def get_samples_ini(batch_size=100): x = torch.rand(batch_size) p0 = torch.sin(2. * np.pi * x).unsqueeze(1) return torch.stack([ x, torch.zeros(batch_size) # t=0 ], axis=-1), p0
时间: 2023-05-26 12:06:15 浏览: 127
这段代码实现了一个函数,函数名为get_samples_ini,输入参数为batch_size,默认为100。在函数内部,首先使用torch.rand生成了一个大小为batch_size的随机数x;然后使用torch.sin和np.pi对x进行一些数学运算,生成另一个张量p0;最后,将x和一个大小为(batch_size,1)的全零张量torch.zeros进行torch.stack操作,返回一个大小为(2,batch_size)的张量。
相关问题
class DistributedSampler(_DistributedSampler): def __init__(self, dataset, num_replicas=None, rank=None, shuffle=True): super().__init__(dataset, num_replicas=num_replicas, rank=rank) self.shuffle = shuffle def __iter__(self): if self.shuffle: g = torch.Generator() g.manual_seed(self.epoch) indices = torch.randperm(len(self.dataset), generator=g).tolist() else: indices = torch.arange(len(self.dataset)).tolist() indices += indices[:(self.total_size - len(indices))] assert len(indices) == self.total_size indices = indices[self.rank:self.total_size:self.num_replicas] assert len(indices) == self.num_samples return iter(indices) def build_dataloader(dataset_cfg, class_names, batch_size, dist, root_path=None, workers=4, seed=None, logger=None, training=True, merge_all_iters_to_one_epoch=False, total_epochs=0): dataset = __all__[dataset_cfg.DATASET]( dataset_cfg=dataset_cfg, class_names=class_names, root_path=root_path, training=training, logger=logger, ) if merge_all_iters_to_one_epoch: assert hasattr(dataset, 'merge_all_iters_to_one_epoch') dataset.merge_all_iters_to_one_epoch(merge=True, epochs=total_epochs) if dist: if training: sampler = torch.utils.data.distributed.DistributedSampler(dataset) else: rank, world_size = common_utils.get_dist_info() sampler = DistributedSampler(dataset, world_size, rank, shuffle=False) else: sampler = None dataloader = DataLoader( dataset, batch_size=batch_size, pin_memory=True, num_workers=workers, shuffle=(sampler is None) and training, collate_fn=dataset.collate_batch, drop_last=False, sampler=sampler, timeout=0, worker_init_fn=partial(common_utils.worker_init_fn, seed=seed) ) return dataset, dataloader, sampler
这段代码是一个用于构建数据加载器的函数。它接受一些参数,包括数据集的配置、类别名称、批次大小、分布式训练标志、数据集的根路径等。
首先,根据数据集的配置和其他参数,创建一个数据集对象。
如果设置了 `merge_all_iters_to_one_epoch` 标志为 `True`,则调用数据集对象的 `merge_all_iters_to_one_epoch` 方法,将所有迭代器合并到一个周期中。
接下来,如果分布式训练标志为 `True`,则根据训练模式创建相应的采样器。对于训练模式,使用 `torch.utils.data.distributed.DistributedSampler` 创建采样器;对于非训练模式,根据当前进程的排名和世界大小创建 `DistributedSampler` 采样器,并设置 `shuffle` 参数为 `False`。
如果不是分布式训练,则采样器为 `None`。
最后,使用 `torch.utils.data.DataLoader` 创建数据加载器,传入数据集对象、批次大小、是否在训练模式下洗牌、数据集对象的 `collate_batch` 方法用于批量整理数据、是否丢弃最后一个批次、采样器以及其他参数。
函数返回数据集对象、数据加载器和采样器。
解读这段代码class randomSequentialSampler(sampler.Sampler): def __init__(self, data_source, batch_size): self.num_samples = len(data_source) self.batch_size = batch_size def __iter__(self): n_batch = len(self) // self.batch_size tail = len(self) % self.batch_size index = torch.LongTensor(len(self)).fill_(0) for i in range(n_batch): random_start = random.randint(0, len(self) - self.batch_size) batch_index = random_start + torch.range(0, self.batch_size - 1) index[i * self.batch_size:(i + 1) * self.batch_size] = batch_index # deal with tail if tail: random_start = random.randint(0, len(self) - self.batch_size) tail_index = random_start + torch.range(0, tail - 1) index[(i + 1) * self.batch_size:] = tail_index return iter(index) def __len__(self): return self.num_samples
这是一个自定义的 PyTorch 数据集采样器,用于在训练神经网络时对数据进行随机采样。具体来说:
- `randomSequentialSampler` 继承了 PyTorch 中的 `sampler.Sampler` 类,实现了 `__init__`、`__iter__` 和 `__len__` 方法。
- `__init__` 方法初始化了数据集的大小和每个 batch 的大小。
- `__iter__` 方法返回一个迭代器,用于遍历所有的样本,其中:
- `n_batch` 表示数据集中 batch 的数量。
- `tail` 表示剩余样本数。
- `index` 是一个长度为数据集大小的 LongTensor,用于存放样本下标。
- 通过循环,对每个 batch 随机选择起始样本下标,并将 batch 中每个样本的下标存储到 `index` 中。
- 处理剩余的不足一整个 batch 的样本,方法同上。
- 返回一个迭代器,用于遍历 `index` 中的所有样本下标。
- `__len__` 方法返回数据集的大小。
这个采样器的作用是将数据集中的样本随机分成若干个 batch,每个 batch 的大小由用户指定,且每个 batch 中的样本顺序也是随机的。这种采样方式可以增加数据集的多样性,提高模型的泛化能力。
阅读全文
相关推荐
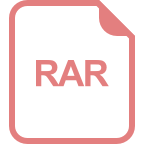
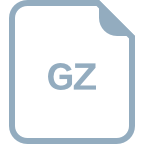














