要求输入的数据矩阵X为C-ordered数组,即按行进行存储。如果数据不满足该要求,需要使用numpy库的ascontiguousarray()函数进行转换。你需要检查输入的数据格式是否正确,并使用ascontiguousarray()函数进行转换。
4. 可能是render3.html文件还没有生成,就执行了打开操作。你可以尝试在打开文件之前等待一段时间,或者手动打开render3.html文件来检查是否生成了正确的图表。
你可以根据以上可能的原因逐一排查,看看哪一个可能是造成报错的原因。
相关问题
pca = PCA(n_components=2) X_pca = pca.fit_transform(pred_images),如何用散点图可视化啊
要使用散点图可视化降维后的数据,你可以将降维后的数据`X_pca`作为横纵坐标,然后使用散点图函数进行绘制。
以下是一个示例代码:
```python
import matplotlib.pyplot as plt
# 绘制散点图
plt.scatter(X_pca[:, 0], X_pca[:, 1])
plt.xlabel('PC1')
plt.ylabel('PC2')
plt.title('PCA Scatter Plot')
plt.show()
```
这段代码使用Matplotlib库绘制散点图。`X_pca[:, 0]`和`X_pca[:, 1]`分别表示降维后的数据在第一个和第二个主成分上的投影,它们作为横纵坐标传递给`plt.scatter()`函数。
为了给散点图添加标签和标题,使用`plt.xlabel()`和`plt.ylabel()`指定坐标轴的标签,使用`plt.title()`指定图表的标题。
最后,使用`plt.show()`函数显示绘制的散点图。
你可以根据需要进一步自定义散点图的样式,例如添加颜色、大小、图例等。
import matplotlib.pyplot as plt from sklearn.decomposition import PCA # 进行PCA降维 pca = PCA(n_components=2) x_pca = pca.fit_transform(x) # 绘制散点图 fig, (ax1, ax2) = plt.subplots(1, 2, figsize=(10, 5)) ax1.scatter(x_pca[:, 0], x_pca[:, 1], c=y) ax1.set_title('Original dataset') # 进行ADASYN过采样 adasyn = ADASYN(random_state=42) x_resampled, y_resampled = adasyn.fit_resample(x, y) # 进行PCA降维 x_resampled_pca = pca.transform(x_resampled) # 绘制散点图 ax2.scatter(x_resampled_pca[:, 0], x_resampled_pca[:, 1], c=y_resampled) ax2.set_title('Resampled dataset') plt.show()把点弄小一点
你可以调整`scatter`函数中的`s`参数来改变散点的大小。例如,将`s`设置为`10`可以使散点变得更小:
```python
import matplotlib.pyplot as plt
from sklearn.decomposition import PCA
# 进行PCA降维
pca = PCA(n_components=2)
x_pca = pca.fit_transform(x)
# 绘制散点图
fig, (ax1, ax2) = plt.subplots(1, 2, figsize=(10, 5))
ax1.scatter(x_pca[:, 0], x_pca[:, 1], c=y, s=10)
ax1.set_title('Original dataset')
# 进行ADASYN过采样
adasyn = ADASYN(random_state=42)
x_resampled, y_resampled = adasyn.fit_resample(x, y)
# 进行PCA降维
x_resampled_pca = pca.transform(x_resampled)
# 绘制散点图
ax2.scatter(x_resampled_pca[:, 0], x_resampled_pca[:, 1], c=y_resampled, s=10)
ax2.set_title('Resampled dataset')
plt.show()
```
该代码将原始数据集和ADASYN过采样后的数据集投影到二维平面上,并使用散点图进行可视化比较。其中,原始数据集的样本点用不同颜色进行区分,而过采样后的样本点则全部用相同颜色表示。所有散点的大小都设置为了`10`。你可以根据需要调整`s`参数的大小。
阅读全文
相关推荐
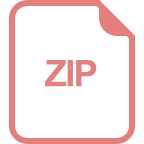
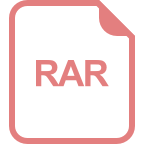
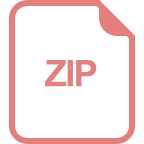













