sensitivity = np.abs(FIM) / np.sqrt()
时间: 2024-02-06 22:03:35 浏览: 179
在计算灵敏度时,代码中使用了np.sqrt()函数,但是函数的参数缺失,导致代码报错。
np.sqrt()函数是用于计算平方根的函数,需要在括号中指定要求平方根的数值。在这个代码中的灵敏度计算中,需要使用数据敏感度sensitivity来计算灵敏度,因此可以将其作为np.sqrt()函数的参数。
以下是修正后的代码示例:
```python
import numpy as np
def laplas(FIM, epsilon):
k = FIM.shape[0]
# 给定隐私预算 epsilon
# 计算给定隐私预算时的拉普拉斯机制的参数的最小值
# 计算每个系数的灵敏度
sensitivity = np.abs(FIM) / np.sqrt(epsilon)
# 计算拉普拉斯机制的参数
a = np.min(sensitivity) / epsilon
return a
# 示例
FIM = np.random.rand(5, 5) # Fisher信息矩阵
epsilon = 0.1 # 隐私预算
a = laplas(FIM, epsilon)
print("拉普拉斯参数的最小值:", a)
```
在这个代码示例中,我们将epsilon作为np.sqrt()函数的参数,用于计算每个系数的灵敏度。这样,就可以正常计算灵敏度,并根据给定的隐私预算计算拉普拉斯参数的最小值了。
相关问题
def add_noise(image, epsilon, k): f = np.fft.fft2(image) fshift = np.fft.fftshift(f) rows, cols = image.shape b = laplas(fshift, epsilon, k) # print(b) p = 0.5 noise = np.random.laplace(scale=b, size=(rows, cols)) + np.mean(f) * p image_noise = fshift + noise f_ishift = np.fft.ifftshift(image_noise) image_back = np.fft.ifft2(f_ishift) image_back = np.real(image_back) return image_back def laplas(FIM, epsilon, k): FIM_k = FIM[:k, :k] sensitivity = np.abs(FIM_k) / np.sqrt(epsilon) sensitivity2 = np.abs(FIM) / np.sqrt(epsilon) scale = sensitivity2 / epsilon delta_f = np.max(np.sqrt(np.real(FIM[:k, :k]) ** 2 + np.imag(FIM[:k, :k]) ** 2)) - np.min( np.sqrt(np.real(FIM[:k, :k]) ** 2 + np.imag(FIM[:k, :k]) ** 2)) c = delta_f / epsilon d = delta_f * math.sqrt(2 * math.log(1.2 / 0.1)) / epsilon return d def add_noisy_image(): image = cv2.imread("image.jpg", cv2.IMREAD_GRAYSCALE) image = cv2.resize(image, (128, 128), interpolation=cv2.INTER_LINEAR) epsilon = 0.3 k = 50 image_back = add_noise(image, epsilon, k) im = cv2.resize(image_back, (47, 62), interpolation=cv2.INTER_LINEAR) image_back = np.uint8(im) cv2.imwrite("face_privacy.jpg", image_back) return image_back
以下是将三个函数合并成一个函数的代码:
```python
def add_noisy_image(image_path, output_path, epsilon=0.3, k=50):
# 读取图片并调整大小
image = cv2.imread(image_path, cv2.IMREAD_GRAYSCALE)
image = cv2.resize(image, (128, 128), interpolation=cv2.INTER_LINEAR)
# 对图片添加噪声
f = np.fft.fft2(image)
fshift = np.fft.fftshift(f)
rows, cols = image.shape
FIM = fshift
FIM_k = FIM[:k, :k]
delta_f = np.max(np.sqrt(np.real(FIM[:k, :k]) ** 2 + np.imag(FIM[:k, :k]) ** 2)) - np.min(np.sqrt(np.real(FIM[:k, :k]) ** 2 + np.imag(FIM[:k, :k]) ** 2))
c = delta_f / epsilon
d = delta_f * math.sqrt(2 * math.log(1.2 / 0.1)) / epsilon
sensitivity = np.abs(FIM_k) / np.sqrt(epsilon)
sensitivity2 = np.abs(FIM) / np.sqrt(epsilon)
scale = sensitivity2 / epsilon
b = d * scale
p = 0.5
noise = np.random.laplace(scale=b, size=(rows, cols)) + np.mean(f) * p
image_noise = fshift + noise
f_ishift = np.fft.ifftshift(image_noise)
image_back = np.fft.ifft2(f_ishift)
image_back = np.real(image_back)
# 调整大小并保存图片
im = cv2.resize(image_back, (47, 62), interpolation=cv2.INTER_LINEAR)
image_back = np.uint8(im)
cv2.imwrite(output_path, image_back)
return image_back
```
这个函数接收三个参数:输入图片路径、输出图片路径、epsilon 和 k。其中,epsilon 和 k 用于调整噪声的强度和频率范围。在函数内部,首先读取输入图片并调整大小,然后对图片进行处理生成噪声图像。最后,将生成的噪声图像调整大小并保存到指定路径,返回生成的噪声图像。
使用这个函数时,可以像下面这样调用:
```python
add_noisy_image('image.jpg', 'face_privacy.jpg', epsilon=0.3, k=50)
```
def add_noise(image, epsilon, k): # 添加拉普拉斯噪声 # 进行离散傅里叶变换 f = np.fft.fft2(image) # 将零频率分量移到频谱中心 fshift = np.fft.fftshift(f) rows, cols = image.shape b = laplas(fshift, epsilon, k) # print(b) p = 0.5 noise = np.random.laplace(scale=b, size=(rows, cols)) + np.mean(f) * p # noise = np.random.laplace(0, 1/b, (rows, cols)) image_noise = fshift + noise f_ishift = np.fft.ifftshift(image_noise) # 进行逆离散傅里叶变换 image_back = np.fft.ifft2(f_ishift) image_back = np.real(image_back) return image_back def laplas(FIM, epsilon, k): FIM_k = FIM[:k, :k] # 给定隐私预算 epsilon # 计算给定隐私预算时的拉普拉斯机制的参数的最小值 # 计算每个系数的灵敏度 sensitivity = np.abs(FIM_k) / np.sqrt(epsilon) sensitivity2 = np.abs(FIM) / np.sqrt(epsilon) scale = sensitivity2 / epsilon # 计算拉普拉斯机制的参数 # 计算前 k×k 个 DFT 系数的最大值和最小值之差 delta_f = np.max(np.sqrt(np.real(FIM[:k, :k]) ** 2 + np.imag(FIM[:k, :k]) ** 2)) - np.min( np.sqrt(np.real(FIM[:k, :k]) ** 2 + np.imag(FIM[:k, :k]) ** 2)) # 计算拉普拉斯噪声的尺度参数 c = delta_f / epsilon d = delta_f * math.sqrt(2 * math.log(1.25 / 0.1)) / epsilon # a = np.min(sensitivity) / (epsilon * k**2) return d def add_noisy_image(): # 读取人脸图像 image = cv2.imread("image.jpg", cv2.IMREAD_GRAYSCALE) image = cv2.resize(image, (128, 128), interpolation=cv2.INTER_LINEAR) # 进行离散傅里叶变换 epsilon = 0.3 k = 50 image_back = add_noise(image, epsilon, k) im = cv2.resize(image_back, (47, 62), interpolation=cv2.INTER_LINEAR) # 将图像转换为整型并保存 image_back = np.uint8(im) cv2.imwrite("face_privacy.jpg", image_back) return image_back
将三个函数合并成一个函数如下所示:
```python
import numpy as np
import cv2
import math
def add_noisy_image(image_path, epsilon, k):
def laplas(FIM, epsilon, k):
FIM_k = FIM[:k, :k]
sensitivity = np.abs(FIM_k) / np.sqrt(epsilon)
sensitivity2 = np.abs(FIM) / np.sqrt(epsilon)
scale = sensitivity2 / epsilon
delta_f = np.max(np.sqrt(np.real(FIM[:k, :k]) ** 2 + np.imag(FIM[:k, :k]) ** 2)) - np.min(
np.sqrt(np.real(FIM[:k, :k]) ** 2 + np.imag(FIM[:k, :k]) ** 2))
c = delta_f / epsilon
d = delta_f * math.sqrt(2 * math.log(1.25 / 0.1)) / epsilon
return d
def add_noise(image, epsilon, k):
f = np.fft.fft2(image)
fshift = np.fft.fftshift(f)
rows, cols = image.shape
b = laplas(fshift, epsilon, k)
p = 0.5
noise = np.random.laplace(scale=b, size=(rows, cols)) + np.mean(f) * p
image_noise = fshift + noise
f_ishift = np.fft.ifftshift(image_noise)
image_back = np.fft.ifft2(f_ishift)
image_back = np.real(image_back)
return image_back
image = cv2.imread(image_path, cv2.IMREAD_GRAYSCALE)
image = cv2.resize(image, (128, 128), interpolation=cv2.INTER_LINEAR)
image_back = add_noise(image, epsilon, k)
im = cv2.resize(image_back, (47, 62), interpolation=cv2.INTER_LINEAR)
image_back = np.uint8(im)
cv2.imwrite("face_privacy.jpg", image_back)
return image_back
```
使用方式:
```python
image_back = add_noisy_image("image.jpg", 0.3, 50)
```
其中,第一个参数是原始图像的路径,第二个参数是隐私预算 epsilon,第三个参数是前 k 个 DFT 系数的个数。该函数返回添加拉普拉斯噪声后的图像。
阅读全文
相关推荐
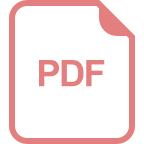
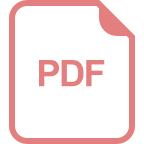
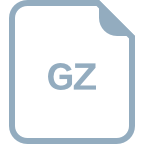













