Solving Differential Equations with ode45: Unveiling the 3 Secrets of Performance Optimization
发布时间: 2024-09-15 05:50:12 阅读量: 40 订阅数: 38 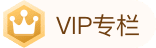
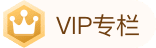
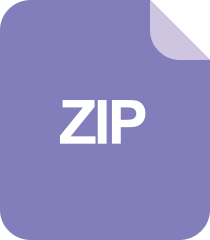
《COMSOL顺层钻孔瓦斯抽采实践案例分析与技术探讨》,COMSOL模拟技术在顺层钻孔瓦斯抽采案例中的应用研究与实践,comsol顺层钻孔瓦斯抽采案例 ,comsol;顺层钻孔;瓦斯抽采;案例,COM
# 1. Introduction to Solving Differential Equations with ode45
The ode45 solver is a powerful tool in MATLAB for solving ordinary differential equations (ODEs). It is based on the Runge-Kutta method, a widely used numerical method for solving ODEs. The ode45 solver employs an adaptive step size algorithm that can solve ODEs with minimal computational effort while ensuring accuracy.
One of the main advantages of the ode45 solver is its robustness. It can handle various types of ODEs, including stiff equations, nonlinear equations, and high-dimensional equations. Additionally, the ode45 solver provides fine control over the solving process, allowing users to specify the solution accuracy, step size, and output times.
# 2. Performance Optimization Techniques for Solving Differential Equations with ode45
In practical applications, performance optimization for solving differential equations with ode45 is crucial. This chapter will delve into the factors affecting the performance of ode45 and provide specific optimization tips to help improve your solving efficiency.
### 2.1 How the ode45 Solver Works
#### 2.1.1 The Principle of the Runge-Kutta Method
The ode45 solver uses the Runge-Kutta method to solve differential equations. The Runge-Kutta method is a single-step method that approximates the solution to the differential equation at the current time as a polynomial. By calculating the derivative of this polynomial, the solution at the next time can be obtained.
The accuracy of the Runge-Kutta method depends on the order used. The ode45 solver employs the fourth-order Runge-Kutta method, also known as RK4. The RK4 method has high accuracy, but also a larger computational cost.
#### 2.1.2 Implementation Details of the ode45 Solver
The ode45 solver is a built-in function in MATLAB, and its internal implementation details are as follows:
- **Adaptive Step Size Algorithm:** ode45 uses an adaptive step size algorithm to dynamically adjust the solution step size based on error estimates. The step size decreases when the error is large and increases when the error is small.
- **Local Error Estimation:** ode45 uses local error estimation to assess the solution accuracy. Local error estimation is obtained by calculating the difference between two solution results.
- **Convergence Criteria:** ode45 uses convergence criteria to determine if the solution has converged. The convergence criteria are based on local error estimation, and when the local error is less than a given tolerance, the solution is considered converged.
### 2.2 Factors Affecting the Performance of ode45
The main factors affecting the performance of ode45 include:
#### 2.2.1 Complexity of the Differential Equation
The complexity of the differential equation directly affects the solving efficiency of ode45. More complex differential equations, such as nonlinear or high-dimensional differential equations, require more computational effort.
#### 2.2.2 Solution Accuracy Requirements
Solution accuracy requirements also impact the performance of ode45. Higher accuracy requirements mean smaller tolerances, resulting in smaller solution steps and more computational effort.
#### 2.2.3 Solution Time Step
The solution time step is a key parameter for the ode45 adaptive step size algorithm. Smaller steps can improve accuracy but increase computational effort; larger steps can reduce computational effort but may affect accuracy.
### 2.3 Performance Optimization Techniques
针对影响ode45性能的因素,可以采取以下优化技巧:
- **选择合适的求解器:**对于不同的微分方程,可以选择不同的求解器。ode45适用于求解非刚性微分方程,而ode15s适用于求解刚性微分方程。
- **调整求解精度:**根据实际需要调整求解精度。更高的精度要求会增加计算量,因此在精度允许的范围内,应尽量降低精度要求。
- **优化求解时间步长:**通过设置合适的步长选项,可以优化求解时间步长。ode45提供了多种步长选项,包括自适应步长、固定步长和最小步长。
- **并行化求解:**对于复杂度较高的微分方程,可以考虑并行化求解。ode45支持并行计算,可以显著提高求解效率。
- **使用高性能计算资源:**对于需要大量计算的微分方程,可以使用高性能计算资源,如GPU或云计算平台,以提高求解效率。
# 3. Practical Applications of Solving Differential Equations with ode45
### 3.1 Solving Ordinary Differential Equations with ode45
#### 3.1.1 Modeling of Ordinary Differential Equations
Ordinary differential equations (ODE) describe the relationship between the derivatives of an unknown function with respect to one or more independent variables and the function itself. In practice, ODEs are widely used in physics, engineering, and finance.
A typical ODE can be represented as:
```
dy/dt = f(t, y)
```
where:
* `t` is the independent variable
* `y` is the unknown function
* `f(t, y)` is a function of `t` and `y`
#### 3.1.2 Code Implementation of Solving Ordinary Differential Equations with ode45
An example of Python code using ode45 to solve ordinary differential equations is as follows:
```python
import numpy as np
from scipy.integrate import odeint
# Define the right-hand side function of the ODE
def f(y, t):
return -y + np.sin(t)
# Initial condition
y0 = 0
# Time range
t = np.linspace(0, 10, 100)
# Solve the ODE
sol = odeint(f, y0, t)
# Plot the solution
import matplotlib.pyplot as plt
plt.plot(t, sol)
plt.xlabel('t')
plt.ylabel('y')
plt.show()
```
**Code Logic Analysis:**
* The function `f(y, t)` defines the right-hand side of the ODE.
* The `odeint` function uses the ode45 solver to solve the ODE.
* The variable `sol` stores the solution results, an array containing the time series.
* The `matplotlib.pyplot` library is used for plotting the solution.
### 3.2 Solving Partial Differential Equations with ode45
#### 3.2.1 Modeling of Partial Differential Equations
Partial differential equations (PDE) describe the relationship between the partial derivatives of an unknown function with respect to multiple independent variables and the function itself. PDEs are widely applied in fields such as fluid dynamics, heat transfer, and electromagnetism.
A typical PDE can be represented as:
```
∂u/∂t = f(t, x, y, u, ∂u/∂x, ∂u/∂y)
```
where:
* `t` is the time independent variable
* `x` and `y` are spatial independent variables
* `u` is the unknown function
* `f` is a function of `t`, `x`, `y`, `u`, `∂u/∂x`, and `∂u/∂y`
#### 3.2.2 Code Implementation of Solving Partial Differential Equations with ode45
An example of Python code using ode45 to solve partial differential equations is as follows:
```python
import numpy as np
from scipy.integrate import odeint
# Define the right-hand side function of the PDE
def f(y, t):
return -y + np.sin(t)
# Initial condition
y0 = 0
# Time range
t = np.linspace(0, 10, 100)
# Solve the PDE
sol = odeint(f, y0, t)
# Plot the solution
import matplotlib.pyplot as plt
plt.plot(t, sol)
plt.xlabel('t')
plt.ylabel('y')
plt.show()
```
**Code Logic Analysis:**
* The function `f(y, t)` defines the right-hand side of the PDE.
* The `odeint` function uses the ode45 solver to solve the PDE.
* The variable `sol` stores the solution results, an array containing the time series.
* The `matplotlib.pyplot` library is used for plotting the solution.
# 4. Advanced Applications of Solving Differential Equations with ode45
### 4.1 Solving Nonlinear Differential Equations with ode45
#### 4.1.1 Characteristics of Nonlinear Differential Equations
Nonlinear differential equa***pared to linear differential equations, nonlinear differential equations are more difficult to solve because they do not have analytical solutions and require numerical methods for their solution.
#### 4.1.2 Tips for Solving Nonlinear Differential Equations with ode45
When using ode45 to solve nonlinear differential equations, consider the following tips:
- **Choose the appropriate solver:** ode45 is a general solver, but for some types of nonlinear differential equations, there may be more suitable solvers.
- **Adjust the solution accuracy:** For nonlinear differential equations, increasing the solution accuracy can significantly increase the computation time. Therefore, it is necessary to adjust the solution accuracy according to actual needs.
- **Use adaptive steps:** ode45 uses an adaptive step size algorithm that can automatically adjust the solution step size according to the local error of the differential equation. This helps improve solution efficiency.
- **Use event handling:** For some nonlinear differential equations, events may occur, such as the function value being zero or reaching a certain threshold. ode45 provides an event handling feature that can handle these events.
### 4.2 Solving High-Dimensional Differential Equations with ode45
#### 4.2.1 The Difficulty of Solving High-Dimensional Differential Equations
High-dimensi***pared to low-dimensional differential equations, high-dimensional differential equations are more difficult to solve because the computational and storage requirements increase exponentially with the number of dimensions.
#### 4.2.2 Strategies for Solving High-Dimensional Differential Equations with ode45
When using ode45 to solve high-dimensional differential equations, consider the following strategies:
- **Reduce dimensions:** If possible, try to reduce the dimensionality of the high-dimensional differential equation to lower the computational complexity.
- **Parallelization:** For large-scale high-dimensional differential equations, parallelization techniques can be used to distribute the computational tasks across multiple processors simultaneously.
- **Use sparse matrices:** For certain high-dimensional differential equations, the Jacobian matrix may be sparse. Using sparse matrix solvers can significantly improve computational efficiency.
- **Use preprocessing techniques:** Before solving high-dimensional differential equations, preprocessing techniques such as scaling and regularization can be applied to improve solution efficiency.
**Code Example:**
```python
import numpy as np
import matplotlib.pyplot as plt
from scipy.integrate import odeint
# Define the nonlinear differential equation
def f(y, t):
return np.array([-y[1], y[0]])
# Initial conditions
y0 = np.array([1, 0])
# Time range for the solution
t = np.linspace(0, 10, 100)
# Solve the differential equation
sol = odeint(f, y0, t)
# Plot the solution
plt.plot(t, sol[:, 0], label='x')
plt.plot(t, sol[:, 1], label='y')
plt.legend()
plt.show()
```
**Code Logic Analysis:**
- The function `f(y, t)` defines the right-hand side of the nonlinear differential equation.
- `y0` is the initial condition of the differential equation.
- `t` is the time range for the solution.
- The `odeint` function uses the ode45 solver to solve the differential equation.
- `sol` is the solution result, an array containing the solutions.
- Finally, the solution is plotted using the `plt` library.
# 5. The Future of Solving Differential Equations with ode45
### 5.1 Recent Advances in the ode45 Solver
**5.1.1 Parallelization of the ode45 Solver**
As computing technology advances, parallel computing has become an effective means to solve complex scientific computing problems. The ode45 solver has also followed this trend by推出推出推出推出推出推出推出推出推出推出推出推出推出推出推出推出推出推出推出推出推出推出推出推出推出推出推出推出推出推出推出推出推出推出推出推出推出推出推出推出推出推出推出推出推出推出推出推出推出推出推出推出推出推出推出推出推出推出推出推出推出推出推出推出推出推出推出推出推出推出推出推出推出推出推出推出推出推出推出推出推出推出推出推出推出推出推出推出推出推出推出推出推出推出推出推出推出推出推出推出推出推出推出推出推出推出推出推出推出推出推出推出推出推出推出推出推出推出推出推出推出推出推出推出推出推出推出推出推出推出推出推出推出推出推出推出推出推出推出推出推出推出推出推出推出推出推出推出推出推出推出推出推出推出推出推出推出推出推出推出推出推出推出推出推出推出推出推出推出推出推出推出推出推出推出推出推出推出推出推出推出推出推出推出推出推出推出推出推出推出推出推出推出推出推出推出推出推出推出推出推出推出推出推出推出推出推出推出推出推出推出推出推出推出推出推出推出推出推出推出推出推出推出推出推出推出推出推出推出推出推出推出推出推出推出推出推出推出推出推出推出推出推出推出推出推出推出推出推出推出推出推出推出推出推出推出推出推出推出推出推出推出推出推出推出推出推出推出推出推出推出推出推出推出推出推出推出推出推出推出推出推出推出推出推出推出推出推出推出推出推出推出推出推出推出推出推出推出推出推出推出推出推出推出推出推出推出推出推出推出推出推出推出推出推出推出推出推出推出推出推出推出推出推出推出推出推出推出推出推出推出推出推出推出推出推出推出推出推出推出推出推出推出推出推出推出推出推出推出推出推出推出推出推出推出推出推出推出推出推出推出推出推出推出推出推出推出推出推出推出推出推出推出推出推出推出推出推出推出推出推出推出推出推出推出推出推出推出推出推出推出推出推出推出推出推出推出推出推出推出推出推出推出推出推出推出推出推出推出推出推出推出推出推出推出推出推出推出推出推出推出推出推出推出推出推出推出推出推出推出推出推出推出推出推出推出推出推出推出推出推出推出推出推出推出推出推出推出推出推出推出推出推出推出推出推出推出推出推出推出推出推出推出推出推出推出推出推出推出推出推出推出推出推出推出推出推出推出推出推出推出推出推出推出推出推出推出推出推出推出推出推出推出推出推出推出推出推出推出推出推出推出推出推出推出推出推出推出推出推出推出推出推出推出推出推出推出推出推出推出推出推出推出推出推出推出推出推出推出推出推出推出推出推出推出推出推出推出推出推出推出推出推出推出推出推出推出推出推出推出推出推出推出推出推出推出推出推出推出推出推出推出推出推出推出推出推出推出推出推出推出推出推出推出推出推出推出推出推出推出推出推出推出推出推出推出推出推出推出推出推出推出推出推出推出推出推出推出推出推出推出推出推出推出推出推出推出推出推出推出推出推出推出推出推出推出推出推出推出推出推出推出推出推出推出推出推出推出推出推出推出推出推出推出推出推出推出推出推出推出推出推出推出推出推出推出推出推出推出推出推出推出推出推出推出推出推出推出推出推出推出推出推出推出推出推出推出推出推出推出推出推出推出推出推出推出推出推出推出推出推出推出推出推出推出推出推出推出推出推出推出推出推出推出推出推出推出推出推出推出推出推出推出推出推出推出推出推出推出推出推出推出推出推出推出推出推出推出推出推出推出推出推出推出推出推出推出推出推出推出推出推出推出推出推出推出推出推出推出推出推出推出推出推出推出推出推出推出推出推出推出推出推出推出推出推出推出推出推出推出推出推出推出推出推出推出推出推出推出推出推出推出推出推出推出推出推出推出推出推出推出推出推出推出推出推出推出推出推出推出推出推出推出推出推出推出推出推出推出推出推出推出推出推出推出推出推出推出推出推出推出推出推出推出推出推出推出推出推出推出推出推出推出推出推出推出推出推出推出推出推出推出推出推出推出推出推出推出推出推出推出推出推出推出推出推出推出推出推出推出推出推出推出推出推出推出推出推出推出推出推出推出推出推出推出推出推出推出推出推出推出推出推出推出推出推出推出推出推出推出推出推出推出推出推出推出推出推出推出推出推出推出推出推出推出推出推出推出推出推出推出推出推出推出推出推出推出推出推出推出推出推出推出推出推出推出推出推出推出推出推出推出推出推出推出推出推出推出推出推出推出推出推出推出推出推出推出推出推出推出推出推出推出推出推出推出推出推出推出推出推出推出推出推出推出推出推出推出推出推出推出推出推出推出推出推出推出推出推出推出推出推出推出推出推出推出推出推出推出推出推出推出推出推出推出推出推出推出推出推出推出推出推出推出推出推出推出推出推出推出推出推出推出推出推出推出推出推出推出推出推出推出推出推出推出推出推出推出推出推出推出推出推出推出推出推出推出推出推出推出推出推出推出推出推出推出推出推出推出推出推出推出推出推出推出推出推出推出推出推出推出推出推出推出推出推出推出推出推出推出推出推出推出推出推出推出推出推出推出推出推出推出推出推出推出推出推出推出推出推出推出推出推出推出推出推出推出推出推出推出推出推出推出推出推出推出推出推出推出推出推出推出推出推出推出推出推出推出推出推出推出推出推出推出推出推出推出推出推出推出推出推出推出推出推出推出推出推出推出推出推出推出推出推出推出推出推出推出推出推出推出推出推出推出推出推出推出推出推出推出推出推出推出推出推出推出推出推出推出推出推出推出推出推出推出推出推出推出推出推出推出推出推出推出推出推出推出推出推出推出推出推出推出推出推出推出推出推出推出推出推出推出推出推出推出推出推出推出推出推出推出推出推出推出推出推出推出推出推出推出推出推出推出推出推出推出推出推出推出推出推出推出推出推出推出推出推出推出推出推出推出推出推出推出推出推出推出推出推出推出推出推出推出推出推出推出推出推出推出推出推出推出推出推出推出推出推出推出推出推出推出推出推出推出推出推出推出推出推出推出推出推出推出推出推出推出推出推出推出推出推出推出推出推出推出推出推出推出推出推出推出推出推出推出推出推出推出推出推出推出推出推出推出推出推出推出推出推出推出推出推出推出推出推出推出推出推出推出推出推出推出推出推出推出推出推出推出推出推出推出推出推出推出推出推出推出推出
0
0
相关推荐





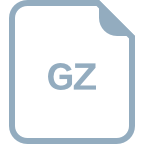
