Full Analysis of fmincon Solution: Iteration, Convergence, and Termination Criteria Elucidated
发布时间: 2024-09-14 11:31:20 阅读量: 32 订阅数: 27 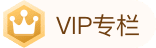
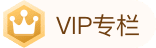
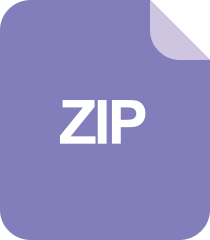
SAE-fmincon:SAE系统设计问题
# 1. Introduction to the fmincon Solver
fmincon is a solver in MATLAB designed for solving nonlinear constrained optimization problems. It employs the interior-point algorithm, which finds the minimum value of the objective function through an iterative process while satisfying the constraints. The fmincon solver is widely used in various optimization problems, including engineering design, financial modeling, and machine learning.
The fmincon solver has the following features:
- **Constraint Handling Capability:** fmincon can handle a variety of constraint conditions, including linear constraints, nonlinear constraints, and boundary constraints.
- **Efficient Algorithm:** The interior-point algorithm is generally more efficient than other optimization algorithms, especially for large-scale problems.
- **User-Friendly Interface:** fmincon is provided through MATLAB function interfaces, making it easy to use and configure.
# 2. Theoretical Basis of the fmincon Solving Process
### 2.1 Mathematical Model of Optimization Problems
Optimization problems can typically be represented by the following mathematical model:
```
min f(x)
subject to:
c(x) <= 0
c_eq(x) = 0
```
Where:
- f(x) is the objective function, representing the function that needs to be minimized.
- x is the decision variable, a vector of n dimensions.
- c(x) are the inequality constraints, representing the inequalities that x must satisfy.
- c_eq(x) are the equality constraints, representing the equalities that x must satisfy.
### 2.2 Principle of the fmincon Solving Algorithm
The fmincon solver adopts the **Sequential Quadratic Programming (SQP)** method to solve optimization problems. The SQP method is an iterative algorithm that starts from a feasible point (a point that satisfies all constraints) and moves along a feasible direction (a direction that does not violate any constraints) until a local optimal solution is found.
The specific steps of the fmincon solving algorithm are as follows:
1. Initialization: Set the current point x to a feasible point, and calculate the objective function value f(x) as well as the constraint condition values c(x) and c_eq(x).
2. Determine the feasible direction: Calculate the constraint condition gradients ∇c(x) and ∇c_eq(x), and determine a feasible direction d such that:
```
∇c(x)^T d <= 0
∇c_eq(x)^T d = 0
```
3. Linear search: Perform a linear search along the feasible direction d to find a step size α that minimizes the objective function value f(x + αd).
4. Update: Update the current point to x + αd, and recalculate the objective function value and constraint condition values.
5. Repeat steps 2-4 until the termination conditions are satisfied.
### 2.3 Constraint Handling Mechanism
The fmincon solver provides various constraint handling mechanisms, including:
***Interior-Point Method:** Converts constraint conditions into penalty functions and adds them to the objective function.
***Exterior-Point Method:** Converts constraint conditions into barrier functions and adds them to the objective function.
***Penalty Function Method:** Converts the degree of violation of constraint conditions into penalty functions and adds them to the objective function.
***Lagrange Multiplier Method:** Introduces Lagrange multipliers, converts constraint conditions into equalities, and adds them to the objective function.
Different constraint handling mechanisms are suitable for different optimization problems. The fmincon solver will automatically select the most appropriate constraint handling mechanism based on the characteristics of the optimization problem.
# 3.1 fmincon Solver Parameter Settings
The fmincon solver offers a rich set of parameter setting options, allowing users to control the behavior and performance of the solving process. The main parameter settings include:
- **Algorithm:** Specifies the solving algorithm, with options including:
- `interior-point`: Interior-point method, suitable for large-scale linear programming problems.
- `sqp`: Sequential Quadratic Programming method, suitable for general nonlinear optimization problems.
- `active-set`: Active-set method, suitable for bounded constraint problems.
- **Display:** Specifies the display level during the solving process, with options including:
- `off`: No information is displayed.
- `iter`: Information is displayed for each iteration.
- `final`: Only information at the end of the solving process is displayed.
- `notify`: Information is displayed during significant events in the solving process.
- **MaxFunEvals:** Specifies the maximum number of function evaluations allowed during the solving process.
- **MaxIter:** Specifies the maximum number of iterations allowed during the solving process.
- **TolFun:** Specifies the tolerance for changes in the objective function value, when the change in the function value is less than this tolerance, the solver considers the function value to have converged.
- **TolX:** Specifies the tolerance for changes in the independent variables, when the change in independent variables is less than this tolerance, the solver considers the independent variables to have converged.
- **TolCon:** Specifies the tolerance for the degree of violation of constraint conditions, when the degree of violation is less than this tolerance, the solver considers the constraint conditions to be satisfied.
### 3.2 Methods for Handling Common Constraints
The fmincon solver supports handling various types of constraints, including:
- **Linear Constraints:** Constraints in the form of `A*x <= b` or `A*x >= b`.
- **Nonlinear Constraints:** Constraints in the form of `c(x) <= 0` or `c(x) >= 0`.
- **Equality Constraints:** Constraints in the form of `c(x) = 0`.
For different types of constraint conditions, the fmincon solver provides different handling methods:
- **Linear Constraints:** Solved using the interior-point method or the active-set method.
- **Nonlinear Constraints:** Solved using the Sequential Quadratic Programming method or the interior-point method.
- **Equality Constraints:** Solved using the Lagrange multiplier method or the penalty function method.
### 3.3 Monitoring and Debugging the Solving Process
During the solving process, users can monitor the progress and status of the solver and debug the solving process. The main methods include:
- **Using the Display parameter:** Setting the Display parameter to `iter` or `notify` can display information during the solving process, including the number of iterations, objective function value, independent variable values, and the degree of violation of constraint conditions.
- **Using the OutputFcn function:** Specify the OutputFcn function to execute it after each iteration and obtain the current status information of the solver.
- **Using the fmincon debugging mode:** Entering `fmincon('debug')` in the MATLAB command window can enter the fmincon debugging mode and perform step-by-step debugging of the solving process.
# 4. Convergence Analysis of the fmincon Solving Process
### 4.1 Definition and Determination of Convergence Conditions
The convergence of the fmincon solving process refers to the ability of the solver to find a solution that satisfies the convergence conditions within a finite number of iterations. Convergence conditions are usually defined as:
```
||x_k - x_{k-1}|| < ε
```
Where:
* x_k: The solution at the current iteration point
* x_{k-1}: The solution at the previous iteration point
* ε: Convergence tolerance, a very small positive number
When the convergence condition is met, it indicates that the solver has found a solution that satisfies the required precision.
### 4.2 Factors Affecting Convergence
The factors that affect the convergence of the fmincon solving process mainly include:
***Nature of the objective function:** The continuity, smoothness, convexity, and other properties of the objective function will affect the convergence speed and stability of the solver.
***Nature of constraint conditions:** The linearity, nonlinearity, equality constraints, inequality constraints, and other properties of the constraint conditions also affect the solver's convergence.
***Solver parameter settings:** Solver parameters, such as the maximum number of iterations and step-size factors, also affect convergence.
***Selection of the initial point:** The closer the initial point is to the optimal solution, the faster the convergence speed.
### 4.3 Common Reasons for Convergence Failure
The fmincon solving process may fail to converge due to the following reasons:
***Objective function or constraints do not meet continuity, smoothness, and other requirements:** In such cases, the solver may have difficulty finding a feasible solution.
***Conflicting constraints:** If the constraints are contradictory, the solver cannot find a feasible solution.
***Improper solver parameter settings:** If solver parameters are set improperly, such as too small a maximum number of iterations or too large a step-size factor, the solver may not find a convergent solution within a limited number of iterations.
***Improper selection of the initial point:** If the initial point is far from the optimal solution, the solver may require more iterations to converge.
***Limited computational precision:** Due to the limited computational precision of computers, the solver may not be able to find a solution that fully satisfies the convergence conditions.
# 5. Termination Conditions of the fmincon Solving Process
### 5.1 Setting and Selection of Termination Conditions
The fmincon solver offers various termination conditions, ***mon termination conditions include:
- **Maximum iteration number:** Specifies that the solver will terminate after reaching the specified maximum number of iterations.
- **Objective function value change threshold:** Specifies that the solver will terminate after the change in the objective function value is less than the specified threshold in consecutive iterations.
- **Gradient norm threshold:** Specifies that the solver will terminate after the norm of the objective function gradient is less than the specified threshold in consecutive iterations.
- **Step size threshold:** Specifies that the solver will terminate after the step size is less than the specified threshold in consecutive iterations.
- **Constraint violation threshold:** Specifies that the solver will terminate after the degree of constraint violation is less than the specified threshold in consecutive iterations.
When selecting termination conditions, the following factors need to be considered:
- **Problem complexity:** Complex problems may require more iterations to converge, so a larger maximum iteration number should be set.
- **Nature of the objective function:** If the objective function has multiple local minima, a smaller objective function value change threshold should be set to avoid falling into local minima.
- **Type of constraint conditions:** If the constraint conditions are very strict, a smaller constraint violation threshold should be set to ensure that the constraint conditions are satisfied.
### 5.2 Impact of Termination Conditions on Solving Results
Termination conditions have a significant impact on solving results:
- **Premature termination:** If the termination conditions are set too宽松, the solver may terminate before the objective function has sufficiently converged, resulting in inaccurate solving results.
- **Late termination:** If the termination conditions are set too strictly, the solver may continue iterating even after the objective function has converged, wasting computational resources.
Therefore, selecting appropriate termination conditions is crucial, as it can ensure the accuracy of solving results while avoiding unnecessary computational overhead.
### 5.3 Optimization Strategies for Termination Conditions
To optimize termination conditions, the following strategies can be adopted:
- **Use multiple termination conditions:** Using multiple termination conditions can improve the robustness of solving. For example, both the maximum iteration number and the objective function value change threshold can be set.
- **Dynamically adjust termination conditions:** Dynamically adjust termination conditions based on the situation during the solving process. For example, if the convergence speed of the objective function is slow, the maximum iteration number can be appropriately increased.
- **Use adaptive termination conditions:** Use adaptive termination conditions that automatically adjust based on information during the solving process. For example, the fmincon solver provides adaptive objective function value change thresholds that can be automatically adjusted based on the convergence of the objective function.
By optimizing termination conditions, the efficiency and accuracy of the fmincon solver can be significantly improved, yielding better solving results.
# 6. Performance Optimization of the fmincon Solving Process
### 6.1 Evaluation Indicators of Solving Efficiency
The evaluation indicators for solving efficiency mainly include the following aspects:
- **Solving time:** The time the solver takes to find a solution that satisfies the termination conditions.
- **Iteration count:** The number of iterations executed by the solver.
- **Objective function value:** The objective function value of the solution found by the solver.
- **Constraint violation degree:** The degree to which the solution found by the solver violates the constraint conditions.
### 6.2 Factors Affecting Solving Efficiency
The factors that affect the efficiency of the fmincon solving process mainly include:
- **Problem size:** The number of problem variables and the number of constraint conditions.
- **Complexity of the objective function and constraint conditions:** The degree of nonlinearity and differentiability of the objective function and constraint conditions.
- **Solver parameter settings:** Solver parameter settings, such as the maximum number of iterations and tolerances.
- **Quality of the initial solution:** The quality of the initial solution has a significant impact on solving efficiency.
### 6.3 Methods for Improving Solving Efficiency
Methods to improve the efficiency of fmincon solving include:
- **Select appropriate solver parameters:** Choose appropriate solver parameters based on the characteristics of the problem, such as the maximum number of iterations and tolerances.
- **Provide a high-quality initial solution:** Providing an initial solution close to the optimal solution can significantly improve solving efficiency.
- **Simplify the objective function and constraints:** If possible, simplify the objective function and constraints to reduce the complexity of the problem.
- **Use parallel computing:** For large-scale problems, parallel computing can be used to improve solving efficiency.
- **Optimize the code:** Optimize the code involved in the solving process to reduce computation time.
0
0
相关推荐
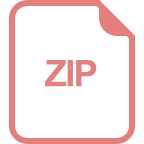
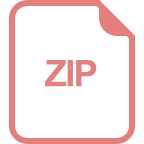
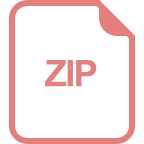
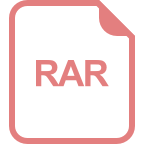
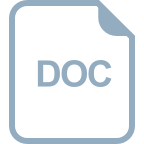
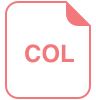