yolov1 network structure
时间: 2023-11-09 07:26:06 浏览: 33
Yolov1 is an object detection network that uses a single neural network to detect objects in images. The network is composed of 24 convolutional layers followed by two fully connected layers. Here is the detailed network structure of Yolov1:
1. Input image of size 448 x 448.
2. Convolutional layer with 64 filters, 7x7 kernel size, 2x2 stride, and ReLU activation.
3. Max pooling layer with 2x2 window size and 2x2 stride.
4. Convolutional layer with 192 filters, 3x3 kernel size, 1x1 stride, and ReLU activation.
5. Max pooling layer with 2x2 window size and 2x2 stride.
6. Convolutional layer with 128 filters, 1x1 kernel size, 1x1 stride, and ReLU activation.
7. Convolutional layer with 256 filters, 3x3 kernel size, 1x1 stride, and ReLU activation.
8. Convolutional layer with 256 filters, 1x1 kernel size, 1x1 stride, and ReLU activation.
9. Convolutional layer with 512 filters, 3x3 kernel size, 1x1 stride, and ReLU activation.
10. Max pooling layer with 2x2 window size and 2x2 stride.
11. Convolutional layer with 256 filters, 1x1 kernel size, 1x1 stride, and ReLU activation.
12. Convolutional layer with 512 filters, 3x3 kernel size, 1x1 stride, and ReLU activation.
13. Convolutional layer with 256 filters, 1x1 kernel size, 1x1 stride, and ReLU activation.
14. Convolutional layer with 512 filters, 3x3 kernel size, 1x1 stride, and ReLU activation.
15. Convolutional layer with 256 filters, 1x1 kernel size, 1x1 stride, and ReLU activation.
16. Convolutional layer with 512 filters, 3x3 kernel size, 1x1 stride, and ReLU activation.
17. Convolutional layer with 256 filters, 1x1 kernel size, 1x1 stride, and ReLU activation.
18. Convolutional layer with 512 filters, 3x3 kernel size, 1x1 stride, and ReLU activation.
19. Convolutional layer with 512 filters, 1x1 kernel size, 1x1 stride, and ReLU activation.
20. Convolutional layer with 1024 filters, 3x3 kernel size, 1x1 stride, and ReLU activation.
21. Max pooling layer with 2x2 window size and 2x2 stride.
22. Convolutional layer with 512 filters, 1x1 kernel size, 1x1 stride, and ReLU activation.
23. Convolutional layer with 1024 filters, 3x3 kernel size, 1x1 stride, and ReLU activation.
24. Convolutional layer with 512 filters, 1x1 kernel size, 1x1 stride, and ReLU activation.
25. Convolutional layer with 1024 filters, 3x3 kernel size, 1x1 stride, and ReLU activation.
26. Convolutional layer with 1024 filters, 3x3 kernel size, 1x1 stride, and ReLU activation.
27. Convolutional layer with 1024 filters, 3x3 kernel size, 2x2 stride, and ReLU activation.
28. Flatten layer.
29. Fully connected layer with 4096 neurons and ReLU activation.
30. Dropout layer with 0.5 probability.
31. Fully connected layer with 1470 neurons.
The output of the network is a vector of length 1470, which is then reshaped to a 7x7x30 tensor representing the predicted bounding boxes and their associated class probabilities.
相关推荐
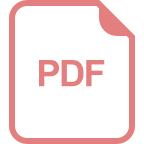














