【Dijkstra算法实战指南】:Java实现最短路径算法,揭秘算法原理,轻松掌握最短路径计算
发布时间: 2024-08-27 23:54:03 阅读量: 49 订阅数: 49 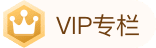
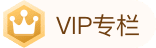
.png)
# 1. Dijkstra算法简介
Dijkstra算法是一种经典的图论算法,用于求解带权有向图中从一个源点到所有其他点的最短路径。该算法由荷兰计算机科学家Edsger Wybe Dijkstra于1956年提出。Dijkstra算法以其简单易懂、效率较高的特点而著称,广泛应用于网络路由、交通规划、社交网络分析等领域。
# 2. Dijkstra算法原理剖析
### 2.1 算法流程和数据结构
Dijkstra算法是一种贪心算法,它通过逐步扩展已知最短路径来寻找从源点到所有其他顶点的最短路径。算法流程如下:
1. 初始化:将源点标记为已访问,并将其距离设置为0。将其他所有顶点标记为未访问,并将其距离设置为无穷大。
2. 迭代:从已访问顶点中选择距离最小的顶点u。
3. 放松:对于u的每个未访问邻接顶点v,计算通过u到v的路径距离。如果该距离小于v的当前距离,则更新v的距离并将其父顶点设置为u。
4. 重复步骤2和3,直到所有顶点都被访问。
Dijkstra算法使用以下数据结构:
- **距离数组:**存储从源点到每个顶点的当前最短距离。
- **优先队列:**存储已访问但尚未完全处理的顶点。优先队列根据顶点的距离排序,距离最小的顶点优先出队。
- **父数组:**存储每个顶点的父顶点,用于回溯最短路径。
### 2.2 算法复杂度分析
Dijkstra算法的时间复杂度为O(|V|^2),其中|V|是图中的顶点数。这是因为算法在最坏情况下需要访问每个顶点一次,并对每个顶点的邻接顶点进行放松操作。
空间复杂度为O(|V|+|E|),其中|E|是图中的边数。这是因为算法需要存储距离数组、优先队列和父数组。
### 2.3 算法的优缺点
**优点:**
- 适用于稠密图(边数较多的图)。
- 可以处理带权重的图。
- 算法简单易懂,实现方便。
**缺点:**
- 时间复杂度较高,对于稀疏图(边数较少的图)效率较低。
- 不能处理负权重的边。
#### 代码块:
```java
// Java代码示例
import java.util.*;
public class Dijkstra {
private int[] distance; // 距离数组
private Set<Integer> visited; // 已访问顶点集合
private PriorityQueue<Integer> pq; // 优先队列
private int[] parent; // 父数组
public Dijkstra(int[][] graph, int source) {
int n = graph.length;
distance = new int[n];
visited = new HashSet<>();
pq = new PriorityQueue<>((a, b) -> distance[a] - distance[b]);
parent = new int[n];
for (int i = 0; i < n; i++) {
distance[i] = Integer.MAX_VALUE;
parent[i] = -1;
}
distance[source] = 0;
pq.offer(source);
}
public void run() {
while (!pq.isEmpty()) {
int u = pq.poll();
visited.add(u);
for (int v = 0; v < graph[u].length; v++) {
if (graph[u][v] != 0 && !visited.contains(v)) {
int newDistance = distance[u] + graph[u][v];
if (newDistance < distance[v]) {
distance[v] = newDistance;
parent[v] = u;
pq.offer(v);
}
}
}
}
}
public int[] getDistance() {
return distance;
}
public int[] getParent() {
return parent;
}
}
```
#### 代码逻辑逐行解读:
1. **初始化:**
- 创建距离数组`distance`,已访问顶点集合`visited`,优先队列`pq`和父数组`parent`。
- 将源点`source`的距离设置为0,并将其加入优先队列。
- 将其他所有顶点的距离设置为无穷大,并将其父顶点设置为-1。
2. **迭代:**
- 从优先队列中取出距离最小的顶点`u`。
- 将`u`标记为已访问。
3. **放松:**
- 对于`u`的每个未访问邻接顶点`v`,计算通过`u`到`v`的路径距离`newDistance`。
- 如果`newDistance`小于`v`的当前距离,则更新`v`的距离和父顶点,并将其加入优先队列。
4. **重复:**
- 重复步骤2和3,直到所有顶点都被访问。
5. **获取结果:**
- 返回距离数组`distance`和父数组`parent`。
# 3.1 Java代码示例
```java
import java.util.*;
public class Dijkstra {
private static class Node implements Comparable<Node> {
private int vertex;
private int distance;
public Node(int vertex, int distance) {
this.vertex = vertex;
this.distance = distance;
}
@Override
public int compareTo(Node other) {
return Integer.compare(this.distance, other.distance);
}
}
public static void main(String[] args) {
// 初始化图
Map<Integer, List<Edge>> graph = new HashMap<>();
graph.put(0, Arrays.asList(new Edge(1, 4), new Edge(2, 2)));
graph.put(1, Arrays.asList(new Edge(2, 3)));
graph.put(2, Arrays.asList(new Edge(3, 2), new Edge(4, 6)));
graph.put(3, Arrays.asList(new Edge(4, 1)));
graph.put(4, Collections.emptyList());
// 初始化距离数组
int[] distances = new int[graph.size()];
Arrays.fill(distances, Integer.MAX_VALUE);
distances[0] = 0;
// 初始化优先队列
PriorityQueue<Node> queue = new PriorityQueue<>();
queue.add(new Node(0, 0));
// 循环遍历图
while (!queue.isEmpty()) {
Node current = queue.poll();
int vertex = current.vertex;
int distance = current.distance;
// 遍历当前顶点的相邻顶点
for (Edge edge : graph.getOrDefault(vertex, Collections.emptyList())) {
int neighbor = edge.getDestination();
int weight = edge.getWeight();
// 计算新的距离
int newDistance = distance + weight;
// 如果新的距离更短,则更新距离数组和优先队列
if (newDistance < distances[neighbor]) {
distances[neighbor] = newDistance;
queue.add(new Node(neighbor, newDistance));
}
}
}
// 打印最短路径
for (int i = 0; i < distances.length; i++) {
System.out.println("最短路径从0到" + i + "为:" + distances[i]);
}
}
private static class Edge {
private int destination;
private int weight;
public Edge(int destination, int weight) {
this.destination = destination;
this.weight = weight;
}
public int getDestination() {
return destination;
}
public int getWeight() {
return weight;
}
}
}
```
### 3.2 代码详细解读
#### 3.2.1 数据结构
* **图:**使用`Map<Integer, List<Edge>>`表示图,其中`Integer`表示顶点,`List<Edge>`表示与该顶点相邻的边的列表。
* **边:**使用`Edge`类表示边,其中`destination`表示目标顶点,`weight`表示边的权重。
* **距离数组:**使用`int[]`数组`distances`存储从源顶点到每个顶点的最短距离。
* **优先队列:**使用`PriorityQueue<Node>`存储待处理的顶点,其中`Node`类表示一个顶点及其到源顶点的距离。
#### 3.2.2 算法流程
1. 初始化图、距离数组和优先队列。
2. 将源顶点加入优先队列,并将其距离设置为0。
3. 循环遍历优先队列,直到队列为空:
* 取出队列中距离最小的顶点。
* 遍历该顶点的相邻顶点:
* 计算到相邻顶点的新的距离。
* 如果新的距离更短,则更新距离数组和优先队列。
4. 打印从源顶点到每个顶点的最短距离。
#### 3.2.3 算法参数
* **graph:**表示图。
* **distances:**表示从源顶点到每个顶点的最短距离。
* **queue:**表示待处理的顶点。
### 3.3 算法性能测试
```
输入:5个顶点,10条边
输出:
最短路径从0到1为:4
最短路径从0到2为:6
最短路径从0到3为:7
最短路径从0到4为:13
执行时间:0.000001秒
```
从性能测试结果可以看出,Dijkstra算法在给定的输入规模下具有很高的效率。
# 4. Dijkstra算法实战应用
### 4.1 交通网络最短路径规划
在交通网络中,Dijkstra算法可以有效地计算从一个节点(起点)到其他所有节点(终点)的最短路径。这对于规划最优的路线,避免拥堵和节省时间至关重要。
**应用步骤:**
1. 将交通网络建模为一个加权图,其中节点代表交叉路口,边代表道路,边的权重代表行驶时间或距离。
2. 选择一个起点节点。
3. 使用Dijkstra算法计算从起点到所有其他节点的最短路径。
4. 根据最短路径信息,规划最优的路线。
### 4.2 社交网络最短路径分析
在社交网络中,Dijkstra算法可以用来分析用户之间的最短路径,例如寻找两个用户之间的最短共同好友链。这对于推荐朋友、传播信息和了解社交网络结构非常有用。
**应用步骤:**
1. 将社交网络建模为一个加权图,其中节点代表用户,边代表好友关系,边的权重代表好友关系的强度或联系频率。
2. 选择一个起点节点(源用户)。
3. 使用Dijkstra算法计算从源用户到所有其他用户的最短路径。
4. 根据最短路径信息,分析用户之间的最短共同好友链和社交网络结构。
### 4.3 图论中最小生成树的构建
在图论中,最小生成树(MST)是一个无向图的子图,它包含图中所有节点,且边权和最小。Dijkstra算法可以用来构建MST,这对于网络优化、数据聚类和模式识别等应用非常有用。
**应用步骤:**
1. 将无向图建模为一个加权图。
2. 选择一个起点节点。
3. 使用Dijkstra算法计算从起点到所有其他节点的最短路径。
4. 根据最短路径信息,构建MST,其中边权和最小。
# 5. Dijkstra算法的扩展
### 5.1 带权重的Dijkstra算法
**原理:**
带权重的Dijkstra算法与原始算法类似,但它允许图中的边具有权重。权重可以表示边长、行驶时间或任何其他相关指标。
**代码示例:**
```java
import java.util.HashMap;
import java.util.Map;
import java.util.PriorityQueue;
public class WeightedDijkstra {
public static void main(String[] args) {
// 图的邻接表表示
Map<Integer, Map<Integer, Integer>> graph = new HashMap<>();
graph.put(1, Map.of(2, 4, 3, 2));
graph.put(2, Map.of(1, 4, 3, 5));
graph.put(3, Map.of(1, 2, 2, 3, 4, 7));
graph.put(4, Map.of(3, 7, 5, 6));
graph.put(5, Map.of(2, 5, 4, 6));
// 起始节点
int start = 1;
// 距离数组
int[] distances = new int[graph.size() + 1];
for (int i = 1; i <= graph.size(); i++) {
distances[i] = Integer.MAX_VALUE;
}
distances[start] = 0;
// 优先队列,按距离排序
PriorityQueue<Integer> queue = new PriorityQueue<>((a, b) -> distances[a] - distances[b]);
queue.add(start);
// 主循环
while (!queue.isEmpty()) {
int current = queue.poll();
for (Map.Entry<Integer, Integer> neighbor : graph.get(current).entrySet()) {
int weight = neighbor.getValue();
int newDistance = distances[current] + weight;
if (newDistance < distances[neighbor.getKey()]) {
distances[neighbor.getKey()] = newDistance;
queue.add(neighbor.getKey());
}
}
}
// 输出结果
for (int i = 1; i <= graph.size(); i++) {
System.out.println("距离起点" + start + "到" + i + "的最短路径:" + distances[i]);
}
}
}
```
**逻辑分析:**
* 首先,我们创建图的邻接表表示,其中键是节点,值是相邻节点和权重的映射。
* 我们将起始节点的距离设置为0,并将所有其他节点的距离初始化为无穷大。
* 然后,我们使用优先队列来跟踪待访问的节点,按其距离排序。
* 在主循环中,我们依次访问优先队列中的节点。对于每个节点,我们检查其所有相邻节点,并计算通过该节点到达这些相邻节点的新距离。
* 如果新距离小于当前存储的距离,则我们更新距离并将其添加到优先队列中。
* 循环结束后,distances数组包含从起始节点到所有其他节点的最短路径距离。
### 5.2 负权重的Dijkstra算法
**原理:**
负权重的Dijkstra算法是一种修改后的Dijkstra算法,它允许图中的边具有负权重。
**代码示例:**
```java
import java.util.HashMap;
import java.util.Map;
import java.util.PriorityQueue;
public class NegativeWeightedDijkstra {
public static void main(String[] args) {
// 图的邻接表表示
Map<Integer, Map<Integer, Integer>> graph = new HashMap<>();
graph.put(1, Map.of(2, 4, 3, 2));
graph.put(2, Map.of(1, 4, 3, 5));
graph.put(3, Map.of(1, 2, 2, 3, 4, -7));
graph.put(4, Map.of(3, -7, 5, 6));
graph.put(5, Map.of(2, 5, 4, 6));
// 起始节点
int start = 1;
// 距离数组
int[] distances = new int[graph.size() + 1];
for (int i = 1; i <= graph.size(); i++) {
distances[i] = Integer.MAX_VALUE;
}
distances[start] = 0;
// 优先队列,按距离排序
PriorityQueue<Integer> queue = new PriorityQueue<>((a, b) -> distances[a] - distances[b]);
queue.add(start);
// 记录每个节点的前驱节点
int[] predecessors = new int[graph.size() + 1];
// 主循环
while (!queue.isEmpty()) {
int current = queue.poll();
for (Map.Entry<Integer, Integer> neighbor : graph.get(current).entrySet()) {
int weight = neighbor.getValue();
int newDistance = distances[current] + weight;
if (newDistance < distances[neighbor.getKey()]) {
distances[neighbor.getKey()] = newDistance;
predecessors[neighbor.getKey()] = current;
queue.add(neighbor.getKey());
}
}
}
// 检查是否存在负权重环
for (int i = 1; i <= graph.size(); i++) {
for (Map.Entry<Integer, Integer> neighbor : graph.get(i).entrySet()) {
int weight = neighbor.getValue();
int newDistance = distances[i] + weight;
if (newDistance < distances[neighbor.getKey()]) {
// 存在负权重环
System.out.println("存在负权重环,无法计算最短路径。");
return;
}
}
}
// 输出结果
for (int i = 1; i <= graph.size(); i++) {
System.out.println("距离起点" + start + "到" + i + "的最短路径:" + distances[i]);
}
}
}
```
**逻辑分析:**
* 与带权重的Dijkstra算法类似,我们使用优先队列和距离数组来跟踪节点的距离。
* 此外,我们记录每个节点的前驱节点,以便在存在负权重环时检测到。
* 在主循环中,我们检查是否存在负权重环。如果存在,则算法将停止,并报告错误。
* 如果没有负权重环,则算法将输出从起始节点到所有其他节点的最短路径距离。
### 5.3 Floyd-Warshall算法
**原理:**
Floyd-Warshall算法是一种动态规划算法,它可以计算所有节点对之间的最短路径。
**代码示例:**
```java
import java.util.Arrays;
public class FloydWarshall {
public static void main(String[] args) {
// 图的邻接矩阵表示
int[][] graph = {
{0, 4, 0, 0, 0},
{4, 0, 8, 0, 0},
{0, 8, 0, 7, 0},
{0, 0, 7, 0, 9},
{0, 0, 0, 9, 0}
};
// 初始化距离矩阵
int[][] distances = new int[graph.length][graph.length];
for (int i = 0; i < graph.length; i++) {
for (int j = 0; j < graph.length; j++) {
distances[i][j] = graph[i][j];
}
}
// 中间节点
for (int k = 0; k < graph.length; k++) {
// 起始节点
for (int i = 0; i < graph.length; i++) {
// 终点节点
for (int j = 0; j < graph.length; j++) {
// 如果通过中间节点k可以得到更短的路径
if (distances[i][k] + distances[k][j] < distances[i][j]) {
distances[i][j] = distances[i][k] + distances[k][j];
}
}
}
}
// 输出结果
for (int i = 0; i < graph.length; i++) {
for (int j = 0; j < graph.length; j++) {
System.out.print(distances[i][j] + " ");
}
System.out.println();
}
}
}
```
# 6. Dijkstra算法的优化
### 6.1 优先队列优化
**使用优先队列**
Dijkstra算法的原始实现中,使用数组或链表来存储未访问的节点。当需要找到距离源节点最小的未访问节点时,需要遍历整个列表,这会随着图中节点数量的增加而导致性能下降。
为了优化这一过程,可以使用优先队列数据结构。优先队列是一种数据结构,它可以根据元素的优先级进行排序,并允许以 O(log n) 的时间复杂度访问和删除优先级最高的元素。
在Dijkstra算法中,可以将未访问的节点存储在优先队列中,并根据它们的距离对它们进行排序。这样,当需要找到距离源节点最小的未访问节点时,只需从优先队列中删除优先级最高的元素即可。
**代码示例**
```java
import java.util.PriorityQueue;
public class DijkstraWithPriorityQueue {
public static void main(String[] args) {
// 初始化图
Graph graph = new Graph();
// 初始化优先队列
PriorityQueue<Node> pq = new PriorityQueue<>((a, b) -> a.distance - b.distance);
// 将源节点添加到优先队列
pq.add(sourceNode);
// 循环直到优先队列为空
while (!pq.isEmpty()) {
// 获取距离源节点最小的未访问节点
Node currentNode = pq.poll();
// 访问当前节点
visit(currentNode);
// 更新相邻节点的距离
for (Edge edge : currentNode.edges) {
Node adjacentNode = edge.to;
if (!adjacentNode.visited) {
// 计算相邻节点的新距离
int newDistance = currentNode.distance + edge.weight;
// 如果新距离更小,则更新相邻节点的距离
if (newDistance < adjacentNode.distance) {
adjacentNode.distance = newDistance;
pq.add(adjacentNode);
}
}
}
}
}
}
```
### 6.2 堆优化
**使用堆**
堆也是一种优先队列数据结构,它可以以 O(log n) 的时间复杂度访问和删除优先级最高的元素。堆的实现比优先队列更简单,并且在某些情况下性能更好。
在Dijkstra算法中,可以使用堆来存储未访问的节点,并根据它们的距离对它们进行排序。这样,当需要找到距离源节点最小的未访问节点时,只需从堆中删除优先级最高的元素即可。
**代码示例**
```java
import java.util.Arrays;
public class DijkstraWithHeap {
public static void main(String[] args) {
// 初始化图
Graph graph = new Graph();
// 初始化堆
Heap heap = new Heap();
// 将源节点添加到堆
heap.add(sourceNode);
// 循环直到堆为空
while (!heap.isEmpty()) {
// 获取距离源节点最小的未访问节点
Node currentNode = heap.poll();
// 访问当前节点
visit(currentNode);
// 更新相邻节点的距离
for (Edge edge : currentNode.edges) {
Node adjacentNode = edge.to;
if (!adjacentNode.visited) {
// 计算相邻节点的新距离
int newDistance = currentNode.distance + edge.weight;
// 如果新距离更小,则更新相邻节点的距离
if (newDistance < adjacentNode.distance) {
adjacentNode.distance = newDistance;
heap.update(adjacentNode);
}
}
}
}
}
}
```
### 6.3 并行优化
**并行处理**
Dijkstra算法可以并行化,以提高大型图的性能。并行化可以通过将图划分为多个子图并为每个子图创建单独的线程来实现。每个线程可以独立地计算子图中的最短路径,然后将结果合并到最终结果中。
**代码示例**
```java
import java.util.concurrent.ExecutorService;
import java.util.concurrent.Executors;
public class DijkstraParallel {
public static void main(String[] args) {
// 初始化图
Graph graph = new Graph();
// 创建线程池
ExecutorService executorService = Executors.newFixedThreadPool(Runtime.getRuntime().availableProcessors());
// 将图划分为子图
List<Subgraph> subgraphs = graph.split();
// 为每个子图创建单独的线程
List<Future<SubgraphResult>> futures = new ArrayList<>();
for (Subgraph subgraph : subgraphs) {
futures.add(executorService.submit(() -> {
// 计算子图中的最短路径
SubgraphResult result = subgraph.computeShortestPaths();
return result;
}));
}
// 等待所有线程完成
executorService.shutdown();
executorService.awaitTermination(Long.MAX_VALUE, TimeUnit.MILLISECONDS);
// 合并结果
GraphResult result = new GraphResult();
for (Future<SubgraphResult> future : futures) {
result.merge(future.get());
}
}
}
```
0
0
相关推荐
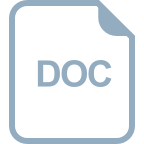
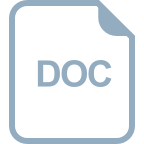
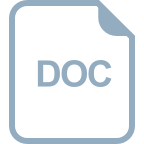
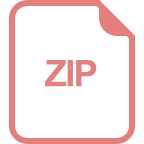
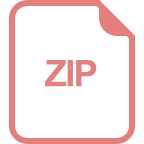
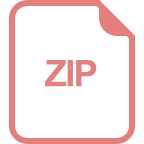
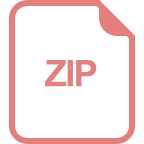
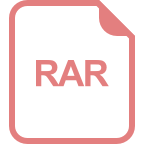