现有一个位置-结构-图卷积神经网络,对其加ARMA滤波器实现卷积,怎么写方法
时间: 2023-07-20 12:06:31 浏览: 159
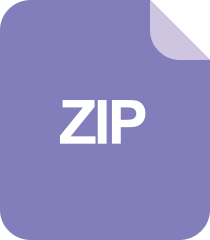
用卷积滤波器matlab代码-Steganalysis:卷积神经网络在隐写分析中的应用
假设你已经有了一个位置-结构-图卷积神经网络的代码,现在要对其加上ARMA滤波器实现卷积,可以按照以下步骤进行:
1. 导入所需的库
```python
import torch
from torch_geometric.nn import ARMAConv
```
2. 定义ARMAConv卷积层
```python
class ARMAConvNet(torch.nn.Module):
def __init__(self, num_features, num_classes):
super(ARMAConvNet, self).__init__()
self.conv1 = ARMAConv(num_features, 16, 3, 2)
self.conv2 = ARMAConv(16, 32, 3, 2)
self.conv3 = ARMAConv(32, 64, 3, 2)
self.fc1 = torch.nn.Linear(64, 128)
self.fc2 = torch.nn.Linear(128, num_classes)
def forward(self, x, edge_index, edge_attr):
x = self.conv1(x, edge_index, edge_attr)
x = torch.nn.functional.relu(x)
x = self.conv2(x, edge_index, edge_attr)
x = torch.nn.functional.relu(x)
x = self.conv3(x, edge_index, edge_attr)
x = torch.nn.functional.relu(x)
x = torch.nn.functional.avg_pool2d(x, kernel_size=x.size()[2:])
x = x.view(-1, 64)
x = self.fc1(x)
x = torch.nn.functional.relu(x)
x = self.fc2(x)
return x
```
其中,ARMAConv的参数依次为:输入特征数、输出特征数、滤波器大小、步长。
3. 训练模型
```python
model = ARMAConvNet(num_features, num_classes)
optimizer = torch.optim.Adam(model.parameters(), lr=0.01, weight_decay=5e-4)
criterion = torch.nn.CrossEntropyLoss()
for epoch in range(num_epochs):
model.train()
optimizer.zero_grad()
out = model(data.x, data.edge_index, data.edge_attr)
loss = criterion(out, data.y)
loss.backward()
optimizer.step()
model.eval()
out = model(data.x, data.edge_index, data.edge_attr)
pred = out.argmax(dim=1)
acc = pred.eq(data.y).sum().item() / len(data.y)
print(f'Epoch: {epoch}, Loss: {loss:.4f}, Accuracy: {acc:.4f}')
```
其中,data为输入数据,包括节点特征、边索引和边属性。
通过以上步骤,就可以将ARMA滤波器加入位置-结构-图卷积神经网络中实现卷积操作。
阅读全文
相关推荐





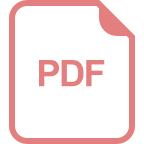
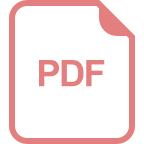
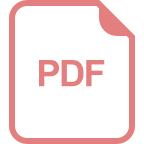
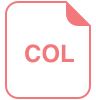
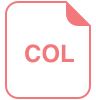
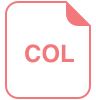
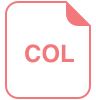
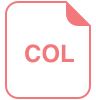
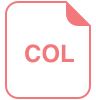
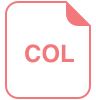
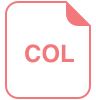