Optimization of MATLAB Gaussian Fitting Code: Enhancing Code Efficiency and Computing Performance
发布时间: 2024-09-14 19:36:09 阅读量: 18 订阅数: 20 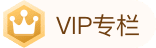
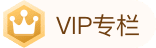
# 1. Overview of MATLAB Gaussian Fitting
Gaussian fitting is a common curve fitting technique used to fit data with Gaussian distribution characteristics. In MATLAB, Gaussian fitting can be achieved using the `fit` function. This function employs a nonlinear least squares algorithm, iteratively optimizing fitting parameters to minimize the fitting error.
The advantages of MATLAB Gaussian fitting include:
***High Precision:** The nonlinear least squares algorithm ensures a high fitting precision.
***Ease of Use:** The `fit` function provides a concise and user-friendly interface, simplifying the fitting process.
***Strong Scalability:** MATLAB's Gaussian fitting toolbox supports various data types and distributions, exhibiting strong scalability.
# 2. Theoretical Basis for MATLAB Gaussian Fitting Code Optimization
### 2.1 Mathematical Principles of Gaussian Fitting Algorithm
Gaussian fitting is a nonlinear least squares problem. Its goal is to find a set of parameters that minimizes the sum of squared residuals between the Gaussian function and a given dataset. The mathematical expression for the Gaussian function is:
```
f(x) = A * exp(-((x - μ) / σ)^2)
```
Where:
- `A` is the amplitude of the Gaussian function.
- `μ` is the center position of the Gaussian function.
- `σ` is the standard deviation of the Gaussian function.
Given a set of data points `(x_i, y_i)`, the objective function of the Gaussian fitting algorithm is:
```
S = ∑(y_i - f(x_i))^2
```
By minimizing the objective function `S`, the optimal parameters `A`, `μ`, `σ` can be found.
### 2.2 Principles and Methods for Code Optimization
When optimizing Gaussian fitting code in MATLAB, the following principles should be followed:
- **Reduce Redundant Calculations:** Avoid recalculating the same values, such as calculating the Gaussian function within a loop.
- **Leverage Vectorized Operations:** Use MATLAB's vectorized operations, such as `matrix multiplication` and `element-wise operations`, to significantly improve computational efficiency.
- **Optimize Data Structures:** Choose appropriate MATLAB data structures, such as sparse matrices, to improve storage efficiency and computational performance.
- **Parallelize Computations:** Utilize multi-core processors or GPUs to parallelize code, further enhancing computational efficiency.
# 3.1 Optimizing Code Structure and Algorithm
#### 3.1.1 Accelerating Calculations with Matrix Operations
MATLAB offers powerful matrix computation capabilities, which can effectively speed up Gaussian fitting calculations. By organizing data into matrices and using matrix operations, unnecessary loops and scalar operations can be avoided, thereby greatly enhancing computational efficiency.
```matlab
% Assuming data is stored in matrix X, with each sample in a row and each feature in a column
[n, m] = size(X); % Get the size of the data matrix
% Build the design matrix
A = [ones(n, 1), X]; % Add a column of all 1s as the bias term
% Calculate the solution to the normal equation
w = (A' * A) \ (A' * y);
```
**Line-by-line explanation of the code logic:**
* `[n, m] = size(X);` - Get the size of the data matrix, where `n` is the number of samples and `m` is the number of features.
* `A = [ones(n, 1), X];` - Construct the design matrix by adding a column of all 1s as the bias term in front of the data matrix `X`.
* `w = (A' * A) \ (A' * y);` - Use the normal equation to solve for the parameters `w` of the linear regression model. Matrix operations `A' * A` and `A' * y` calculate the covariance matrix of the design matrix and the product of the design matrix and the target vector `y`, respectively.
#### 3.1.2 Optimizing Loop Structures to Reduce Redundant Calculations
In Gaussian fitting code, there are often numerous loop operations. By optimizing loop structures, redundant calculations can be reduced, improving code efficiency.
```matlab
% Assuming we need to calculate the sum of squared residuals for each sample
residuals = zeros(n, 1); % Initialize the vector to store the sum of squared residuals for each sample
for i = 1:n
residuals(i) = norm(y(i) - A(i, :) * w)^2;
end
```
**Line-by-line explanation of the code logic:**
* `residuals = zeros(n, 1);` - Initialize the vector to store the sum of squared residuals for each sample.
* `for i = 1:n` - Use a `for` loop to iterate over each sample.
* `residuals(i) = norm(y(i) - A(i, :) * w)^2;` - Calculate the sum of s
0
0
相关推荐
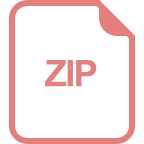
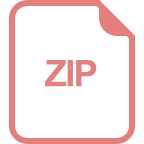
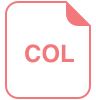
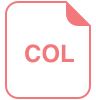
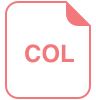
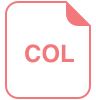
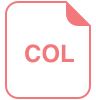
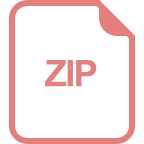
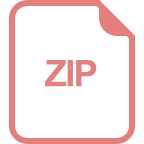