用folat不能为0.9怎么解决
时间: 2024-03-17 11:47:50 浏览: 77
在计算机中,由于浮点数的存储方式和精度限制,可能会导致浮点数的表示和计算结果与预期不同。这也是为什么一些比较操作不能直接使用等号比较,而需要使用一个误差范围来判断两个浮点数是否相等。
如果你需要使用0.9这个特定的浮点数,可以考虑使用一个误差范围来比较它和其他浮点数之间的关系,例如:
```
float x = 0.9;
float epsilon = 0.0001; // 误差范围
if (fabs(x - 0.9) < epsilon) {
// x 和 0.9 相等
}
```
其中,`fabs()`函数用于求一个浮点数的绝对值,可以避免出现负数误差的情况。通过调整`epsilon`的值,可以控制误差范围的大小。
相关问题
ValueError: could not convert string '0\t0\t0.3\t0.6\t0.9\t1.2\t1.5\t1.8\t2.1' to float64 at row 0, column 1.
这个错误指出在第一行的第二列无法将字符串'0\t0\t0.3\t0.6\t0.9\t1.2\t1.5\t1.8\t2.1'转换为float64。这可能是由于字符串中包含了制表符(\t)而不是标准的数字。为了解决这个问题,你可以尝试使用Python的字符串处理方法,如split()函数来分割字符串,并将字符串转换为浮点数。下面是一个示例代码段,展示了如何处理这个错误:
```
# 假设你的字符串是'0\t0\t0.3\t0.6\t0.9\t1.2\t1.5\t1.8\t2.1'
string = '0\t0\t0.3\t0.6\t0.9\t1.2\t1.5\t1.8\t2.1'
values = string.split('\t')
float_values = [float(value) for value in values]
```
你可以尝试使用这个代码段来处理你的字符串,并将其转换为浮点数。这样就可以避免这个错误了。
写代码用蚁群算法解决旅行商问题
以下是使用蚁群算法解决旅行商问题的Python代码:
```python
import random
import math
class Ant:
def __init__(self, start_city, num_cities, pheromone_matrix, distance_matrix, alpha=1.0, beta=3.0, q0=0.9):
self.start_city = start_city
self.current_city = start_city
self.visited_cities = [start_city]
self.num_cities = num_cities
self.pheromone_matrix = pheromone_matrix
self.distance_matrix = distance_matrix
self.alpha = alpha
self.beta = beta
self.q0 = q0
def select_next_city(self):
probabilities = []
total_prob = 0.0
q = random.uniform(0, 1)
if q < self.q0:
max_prob = 0.0
max_city = None
for city in range(self.num_cities):
if city not in self.visited_cities:
prob = self.pheromone_matrix[self.current_city][city] ** self.alpha * \
(1.0 / self.distance_matrix[self.current_city][city]) ** self.beta
if prob > max_prob:
max_prob = prob
max_city = city
next_city = max_city
else:
for city in range(self.num_cities):
if city not in self.visited_cities:
prob = self.pheromone_matrix[self.current_city][city] ** self.alpha * \
(1.0 / self.distance_matrix[self.current_city][city]) ** self.beta
probabilities.append((city, prob))
total_prob += prob
if total_prob == 0.0:
next_city = None
else:
probabilities = [(city, prob / total_prob) for city, prob in probabilities]
next_city = self.roulette_wheel(probabilities)
return next_city
def roulette_wheel(self, probabilities):
r = random.uniform(0, 1)
cumulative_prob = 0.0
for city, prob in probabilities:
cumulative_prob += prob
if cumulative_prob >= r:
return city
assert False, 'Should not reach here'
def travel(self):
while len(self.visited_cities) < self.num_cities:
next_city = self.select_next_city()
if next_city is None:
break
self.visited_cities.append(next_city)
self.current_city = next_city
def tour_length(self):
tour_len = 0.0
for i in range(self.num_cities):
tour_len += self.distance_matrix[self.visited_cities[i - 1]][self.visited_cities[i]]
return tour_len
class ACO:
def __init__(self, num_ants, num_iterations, num_cities, distance_matrix, alpha=1.0, beta=3.0, rho=0.1, q0=0.9):
self.num_ants = num_ants
self.num_iterations = num_iterations
self.num_cities = num_cities
self.distance_matrix = distance_matrix
self.alpha = alpha
self.beta = beta
self.rho = rho
self.q0 = q0
self.pheromone_matrix = [[1.0 / num_cities for j in range(num_cities)] for i in range(num_cities)]
def run(self):
best_tour_len = float('inf')
best_tour = None
for i in range(self.num_iterations):
ants = [Ant(random.randint(0, self.num_cities - 1), self.num_cities, self.pheromone_matrix,
self.distance_matrix, self.alpha, self.beta, self.q0) for j in range(self.num_ants)]
for ant in ants:
ant.travel()
tour_len = ant.tour_length()
if tour_len < best_tour_len:
best_tour_len = tour_len
best_tour = ant.visited_cities
for i in range(self.num_cities):
j = (i + 1) % self.num_cities
self.pheromone_matrix[ant.visited_cities[i]][ant.visited_cities[j]] *= 1.0 - self.rho
self.pheromone_matrix[ant.visited_cities[i]][ant.visited_cities[j]] += self.rho / tour_len
return best_tour, best_tour_len
if __name__ == '__main__':
# Example usage
num_cities = 10
coords = []
for i in range(num_cities):
x = random.uniform(0, 1)
y = random.uniform(0, 1)
coords.append((x, y))
distance_matrix = [[0.0 for j in range(num_cities)] for i in range(num_cities)]
for i in range(num_cities):
for j in range(num_cities):
if i != j:
dx = coords[i][0] - coords[j][0]
dy = coords[i][1] - coords[j][1]
distance_matrix[i][j] = math.sqrt(dx ** 2 + dy ** 2)
aco = ACO(num_ants=10, num_iterations=100, num_cities=num_cities, distance_matrix=distance_matrix)
best_tour, best_tour_len = aco.run()
print('Best tour:', best_tour)
print('Best tour length:', best_tour_len)
```
该代码通过随机生成城市坐标,并计算两两城市之间的距离来构造旅行商问题的数据。然后使用蚁群算法来寻找最优解,其中包括了Ant和ACO两个类,分别实现了蚂蚁和蚁群算法的相关操作。最终输出最优解及其长度。
相关推荐
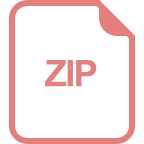
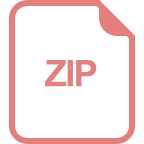













