The Application of fmincon in Machine Learning: Optimizing Model Parameters and Hyperparameters
发布时间: 2024-09-14 11:51:04 阅读量: 14 订阅数: 20 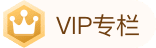
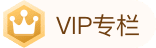
# 1. Introduction to fmincon**
fmincon is a powerful optimization function in MATLAB used to solve nonlinear constrained optimization problems. It employs the Sequential Quadratic Programming (SQP) algorithm, an iterative method that solves a quadratic sub-problem in each iteration. fmincon can handle constrained optimization problems with both continuous and discrete variables.
The general form of fmincon is as follows:
```
[x, fval, exitflag, output] = fmincon(fun, x0, A, b, Aeq, beq, lb, ub, nonlcon, options)
```
Where:
* `fun`: The objective function, which takes a vector `x` as input and returns a scalar value.
* `x0`: The initial guess solution.
* `A` and `b`: The coefficient matrix and right-hand side vector for linear inequality constraints.
* `Aeq` and `beq`: The coefficient matrix and right-hand side vector for linear equality constraints.
* `lb` and `ub`: The lower and upper bounds for the variables.
* `nonlcon`: The nonlinear constraint function that takes a vector `x` as input and returns a structure containing the values of the nonlinear constraints and the Jacobian matrix.
* `options`: Optimization options to control the algorithm's behavior.
# 2. The Application of fmincon in Machine Learning**
fmincon is a robust optimization algorithm with widespread applications in machine learning. It can be used to optimize model parameters and hyperparameters to enhance model performance.
**2.1 Model Parameter Optimization**
Model parameter optimization refers to adjusting the tunable parameters within a model to minimize the loss function or objective function. fmincon can be used to optimize the parameters of various machine learning models, including:
**2.1.1 Linear Regression**
Linear regression is a simple machine learning algorithm used for predicting continuous values. fmincon can be employed to optimize the weights and intercept parameters of a linear regression model to minimize the sum of squared error loss function.
**Code Block:**
```python
import numpy as np
from scipy.optimize import fmin_l_bfgs_b
def linear_regression(X, y):
"""
Optimize the linear regression model using fmin_l_bfgs_b.
Parameters:
X: Feature matrix
y: Target variable
Returns:
Optimal weights and intercept
"""
# Define loss function
def loss_function(params):
w, b = params
return np.mean((np.dot(X, w) + b - y) ** 2)
# Initial parameters
initial_params = np.zeros(X.shape[1] + 1)
# Optimize parameters
params, _, _ = fmin_l_bfgs_b(loss_function, initial_params)
# Return optimal parameters
return params
```
**Logical Analysis:**
* The `loss_function` defines the squared error loss function, calculating the mean squared difference between predicted and actual values.
* The `fmin_l_bfgs_b` function uses the L-BFGS algorithm to optimize the loss function and returns the optimal parameters.
* The `params` contain the optimal weights and intercept, which can be used to predict new data.
**2.1.2 Logistic Regression**
Logistic regression is a machine learning algorithm for binary classification. fmincon can optimize the weights and intercept parameters of a logistic regression model to minimize the log-likelihood loss function.
**Code Block:**
```python
import numpy as np
from scipy.optimize import fmin_l_bfgs_b
def logistic_regression(X, y):
"""
Optimize the logistic regression model using fmin_l_bfgs_b.
Parameters:
X: Feature matrix
y: Target variable (binary classification)
Returns:
Optimal weights and intercept
"""
# Define loss function
def loss_function(params):
w, b = params
return np.mean(-y * np.log(sigmoid(np.dot(X, w) + b)) - (1 - y) * np.log(1 - sigmoid(np.dot(X, w) + b)))
# Define sigmoid function
def sigmoid(x):
return 1 / (1 + np.exp(-x))
# Initial parameters
initial_params = np.zeros(X.shape[1] + 1)
# Optimize parameters
params, _, _ = fmin_l_bfgs_b(loss_function, initial_params)
# Return optimal parameters
return params
```
**Logical Analysis:**
* The `loss_function` defines the log-likelihood loss function, calculating the mean cross-entropy between predicted probabilities and true labels.
* The `sigmoid` function converts
0
0
相关推荐
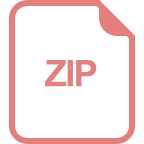
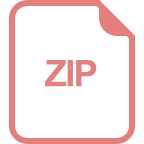
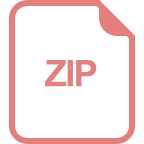
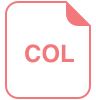
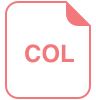
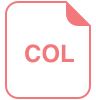
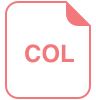
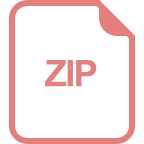
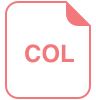