详细解释:// 1. get camera intrinsic and pose base::BaseCameraModelPtr camera_model = QueryCameraModel(camera); if (camera_model == nullptr) { AERROR << "Failed to get camera model for " << camera->GetSensorId(); return distance; } Eigen::Matrix4d world2camera_pose; if (!QueryWorld2CameraPose(camera, &world2camera_pose)) { AERROR << "Failed to query camera pose"; return distance; } Eigen::Matrix4d lidar2world_pose; if (!QueryLidar2WorldPose(lidar, &lidar2world_pose)) { AERROR << "Failed to query lidar pose"; return distance; } Eigen::Matrix4d lidar2camera_pose = static_cast<Eigen::Matrix<double, 4, 4, 0, 4, 4>>(world2camera_pose * lidar2world_pose);
时间: 2024-04-27 13:25:16 浏览: 129
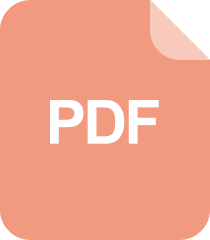
Camera Calibration
这段代码主要实现了获取相机内参和位姿,以及获取激光雷达到相机的变换矩阵。具体步骤如下:
1. 先通过传入的相机对象获取相机的内参,即相机的焦距、像素中心点等参数,这里调用了QueryCameraModel函数,返回的是一个BaseCameraModelPtr类型的智能指针,指向了相机模型对象。
2. 如果获取相机模型对象失败,则输出错误信息并返回distance(距离)。
3. 接着调用QueryWorld2CameraPose函数获取相机在世界坐标系下的位姿,即相机的位置和朝向。
4. 如果获取相机位姿失败,则输出错误信息并返回distance。
5. 接着调用QueryLidar2WorldPose函数获取激光雷达在世界坐标系下的位姿。
6. 如果获取激光雷达位姿失败,则输出错误信息并返回distance。
7. 计算激光雷达到相机的变换矩阵,即lidar2camera_pose,这里将相机的位姿和激光雷达的位姿进行矩阵相乘得到。
8. 最后返回distance。
阅读全文
相关推荐
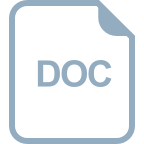
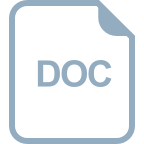














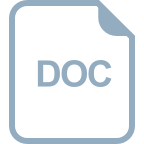