fmincon Convergence Slowdown Troubleshooting Guide: Identifying and Resolving Convergence Issues
发布时间: 2024-09-14 11:34:10 阅读量: 37 订阅数: 27 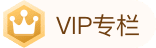
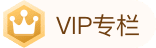
# 1. Overview of the fmincon Convergence Algorithm
fmincon is a function in MATLAB designed to solve nonlinear constrained optimization problems. It employs the Sequential Quadratic Programming (SQP) algorithm, which transforms the problem into a series of quadratic programming sub-problems and iteratively solves them. The convergence speed of the SQP algorithm depends on the complexity of the objective function, the extent of the constraints, and the choice of initial points.
# 2. Potential Causes of Slow Convergence
The issue of fmincon convergence may stem from various factors, including:
### 2.1 Complexity of the Objective Function
The complexity of the objective function significantly affects the convergence speed. High-dimensional, non-convex, or non-smooth objective functions tend to result in slow convergence, as the optimization algorithm struggles to find the global optimum.
**Strategies:**
* Consider simplifying the objective function, such as through linearization or approximation.
* Try using optimization algorithms designed for complex objective functions, like genetic algorithms or particle swarm optimization.
### 2.2 Improper Selection of Starting Points
The choice of starting points is crucial for convergence speed. If the starting point is far from the optimal solution, the optimization algorithm may require many iterations to converge.
**Strategies:**
* Try different starting points, such as random points or points selected based on prior knowledge about the problem.
* Consider using a warm start, which begins from a previously optimized solution.
### 2.3 Restrictive Constraints
Constraints can limit the search space of the optimization algorithm, leading to slow convergence, especially when they are tight or nonlinear.
**Strategies:**
* Loosen or adjust the constraints to provide a broader search space.
* Consider algorithms specifically designed for constrained optimization problems, like interior-point methods or penalty methods.
### 2.4 Numerical Precision Issues
Limited numerical precision can cause the optimization algorithm to get stuck in local optima or experience slow convergence.
**Strategies:**
* Use higher numerical precision, such as double-precision floating-point numbers.
* Consider using algorithms with higher numerical stability.
#### Code Example
The following code block demonstrates the impact of the complexity of the objective function on convergence speed:
```python
import numpy as np
from scipy.optimize import fmin_l_bfgs_b
# Define a high-dimensional objective function
def objective_high_dim(x):
return np.sum(x**2) + np.sum(np.sin(x))
# Define a low-dimensional objective function
def objective_low_dim(x):
return x**2 + np.sin(x)
# Set starting points
x0_high_dim = np.random.rand(100)
x0_low_dim = 0.5
# Optimize the high-dimensional objective function
res_high_dim = fmin_l_bfgs_b(objective_high_dim, x0_high_dim)
# Optimize the low-dimensional objective function
res_low_dim = fmin_l_bfgs_b(objective_low_dim, x0_low_dim)
# Print the number of iterations
print("High-dimensional objective function iterations:", res_high_dim.nit)
print("Low-dimensional objective function iterations:", res_low_dim.nit)
```
**Logical Analysis:**
This code block compares the convergence speed of high-dimensional and low-dimensional objective functions. The high-dimensional objective function requires more iterations to converge, indicating that the complexity of the objective function affects convergence speed.
#### Table Example
The following table summarizes potential causes of slow convergence and their corresponding strategies:
| Cause | Strategies |
|---|---|
| Complexity of Objective Function | Simplify Objective Function, Use Specialized Algorithms |
| Improper Selection of Starting Points | Use Different Starting Points, Warm Start |
| Restrictive Constraints | Loosen Constraints, Use Constraint Optimization Algorithms |
| Numerical Precision Issues | Use Higher Precision, Numerically Stable Algorithms |
#### Mermaid Flowchart Example
The following mermaid flowchart illustrates the troubleshooting process for slow convergence:
```mermaid
graph LR
subgraph Slow Convergence
start(Slow Convergence) --> ObjectiveFunctionComplexity --> SimplifyObjectiveFunction
start(Slow Convergence) --> ImproperStartingPointSelection --> UseDifferentStartingPoints
start(Slow Convergence) --> RestrictiveConstraints --> LoosenConstraints
start(Slow Convergence) --> NumericalPrecisionIssues --> UseHigherPrecision
end
```
# 3. Methods for Identifying Slow Convergence
### 3.1 Monitoring the Iterative Process
Monitoring the iterative process is a vital means of identifying slow convergence. Observing changes in key indicators during iterations can help timely detect convergence anomalies.
#### Key Indicator Monitoring
Key indicators include:
- **Objective Function Value:** The trend of changes in the objective function value reflects the progress of the optimization algorithm.
- **Gradient Norm:** The gradient norm measures the extent of change in the objective function at the current point, and its reduction indicates that the algorithm is approaching the extremum.
- **Step Size:** The step size represents the distance moved by the algorithm in each iteration, and its reduction indicates convergence.
- **Constraint Violation Degree:** For constrained optimization problems, the constraint violation degree reflects the algorithm's degree of satisfaction with the constraints.
#### Monitoring Methods
Key indicators can be monitored by the following methods:
- **Logging:** Record key indicators into log files for subsequent analys
0
0
相关推荐
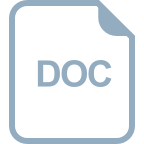
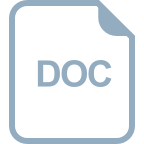
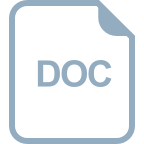
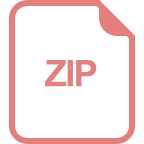
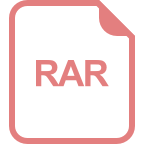
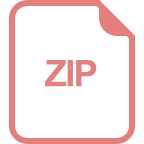