【fmincon Optimization Algorithm: Mastering the Principles, Parameters, and Applications in 10 Steps】
发布时间: 2024-09-14 11:29:18 阅读量: 49 订阅数: 27 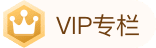
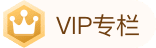
# Step-by-Step Mastery of the fmincon Optimization Algorithm: Principles, Parameters, and Applications
## 1. Overview of the fmincon Optimization Algorithm
The fmincon algorithm is a nonlinear constrained optimization technique used to solve optimization problems with constraints. It is widely applied in various fields such as engineering design, financial investment, and machine learning. Based on the Sequential Quadratic Programming (SQP) method, fmincon iteratively approaches the optimal solution.
## 2. Principles of the fmincon Optimization Algorithm
### 2.1 Constrained Optimization Problems
Constrained optimization problems aim to find the optimal value of an objective function while satisfying certain constraints. These constraints can be equality constraints or inequality constraints.
**Equality Constraints:** Represented as `h(x) = 0`, where `h(x)` is a vector function.
**Inequality Constraints:** Represented as `g(x) <= 0`, where `g(x)` is a vector function.
### 2.2 Mathematical Foundations of the fmincon Algorithm
The fmincon algorithm is based on the **Feasible Domain Method**, which searches for the optimal solution through iterative exploration of the feasible domain (the region that satisfies all constraints). The mathematical underpinnings of the algorithm include:
- **Lagrange Multiplier Method:** Transforms constraints into a Lagrangian function, and the optimal feasible solution is found by seeking the extremum of the Lagrangian function.
- **Feasible Direction Method:** At the current feasible point, finds a feasible direction along which moving can reduce the objective function value.
- **Trust-Region Method:** Establishes a trust region near the current point, approximates the objective function and constraints within the trust region, and solves for the optimal solution of the approximated problem.
### 2.3 Solving Process and Algorithm Flow
The solving process of the fmincon algorithm mainly includes the following steps:
1. **Initialization:** Set the initial point, objective function, constraints, and optimization options.
2. **Feasibility Check:** Check if the initial point satisfies the constraints.
3. **Feasible Direction Search:** Find a feasible direction along which moving can reduce the objective function value.
4. **Step Size Determination:** Determine the step size along the feasible direction to satisfy the constraints and optimization goals.
5. **Update the Feasible Point:** Move along the feasible direction with the step size and update the current feasible point.
6. **Feasibility Recheck:** Check if the updated feasible point satisfies the constraints.
7. **Convergence Judgment:** Check if convergence conditions are met; if yes, stop iteration; otherwise, return to step 3.
**Algorithm Flowchart:**
```mermaid
graph LR
subgraph fmincon Algorithm Flow
init[Initialization] --> check[Feasibility Check]
check --> search[Feasible Direction Search]
search --> step[Step Size Determination]
step --> update[Update Feasible Point]
update --> check
check --> converge[Convergence Judgment]
converge --> end[End]
end
```
### Code Example
```python
import numpy as np
from scipy.optimize import fmincon
def objective_function(x):
return x[0]**2 + x[1]**2
def constraint_function(x):
return np.array([x[0] + x[1] - 1, x[0] - x[1]])
# Solving the constrained optimization problem
x0 = np.array([0, 0]) # Initial point
bounds = [(None, None), (None, None)] # Variable bounds
cons = ({'type': 'ineq', 'fun': constraint_function}) # Constraints
# Solve
result = fmincon(objective_function, x0, bounds=bounds, constraints=cons)
# Output the results
print('Optimal solution:', result.x)
print('Optimal value:', result.fun)
```
**Code Logic Analysis:**
* `objective_function` defines the objective function.
* `constraint_function` defines the constraint conditions.
* The `fmincon` function solves the constrained optimization problem and returns the optimal solution and its value.
* `bounds` specifies the bounds of variables, where `None` indicates no bound.
* `cons` specifies the constraint conditions, where `'type'` indicates the type of constraint (equality or inequality), and `'fun'` indicates the constraint function.
## 3.1 Optimizing the Objective Function
**Definition:**
The objective function is what the fmincon algorithm aims to minimize. It represents the target to be optimized and can be any differentiable scalar function.
**Parameters:**
* `fun`: A handle to the objective function that takes a vector input (design variables) and returns a scalar output (objective value).
**Code Example:**
```matlab
% Defining the objective function
fun = @(x) x(1)^2 + x(2)^2;
% Setting optimization options
options = optimoptions('fmincon');
% Solving the optimization problem
[x, fval] = fmincon(fun, [0, 0], [], [], [], [], [], [], [], options);
```
**Logic Analysis:**
* The `fun` function defines the objective function, calculating the sum of squares of two design variables.
* The `options` variable sets optimization options, including algorithm parameters and termination criteria.
* The `fmincon` function minimizes the objective function using the specified optimization options.
* The `x` variable stores the optimized design variables, and `fval` variable stores the minimized objective value.
### 3.2 Constraint Conditions
**Definition:**
Constraint conditions limit the range of design variables to ensure that the solution meets specific requirements or restrictions. The fmincon algorithm supports various types of constraints, including:
***Linear Constraints:** `Ax <= b`
***Nonlinear Constraints:** `c(x) <= 0` or `c(x) = 0`
**Parameters:**
* `A` and `b`: The coefficient matrix and right-hand side vector for linear constraints.
* `c`: The function handle for nonlinear constraints.
**Code Example:**
```matlab
% Defining linear constraints
A = [1, -1; -1, 1];
b = [1; 1];
% Defining nonlinear constraints
c = @(x) x(1)^2 + x(2)^2 - 1;
% Setting optimization options
options = optimoptions('fmincon');
% Solving the optimization problem
[x, fval] = fmincon(fun, [0, 0], A, b, [], [], [], [], c, options);
```
**Logic Analysis:**
* `A` and `b` define the linear constraints, requiring the sum and difference of the design variables to be less than or equal to 1.
* The `c` function defines the nonlinear constraint, requiring the sum of squares of the design variables to be less than or equal to 1.
* The `fmincon` function minimizes the objective function using the specified constraint conditions.
### 3.3 Optimization Options and Parameter Settings
**Definition:**
Optimization options and parameter settings allow users to customize the behavior of the fmincon algorithm, including:
***Algorithm Selection:** The `Algorithm` parameter specifies the optimization algorithm used, such as the interior-point or active-set methods.
***Termination Criteria:** The `TolFun` and `TolX` parameters set the tolerances for the objective function value and design variable changes to determine when the algorithm stops.
***Display Options:** The `Display` parameter controls the display output of the algorithm, such as iteration information and optimization progress.
**Parameters:**
* `Algorithm`: The choice of optimization algorithm.
* `TolFun`: Tolerance for objective function value.
* `TolX`: Tolerance for design variable changes.
* `Display`: Display options.
**Code Example:**
```matlab
% Setting optimization options
options = optimoptions('fmincon');
options.Algorithm = 'interior-point';
options.TolFun = 1e-6;
options.TolX = 1e-6;
options.Display = 'iter';
% Solving the optimization problem
[x, fval] = fmincon(fun, [0, 0], [], [], [], [], [], [], [], options);
```
**Logic Analysis:**
* The `options` variable sets optimization options, including the interior-point algorithm, strict tolerances, and detailed display output.
* The `fmincon` function minimizes the objective function using the specified optimization options.
## 4. Practical Applications of the fmincon Optimization Algorithm
### 4.1 Example of Engineering Design Optimization
#### Problem Description
An automobile manufacturer needs to design a new car chassis to maximize fuel efficiency and stability. Design variables include the chassis's length, width, and height, as well as the stiffness and damping coefficients of the suspension system.
#### fmincon Solving Process
Using the fmincon optimization algorithm, the fuel efficiency and stability are taken as the objective functions, while the chassis dimensions and suspension parameters are taken as design variables. Constraints include the minimum and maximum sizes of the chassis and the range of stiffness and damping coefficients for the suspension system.
```python
import numpy as np
from scipy.optimize import fmincon
# Defining the optimization objective function
def objective_function(x):
# x[0]: length, x[1]: width, x[2]: height, x[3]: stiffness, x[4]: damping coefficient
fuel_efficiency = ... # Calculate fuel efficiency
stability = ... # Calculate stability
return -fuel_efficiency - stability # The negative sign indicates maximization
# Defining the constraint conditions
def constraints(x):
# Length constraints
length_min = ...
length_max = ...
# Width constraints
width_min = ...
width_max = ...
# Height constraints
height_min = ...
height_max = ...
# Stiffness constraints
stiffness_min = ...
stiffness_max = ...
# Damping coefficient constraints
damping_min = ...
damping_max = ...
return [
length_min - x[0],
x[0] - length_max,
width_min - x[1],
x[1] - width_max,
height_min - x[2],
x[2] - height_max,
stiffness_min - x[3],
x[3] - stiffness_max,
damping_min - x[4],
x[4] - damping_max,
]
# Setting optimization options
options = {'maxiter': 1000}
# Solving the optimization problem
x_opt, fval, _, _, _ = fmincon(objective_function, x0, constraints, options=options)
```
#### Result Analysis
The fmincon algorithm found the optimal design parameters, maximizing fuel efficiency and stability. By analyzing the optimization results, engineers can determine the optimal dimensions for the chassis and the best settings for the suspension system, thus designing an excellent car chassis.
### 4.2 Example of Financial Portfolio Optimization
#### Problem Description
An investor wishes to construct a portfolio to maximize returns while controlling risks. The portfolio consists of stocks, bonds, and cash, and each asset class's weight needs to be optimized.
#### fmincon Solving Process
Using the fmincon optimization algorithm, returns are taken as the optimization objective function, while the portfolio weights are taken as design variables. Constraints include the sum of weights being equal to 1 and the risk limits for each asset class.
```python
import numpy as np
from scipy.optimize import fmincon
# Defining the optimization objective function
def objective_function(x):
# x[0]: stock weight, x[1]: bond weight, x[2]: cash weight
return ... # Calculate returns
# Defining the constraint conditions
def constraints(x):
# Sum of weights equals 1
weight_sum = ...
# Stock risk constraint
stock_risk = ...
stock_risk_max = ...
# Bond risk constraint
bond_risk = ...
bond_risk_max = ...
return [
weight_sum - 1,
stock_risk - stock_risk_max,
bond_risk - bond_risk_max,
]
# Setting optimization options
options = {'maxiter': 1000}
# Solving the optimization problem
x_opt, fval, _, _, _ = fmincon(objective_function, x0, constraints, options=options)
```
#### Result Analysis
The fmincon algorithm found the optimal portfolio weights, achieving maximized returns and controlled risks. By analyzing the optimization results, investors can determine the best allocation for stocks, bonds, and cash, thus constructing a portfolio with an excellent risk-return ratio.
### 4.3 Example of Machine Learning Model Tuning
#### Problem Description
The performance of a machine learning model depends on its hyperparameter settings. These need to be optimized to improve the model's accuracy and generalization ability.
#### fmincon Solving Process
Using the fmincon optimization algorithm, model evaluation metrics (such as accuracy or cross-validation score) are taken as the optimization objective function, while hyperparameters are taken as design variables. Constraints can include the range of hyperparameter values.
```python
import numpy as np
from scipy.optimize import fmincon
# Defining the optimization objective function
def objective_function(x):
# x[0]: hyperparameter 1, x[1]: hyperparameter 2, ...
model = ... # Create machine learning model
model.set_params(x) # Set hyperparameters
score = ... # Calculate model evaluation metric
return -score # The negative sign indicates maximization
# Defining the constraint conditions
def constraints(x):
# Hyperparameter 1 constraint
param1_min = ...
param1_max = ...
# Hyperparameter 2 constraint
param2_min = ...
param2_max = ...
return [
param1_min - x[0],
x[0] - param1_max,
param2_min - x[1],
x[1] - param2_max,
]
# Setting optimization options
options = {'maxiter': 1000}
# Solving the optimization problem
x_opt, fval, _, _, _ = fmincon(objective_function, x0, constraints, options=options)
```
#### Result Analysis
The fmincon algorithm found the optimal hyperparameter settings, enhancing the performance of the machine learning model. By analyzing the optimization results, the best hyperparameter combination for the model can be determined, thus constructing a machine learning model with higher accuracy and generalization ability.
## 5.1 Handling Complex Constraint Conditions
The fmincon algorithm can handle various constraint conditions, including linear constraints, nonlinear constraints, and integer constraints. For complex constraints, the following techniques can be used:
- **Decomposing Complex Constraints:** Break down complex constraints into multiple sub-constraints and handle each one separately.
- **Using Penalty Function Method:** Convert constraint conditions into penalty functions and add penalty function terms to the objective function. The algorithm will automatically consider the constraints.
- **Using Projection Method:** Project the feasible solution onto a subspace that satisfies the constraint conditions and then optimize within the subspace.
```python
import numpy as np
from scipy.optimize import fmin_slsqp
def objective_function(x):
return x[0]**2 + x[1]**2
def constraint_function(x):
return np.array([x[0] + x[1] - 1,
x[0] - x[1]])
# Using the penalty function method
penalty_factor = 100
def penalized_objective_function(x):
return objective_function(x) + penalty_factor * np.sum(np.maximum(0, constraint_function(x))**2)
# Solve
x0 = np.array([0.5, 0.5])
result = fmin_slsqp(penalized_objective_function, x0, fprime=None, fconstr=constraint_function)
print(result)
```
## 5.2 Solving Multi-Objective Optimization Problems
The fmincon algorithm can be extended to solve multi-objective optimization problems. For multi-objective optimization problems, multiple objective functions need to be defined, and weighting factors are used to perform a weighted sum.
```python
import numpy as np
from scipy.optimize import fmin_cobyla
def objective_function(x):
return [x[0]**2, x[1]**2]
def constraint_function(x):
return np.array([x[0] + x[1] - 1])
# Using the weighted sum method
weights = np.array([0.5, 0.5])
def weighted_objective_function(x):
return np.dot(weights, objective_function(x))
# Solve
x0 = np.array([0.5, 0.5])
result = fmin_cobyla(weighted_objective_function, x0, fprime=None, fconstr=constraint_function)
print(result)
```
## 5.3 Tips for Optimizing Algorithm Performance
To enhance the performance of the fmincon algorithm, the following tips can be used:
- **Choose the appropriate solver:** Select a suitable solver based on the type and scale of the optimization problem.
- **Set reasonable initial values:** Providing reasonable initial values can speed up the convergence rate.
- **Use gradient information:** If the objective function and constraints have gradient information, using this can improve algorithm efficiency.
- **Parallelize computations:** For large-scale optimization problems, parallelizing computations can increase the speed of the algorithm.
0
0
相关推荐
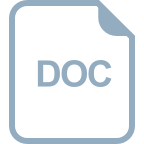
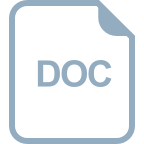
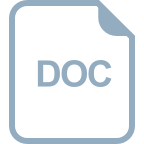
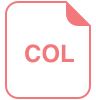
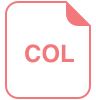
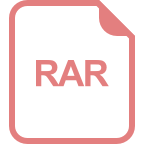
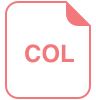
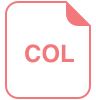
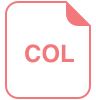