A.append([x_coefficient, y_coefficient]) B.append([b]) B = np.array(B) A_pseudo = np.linalg.pinv(A)
时间: 2023-06-25 08:01:47 浏览: 171
这段代码是在进行矩阵运算,具体来说是在求解一个线性方程组的解。首先,将系数矩阵 A 中的每一行的 x 系数和 y 系数以列表形式存储在 A 列表中,每个方程的常数 b 存储在 B 列表中。然后,将 B 列表转换为 NumPy 数组。接下来,使用 numpy.linalg.pinv() 函数计算矩阵 A 的伪逆(也称为 Moore-Penrose 逆),得到 A_pseudo 矩阵。最后,通过矩阵乘法 np.dot(A_pseudo, B) 计算出线性方程组 Ax=b 的解。
相关问题
def trilaterate2D(self): A = [] B = [] # trilateration using SVD for idx in range(4): if idx == 0: # i:1 j:4 x_coefficient = self.position[3][0] - self.position[idx][0] # x1-xidx y_coefficient = self.position[3][1] - self.position[idx][1] # y1-yidx b = 1 / 2 * (self.distances[idx] ** 2 - self.distances[3] ** 2 - ((self.position[idx][0] - self.position[3][0]) ** 2 + ( self.position[idx][1] - self.position[3][1]) ** 2)) \ + x_coefficient * self.position[3][0] + y_coefficient * self.position[3][1] A.append([x_coefficient, y_coefficient]) B.append([b]) else: x_coefficient = self.position[0][0] - self.position[idx][0] # x1-xidx y_coefficient = self.position[0][1] - self.position[idx][1] # y1-yidx b = 1 / 2 * (self.distances[idx] ** 2 - self.distances[0] ** 2 - ((self.position[idx][0] - self.position[0][0]) ** 2 + ( self.position[idx][1] - self.position[0][1]) ** 2)) \ + x_coefficient * self.position[0][0] + y_coefficient * self.position[0][1] A.append([x_coefficient, y_coefficient]) B.append([b]) B = np.array(B) A_pseudo = np.linalg.pinv(A) self.result = np.dot(A_pseudo, B) result_x = self.result[0] result_y = self.result[1]
这段代码是用于进行二维三点定位的。其中,A和B分别代表了方程组Ax=B中的系数矩阵和常数矩阵。通过奇异值分解(SVD)的方法求解该方程组,得到位置的坐标值。具体地,该方法通过计算三个定位点与目标点之间的距离,以及三个定位点的坐标值,构建一个二次方程组来求解目标点的位置坐标。这种方法可以应用于无线传感器网络等领域中的目标定位问题。
import numpy as np import math import matplotlib.pyplot as plt import tkinter as tk import tkinter.messagebox import pandas as pd from openpyxl import load_workbook from warnings import simplefilter engine_torque = 10 i0 = 2.088 i1 = 2.928 ig = 2.929 efficiency = 0.96 Wheel_radius = 0.3059 slope = 0 #坡度单位弧度 slope_cos = math.cos(slope) slope_sin = math.sin(slope) rolling_resistance_coefficient = 0.01 air_coefficient = 0.28 face_area = 0.4 air_density = 1.2258 vehicle_speed = 0 weight = 268 step_size = 0.01 flag = 0 time = 0 vehicle_speed_plot = [] time_plot = [] def drive_force(engine_torque,i0,i1,ig,efficiency,Wheel_radius): drive_force = engine_torque*i0*ig*i1*efficiency/Wheel_radius return drive_force def rolling_resistance(weight,rolling_resistance_coefficient,slope_cos): rolling_resistance = weight*rolling_resistance_coefficient*slope_cos return rolling_resistance def air_resistance(air_coefficient,face_area,air_density,relative_speed): air_resistance = 0.5*air_coefficient*face_area*air_density*relative_speed*relative_speed return air_resistance def grade_resistance(weight,slope_sin): grade_resistance = weight*slope_sin return grade_resistance while flag==0: relative_speed = vehicle_speed vehicle_acclerate = (drive_force(engine_torque,i0,i1,ig,efficiency,Wheel_radius)-rolling_resistance(weight,rolling_resistance_coefficient,slope_cos)-air_resistance(air_coefficient,face_area,air_density,relative_speed))/weight vehicle_speed = vehicle_acclerate*step_size+vehicle_speed running_distance = relative_speed*step_size+0.5*vehicle_acclerate*step_size*step_size time = time+step_size if time == 10: flag = 1 vehicle_speed_plot.append(vehicle_speed) time_plot.append(time)
这段代码使用了多个变量和函数来计算车辆在直线道路上的行驶情况。具体来说,代码的主要功能是根据车辆的动力、阻力和坡度等因素来计算车辆在直线道路上的运动状态,并将结果存储在列表中以便后续使用。
代码中的变量和函数的含义如下:
- engine_torque:发动机扭矩
- i0、i1、ig:传动系数
- efficiency:传动效率
- Wheel_radius:车轮半径
- slope:坡度
- slope_cos、slope_sin:坡度的余弦值和正弦值
- rolling_resistance_coefficient:滚动阻力系数
- air_coefficient:空气阻力系数
- face_area:车辆正面面积
- air_density:空气密度
- vehicle_speed:车辆速度
- weight:车辆重量
- step_size:时间步长
- flag:循环标志,初始值为 0
- time:当前时间,初始值为 0
- vehicle_speed_plot:车辆速度列表
- time_plot:时间列表
代码中的函数包括:
- drive_force:计算车辆的驱动力
- rolling_resistance:计算车辆的滚动阻力
- air_resistance:计算车辆的空气阻力
- grade_resistance:计算车辆的坡度阻力
代码的主体部分是一个 while 循环,当 flag 等于 0 时,会一直循环下去。每次循环时,根据车辆的动力、阻力和坡度等因素来计算车辆的速度和加速度,并更新列表中的数据。当时间达到 10 秒时,循环停止。
最后,代码中使用了 numpy、math、matplotlib.pyplot、tkinter、pandas 和 openpyxl 等模块来实现各种功能。
阅读全文
相关推荐
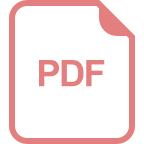
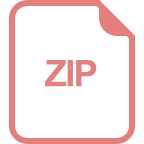
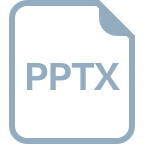














