fmincon Nonlinear Constrained Optimization Tips: A Powerful Tool for Handling Complex Constraints
发布时间: 2024-09-14 11:37:59 阅读量: 31 订阅数: 33 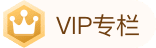
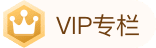
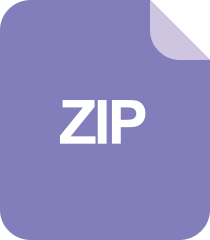
Algorithm-Nonlinear-Optimization-Algorithms.zip
# Introduction to fmincon for Nonlinear Constrained Optimization: A Powerful Tool for Handling Complex Constraints
fmincon is a robust function in MATLAB designed to tackle nonlinear constraint optimization problems. It empowers users to define objective functions, constraints, and optimization parameters to solve intricate optimization challenges. Utilizing a sequential quadratic programming algorithm, fmincon iteratively locates the minimum of the objective function within the constraints' boundaries.
Unlike unconstrained optimization, nonlinear constraint optimization involves conditions that must be satisfied. fmincon supports various constraint types, including linear, nonlinear, and boundary constraints. By specifying these constraints, users can confine the problem within a feasible solution space.
# 2. fmincon Optimization Algorithm and Parameter Settings
### 2.1 Overview of the Optimization Algorithm
fmincon is a powerful function in MATLAB for solving nonlinear constraint optimization problems. It employs an interior-point method, an iterative algorithm that approximates the optimal solution by solving a series of subproblems. The interior-point method handles constraints by iterating within the feasible domain, ensuring that the constraints are satisfied.
### 2.2 Constraint Handling Methods
fmincon supports various constraint types, including linear, nonlinear, and boundary constraints. For linear constraints, it uses linear programming techniques, while for nonlinear constraints, it adopts nonlinear programming techniques.
### 2.3 Tips for Parameter Configuration
The parameter configuration in fmincon is crucial for optimization performance. Key parameters include:
- **Algorithm:** Specifies the optimization algorithm, such as the interior-point method or sequential quadratic programming.
- **Display:** Controls the display level of the optimization process.
- **FunctionTolerance:** Tolerance for the optimization function value.
- **MaxFunEvals:** Maximum number of function evaluations.
- **MaxIter:** Maximum number of iterations.
It is recommended to start with default parameters and adjust them as needed to improve performance.
**Example Code:**
```matlab
% Define the optimization function
fun = @(x) x(1)^2 + x(2)^2;
% Define linear constraints
A = [1, 1; -1, 1];
b = [2; 1];
% Define boundary constraints
lb = [0; 0];
ub = [1; 1];
% Set optimization parameters
options = optimoptions('fmincon', 'Algorithm', 'interior-point', ...
'Display', 'iter', 'FunctionTolerance', 1e-6, 'MaxFunEvals', 1000, 'MaxIter', 100);
% Solve the optimization problem
[x, fval] = fmincon(fun, [0.5; 0.5], A, b, [], [], lb, ub, [], options);
% Print the optimization results
disp(['Optimal solution: ', num2str(x)]);
disp(['Optimal value: ', num2str(fval)]);
```
**Code Logic Analysis:**
* `fun` defines the optimization function, aiming to solve for the sum of squares of x1 and x2.
* `A` and `b` define the linear constraints, namely x1 + x2 ≤ 2 and -x1 + x2 ≤ 1.
* `lb` and `ub` define the boundary constraints, meaning x1 and x2 are within the range [0, 1].
* `options` set the optimization parameters, including the algorithm, display level, tolerance, and maximum number of iterations.
* `fmincon` solves the optimization problem, returning the optimal solution `x` and optimal value `fval`.
# 3.1 Linear Constraints
**Linear constraints** are the most common type of constraints in fmincon, taking the form:
```
A * x <= b
```
Where:
* A is an m x n matrix, with m representing the number of constraints and n the number of variables.
* x is an n x 1 vector of variables.
* b is an m x 1 vector of constants.
**Code Block:**
```matlab
% Define linear constraints
A = [1, 2; -1, 1];
b = [4; 2];
% Set optimization options
options = optimset('Algorithm', 'interior-point');
% Solve the optimization problem
[x, fval] = fmincon(@(x) x(1)^2 + x(2)^2, [0; 0], A, b, [], [], [], [], [], options);
```
**Logic Analysis:**
* `A` and `b` define two linear constraints: `x(1) + 2x(2) <= 4` and `-x(1) + x(2) <= 2`.
* The `optimset` function sets the optimization algorithm to the interior-point method.
* The `fmincon` function solves the optimization problem, where the objective function is `x(1)^2 + x(2)^2`.
### 3.2 Nonlinear Constraints
**Nonlinear constraints** are more complex, taking the form:
```
c(x) <= 0
```
Where:
* c(x) is a nonlinear function representing the constraint condition.
**Code Block:**
```matlab
% Define nonlinear constraints
c = @(x) x(1)^2 + x(2)^2 - 1;
% Set optimization options
options = optimset('Algorithm', 'sqp');
% Solve the optimization problem
[x, fval] = fmincon(@(x) x(1)^2 + x(2)^2, [0; 0], [], [], [], [], [], [], c, options);
```
**Logic Analysis:**
* The `c` function defines the nonlinear constraint: `x(1)^2 + x
0
0
相关推荐







