Application of MATLAB Gaussian Fitting in Signal Processing: Extracting Useful Information from Noise to Enhance Signal Clarity
发布时间: 2024-09-14 19:29:00 阅读量: 16 订阅数: 20 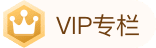
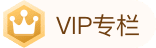
# Application of MATLAB Gaussian Fitting in Signal Processing: Extracting Useful Information from Noise and Enhancing Signal Clarity

## 1. Overview of MATLAB and the Theory of Gaussian Fitting
MATLAB is an advanced programming language widely used in scientific computing and data analysis. It provides an interactive environment that allows users to perform complex mathematical and scientific calculations with ease.
Gaussian fitting is a statistical modeling technique used to fit curves for normal distributions, also known as Gaussian distributions. A normal distribution is a common probability distribution characterized by a symmetric bell-shaped curve. In signal processing, Gaussian fitting is employed to analyze and process signals that exhibit characteristics of a normal distribution.
## 2. Application of Gaussian Fitting in Si***
***
***
***
***
***
***
***
***
***
***
***
```matlab
% Generate noise signal
data = randn(1000, 1);
% Fit normal distribution
[mu, sigma] = normfit(data);
% Plot the fitting curve
x = linspace(-3, 3, 1000);
y = normpdf(x, mu, sigma);
plot(x, y, 'r', 'LineWidth', 2);
hold on;
scatter(data, zeros(size(data)), 50, 'b', 'filled');
legend('Fitting Curve', 'Noise Signal');
```
**Logical Analysis:**
- The `randn` function generates normally distributed random data.
- The `normfit` function uses the least squares method to fit the normal distribution.
- The `linspace` function generates linearly spaced points.
- The `normpdf` function calculates the probability density function of the normal distribution.
- The `plot` function draws the fitting curve.
- The `scatter` function plots the noise signal.
#### 2.2.2 Maximum Likelihood Estimation Method
The maximum likelihood estimation method is another commonly used Gaussian fitting algorithm. It finds the optimal fitting parameters by maximizing the likelihood function of the noise signal.
```matlab
% Generate noise signal
data = randn(1000, 1);
% Fit normal distribution
[mu, sigma] = mle(data, 'distribution', 'normal');
% Plot the fitting curve
x = linspace(-3, 3, 1000);
y = normpdf(x, mu, sigma);
plot(x, y, 'r', 'LineWidth', 2);
hold on;
scatter(data, zeros(size(data)), 50, 'b', 'filled');
legend('Fitting Curve', 'Noise Signal');
```
**Logical Analysis:**
- The `mle` function uses the maximum likelihood estimation method to fit the normal distribution.
- The rest of the code is similar to the least squares method.
## 3.1 Processing of Noise Signals
#### 3.1.1 Generation of Noise Signals
Noise signals are typically random and non-periodic, capable of simulating noise interference in real-world environments. In MATLAB, the `randn` function can be used to generate normally distributed noise signals, with the syntax:
```
noise = randn(m, n);
```
Here, `m` and `n` denote the number of rows and columns of the noise signal, respectively. For example, to generate a normally distributed noise signal with 100 rows and 100 columns:
```
noise = randn(100, 100);
```
#### 3.1.2 Denoising of Noise Signals Using Gaussian Fitting
Gaussian fitting can effectively remove high-frequency components from noise signals, thus achieving the goal of denoising. In MATLAB, the `fit` function can be used for Gaussian fitting of noise signals, with the syntax:
```
[fitresult, gof] = fit(x, y, 'gauss1');
```
Here, `x` and `y` represent the coordinates of the noise signal, `fitresult` is the fitting result, and `gof` is the goodness-of-fit information. For example, applying Gaussian fitting to the noise signal generated above:
```
[fitresult, gof] = fit(1:100, noise, 'gauss1');
```
The fitting resul
0
0
相关推荐
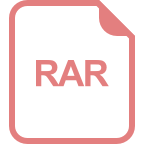
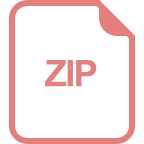
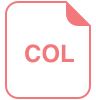
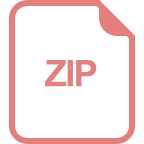
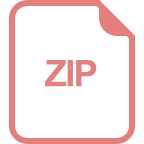
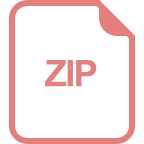
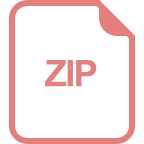
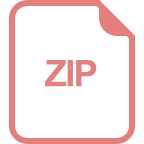