MATLAB Reading of Structured Data in TXT Files: Data Organization Experts, Efficient Management of Complex Structures
发布时间: 2024-09-13 21:29:14 阅读量: 26 订阅数: 30 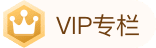
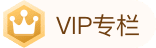
# Introduction to MATLAB Data Structures
MATLAB data structures are a special data type used to store and organize related data. They consist of a series of key-value pairs known as fields, where keys are strings, and values can be any MATLAB data type. Structures provide a convenient method for grouping related data and storing it in a way that is easy to manage and access.
Structures can be created using the `struct` function or by directly using dot or curly brace syntax. For example, the following code creates a structure named `student` with `name`, `age`, and `gpa` fields:
```matlab
student = struct('name', 'John Doe', 'age', 21, 'gpa', 3.8);
```
# Reading Structured Data from TXT Files in MATLAB
There are various methods for reading structured data from TXT files in MATLAB, each with its own advantages and disadvantages. This section will introduce the three most commonly used methods: the `textscan` function, the `importdata` function, and the `load` function.
### Data Organization and Formatting
Before reading structured data from a TXT file, it is essential to ensure that the data is organized and formatted correctly. The data in the TXT file should be organized in text format, where each line represents a structure, and each column represents a field. Fields should be separated by delimiters (such as commas or tabs).
For example, the following TXT file contains two structures with two fields each:
```
name,age
John,25
Mary,30
```
### Using the textscan Function to Read Data
The `textscan` function is a powerful tool for reading structured data. It allows users to specify the data format and use regular expressions to extract specific fields.
```matlab
% Open the TXT file
fid = fopen('data.txt', 'r');
% Read the file contents
data = textscan(fid, '%s %d', 'Delimiter', ',');
% Close the file
fclose(fid);
% Create a structure
names = data{1};
ages = data{2};
struct_data = struct('name', names, 'age', ages);
```
**Line-by-line code logic explanation:**
1. `fid = fopen('data.txt', 'r')`: Open the TXT file, specifying the filename as `data.txt`, and set the mode to read-only (`'r'`).
2. `data = textscan(fid, '%s %d', 'Delimiter', ',')`: Use the `textscan` function to read the file content. `%s %d` specifies the data format, where `%s` denotes a string and `%d` denotes an integer. `'Delimiter', ','` specifies the field delimiter as a comma.
3. `fclose(fid)`: Close the file.
4. `names = data{1}`: Extract the first output cell, which is the string field.
5. `ages = data{2}`: Extract the second output cell, which is the integer field.
6. `struct_data = struct('name', names, 'age', ages)`: Create a structure with field names `'name'` and `'age'`, and field values as `names` and `ages`, respectively.
### Using the importdata Function to Read Data
The `importdata` function is a simpler method to read structured data, as it automatically detects the data format and imports it as a structure.
```matlab
% Import the TXT file
data = importdata('data.txt', ',');
% Get field names
field_names = fieldnames(data);
% Create a structure
struct_data = struct();
for i = 1:length(field_names)
struct_data.(field_names{i}) = data.(field_names{i});
end
```
**Line-by-line code logic explanation:**
1. `data = importdata('data.txt', ',')`: Use the `importdata` function to import the TXT file, specifying the filename as `data.txt` and the delimiter as a comma.
2. `field_names = fieldnames(data)`: Get the field names of the structure.
3. `struct_data = struct()`: Create an empty structure.
4. `for i = 1:length(field_names)`: Iterate over the field names.
5. `struct_data.(field_names{i}) = data.(field_names{i})`: Add fields to the structure, with field names as `field_names{i}` and field values as `data.(field_names{i})`.
### Using the load Function to Read Data
The `load` function is a straightforward method for reading structured data, which loads the data from the TXT file into the workspace, where it can be assigned to a structure variable.
```matlab
% Load the TXT file
data = load('data.txt', '-ascii');
% Create a structure
struct_data = struct('name', data(:, 1), 'age', data(:, 2));
```
**Line-by-line code logic explanation:**
1. `data = load('data.txt', '-ascii')`: Use the `load` function to load the TXT file, with `'-ascii'` specifying the data format as ASCII text.
2. `struct_data = struct('name', data(:, 1), 'age', data(:, 2))`: Create a structure with field names `'name'` and `'age'`, and field values as `data(:, 1)` and `data(:, 2)`, respectively.
# Accessing and Modifying Structure Fields
Structure fields can be accessed
0
0
相关推荐








