【Unveiling the Mystery of Gaussian Fitting in MATLAB: Master Fitting Techniques from Theory to Practice】
发布时间: 2024-09-14 19:21:40 阅读量: 21 订阅数: 19 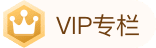
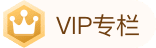
**【Demystifying MATLAB Gaussian Fitting】: Master the Fitting Techniques, from Theory to Practice**
# 1. Introduction to MATLAB Gaussian Fitting
Gaussian fitting is a statistical modeling technique based on the Gaussian distribution, used for fitting data and estimating its parameters. In MATLAB, Gaussian fitting can be achieved using the fitgmdist function.
The Gaussian distribution is a continuous probability distribution characterized by its bell-shaped curve. It is widely applied in nature and engineering to describe various phenomena, such as measurement errors, random noise, and signal strength.
The purpose of Gaussian fitting is to find a set of Gaussian distribution parameters (such as mean, variance, and weights) to best fit the given data. These parameters can describe the characteristics of the data distribution and are used for prediction and decision-making.
# 2. Theoretical Foundations of Gaussian Function
### 2.1 Mathematical Model of Gaussian Distribution
#### 2.1.1 One-dimensional Gaussian Distribution
The one-dimensional Gaussian distribution, also known as the normal distribution, has the probability density function:
```
f(x) = (1 / (σ√(2π))) * e^(-(x - μ)² / (2σ²))
```
Where:
* μ represents the mean, indicating the center position of the distribution.
* σ is the standard deviation, indicating the dispersion of the distribution.
#### 2.1.2 Multidimensional Gaussian Distribution
The multidimensional Gaussian distribution is the generalization of the Gaussian distribution in multidimensional space, with the probability density function:
```
f(x) = (1 / ((2π)^n |Σ|)^1/2)) * e^(-1/2 * (x - μ)^T Σ⁻¹ (x - μ))
```
Where:
* n is the dimension.
* μ is the mean vector.
* Σ is the covariance matrix, indicating the correlation between different dimensions.
### 2.2 Principles of Gaussian Fitting
Gaussian fitting is a type of nonlinear regression technique aimed at finding a set of parameters that make the Gaussian distribution model most suitable for the given data.
The principles of Gaussian fitting are as follows:
1. Define a Gaussian distribution model with unknown parameters.
2. Use optimization algorithms to minimize the error between the model and the data.
3. Obtain the optimal parameters, that is, the fitting parameters.
The fitting parameters include:
***Mean (μ):** The central position of the distribution.
***Standard Deviation (σ):** The dispersion of the distribution.
***Covariance Matrix (Σ):** The correlation between different dimensions (for multidimensional Gaussian distribution).
# 3. Practicing Gaussian Fitting in MATLAB
### 3.1 Data Preparation and Preprocessing
#### 3.1.1 Data Import and Visualization
Before Gaussian fitting, data must be imported into the MATLAB workspace. The following command can be used to import data:
```
data = importdata('data.csv');
```
Where 'data.csv' is the path to the data file.
After importing the data, the `plot` function can be used to visualize the data:
```
plot(data);
```
#### 3.1.2 Data Preprocessing and Noise Reduction
Before Gaussian fitting, ***mon preprocessing methods include:
***Smoothing filters:** Using smoothing filters (such as moving average filters) to remove high-frequency noise.
***De-trending:** Using de-trending methods (such as linear regression) to remove trends from the data.
***Outlier handling:** Identifying and removing outliers, such as using standard deviation thresholds or box plots.
### 3.2 Gaussian Fitting Functions
#### 3.2.1 Usage of the fitgmdist Function
The `fitgmdist` function is used for Gaussian fitting in MATLAB. The syntax for this function is:
```
gm = fitgmdist(data, nComponents, 'Options', options);
```
Where:
* `data` refers to the data to be fitted.
* `nComponents` is the number of Gaussian components in the Gaussian mixture model.
* `Options` is an optional parameter that specifies fitting options, such as the maximum number of iterations and tolerance.
#### 3.2.2 Selection of Regularization Parameters
The `fitgmdist` function has an important parameter `RegularizationValue`, which is used to control the model's regularization. Regularization helps prevent overfitting of the model. The range for the regularization parameter is from 0 to 1, where 0 indicates no regularization and 1 indicates full regularization.
The choice of regularization parameters depends on the noise level of the data and the complexity of the model. For data with high noise, a larger regularization parameter is needed to prevent overfitting. For data with low noise, a smaller regularization parameter can be used to achieve more accurate fitting.
# 4. Analysis of Gaussian Fitting Results
### 4.1 Parameter Estimation and Confidence Intervals
#### 4.1.1 Meaning and Interpretation of Parameters
The parameters of the Gaussian fitting model include mean (μ), standard deviation (σ), and amplitude (A).
***Mean (μ):** The central position of the Gaussian distribution, representing the average value of the data.
***Standard deviation (σ):** The width of the Gaussian distribution, indicating the dispersion of the data.
***Amplitude (A):** The peak height of the Gaussian distribution, representing the maximum value of the data.
### 4.1.2 Calculation of Confidence Intervals
Confidence intervals are a measure of the reliability of parameter estimates. For Gaussian fitting, confidence intervals can be calculated using the following formula:
```
μ ± z * σ / √n
```
Where:
* μ is the estimated value of the parameter.
* σ is the standard deviation of the parameter.
* n is the sample size of the data.
* z is the z-value corresponding to the confidence level.
### 4.2 Evaluating the Goodness of Model Fit
#### 4.2.1 Residual Analysis
Residuals are the differences between observed values and model-fitted values. Residual analysis can help assess the goodness of the model fit. Ideally, residuals should be randomly distributed around zero and show no patterns.
#### 4.2.2 R-Squared Value and Adjusted R-Squared Value
The R-squared value (R^2) is a common measure of model fit goodness. It represents the proportion of data variation explained by the model. R-squared values range from 0 to 1, with higher values indicating better model fit.
The Adjusted R-squared value (Adjusted R^2) is a correction to the R-squared value, taking into account the number of parameters in the model. Adjusted R-squared values are usually more reliable than R-squared values because they can prevent overfitting.
### 4.2.3 Model Selection
When performing Gaussian fitting, it is often necessary to select the most appropriate model. Model selection can be done through the following steps:
1. **Fit multiple models:** Fit multiple Gaussian models using different parameter combinations.
2. **Compare model goodness of fit:** Use R-squared values or Adjusted R-squared values to compare the goodness of fit of different models.
3. **Select the best model:** Choose the model with the highest R-squared value or Adjusted R-squared value.
### 4.2.4 Model Validation
Model validation is the process of evaluating the generalization ability of a model on unknown data. The following steps can be taken for model validation:
1. **Divide the data into training and test sets:** Split the dataset into two parts, where the training set is used to fit the model and the test set is used to evaluate the model.
2. **Fit the model on the training set:** Use the training set to fit the Gaussian model.
3. **Evaluate the model on the test set:** Use the test set to assess the goodness of fit of the model.
If the model's goodness of fit on the test set is similar to that on the training set, it indicates that the model has good generalization ability.
# 5. Cases of Gaussian Fitting in Practical Applications
**5.1 Denoising in Image Processing**
**5.1.1 Principles of Gaussian Filtering**
Gaussian filtering is a technique for image denoising that uses a Gaussian kernel to convolve with the image to smooth it, thereby removing noise. The Gaussian kernel is a weight matrix with a Gaussian distribution shape, where the center weight is the largest and decreases outward.
**5.1.2 Implementation of Gaussian Filtering in MATLAB**
The `imgaussfilt` function is used for Gaussian filtering in MATLAB. The syntax for this function is:
```
B = imgaussfilt(A, sigma)
```
Where:
* `A` is the input image.
* `sigma` is the standard deviation of the Gaussian kernel, controlling the smoothness of the filter.
* `B` is the output filtered image.
**Code Block:**
```
% Read in the image
image = imread('noisy_image.jpg');
% Gaussian filtering with sigma=2
filtered_image = imgaussfilt(image, 2);
% Display the original and filtered images
subplot(1,2,1);
imshow(image);
title('Original Image');
subplot(1,2,2);
imshow(filtered_image);
title('Image after Gaussian Filtering');
```
**Logical Analysis:**
* Read in the original image `image`.
* Use the `imgaussfilt` function to apply Gaussian filtering to the image, with `sigma` set to 2.
* Display the original and filtered images in two subplots.
**5.2 Peak Detection in Signal Processing**
**5.2.1 Principles of Peak Detection**
Peak detection is a technique in signal processing used to identify peaks in a signal. Gaussian fitting can be used for peak detection because it can fit the shape of signal peaks.
**5.2.2 Using Gaussian Fitting for Peak Detection in MATLAB**
The `findpeaks` function is used for peak detection in MATLAB. The syntax for this function is:
```
[peaks, locations] = findpeaks(signal, minPeakHeight, minPeakDistance)
```
Where:
* `signal` is the input signal.
* `minPeakHeight` is the minimum peak height.
* `minPeakDistance` is the minimum peak spacing.
* `peaks` are the peak values.
* `locations` are the peak positions.
**Code Block:**
```
% Read in the signal
signal = load('signal.mat');
% Gaussian fitting
[~, locations] = findpeaks(signal, 0.5, 10);
% Fit the Gaussian distribution
options = statset('MaxIter', 1000);
gm = fitgmdist(signal(locations), 1, 'Options', options);
% Display the signal and fitted Gaussian distribution
plot(signal);
hold on;
plot(locations, gm.mu, 'ro');
xlabel('Time');
ylabel('Amplitude');
title('Signal and Gaussian Fitting');
```
**Logical Analysis:**
* Read in the signal `signal`.
* Use the `findpeaks` function to detect peaks and obtain their positions `locations`.
* Use the `fitgmdist` function to fit a Gaussian distribution, where the `MaxIter` parameter sets the maximum number of iterations.
* Plot the original signal and the fitted Gaussian distribution in the graph.
# 6. Extensions and Optimization of Gaussian Fitting
### 6.1 Multipeak Gaussian Fitting
#### 6.1.1 Model of Multipeak Gaussian Distribution
The multipeak Gaussian distribution is a Gaussian distribution with multiple peaks. Its probability density function is:
```
p(x) = 1/(2πσ^2)^n/2 * exp(-1/2(x-μ)^TΣ^-1(x-μ))
```
Where:
* n is the data dimension.
* μ is the mean vector.
* Σ is the covariance matrix.
For a multipeak Gaussian distribution, μ and Σ represent the centers and covariances of each peak, respectively.
#### 6.1.2 Implementation of Multipeak Gaussian Fitting in MATLAB
The `fitgmdist` function can be used to perform multipeak Gaussian fitting in MATLAB. The syntax for this function is:
```
gm = fitgmdist(data, k, 'RegularizationValue', lambda)
```
Where:
* `data` is the input data.
* `k` is the number of peaks.
* `RegularizationValue` is the regularization parameter, which helps prevent overfitting.
The following code example demonstrates how to use the `fitgmdist` function for multipeak Gaussian fitting:
```
% Generate multipeak Gaussian distribution data
data = [randn(100, 2) + [2, 2]; randn(100, 2) + [-2, -2]];
% Fit the multipeak Gaussian model
gm = fitgmdist(data, 2, 'RegularizationValue', 0.01);
% Retrieve fitting parameters
mu = gm.mu;
Sigma = gm.Sigma;
% Visualize the fitting results
figure;
scatter(data(:, 1), data(:, 2));
hold on;
ezcontour(@(x, y)mvnpdf([x, y], mu(1, :), Sigma(:,:,1)), [-5, 5], [-5, 5]);
ezcontour(@(x, y)mvnpdf([x, y], mu(2, :), Sigma(:,:,2)), [-5, 5], [-5, 5]);
legend('Data', 'Component 1', 'Component 2');
xlabel('x');
ylabel('y');
title('Multi-Peak Gaussian Fit');
```
### 6.2 Application of Optimization Algorithms in Gaussian Fitting
#### 6.2.1 Principles of Optimization Algorithms
Optimization algorithms are used to find the minimum or maximum values of a function. In Gaussian fitting, ***
***mon optimization algorithms include:
* Gradient Descent Method
* Conjugate Gradient Method
* Newton's Method
#### 6.2.2 Using Optimization Algorithms for Gaussian Fitting in MATLAB
The `fminunc` function can be used for optimization in MATLAB. The syntax for this function is:
```
[x, fval] = fminunc(fun, x0, options)
```
Where:
* `fun` is the objective function.
* `x0` is the initial parameter value.
* `options` are the optimization options.
The following code example demonstrates how to use the `fminunc` function to optimize the Gaussian fitting model:
```
% Define the objective function
fun = @(x) sum((data - x(1) * exp(-(data - x(2))^2 / (2 * x(3)^2))).^2);
% Initial parameter values
x0 = [1, 0, 1];
% Optimize parameters
options = optimset('Display', 'iter');
[x, fval] = fminunc(fun, x0, options);
% Retrieve fitting parameters
a = x(1);
b = x(2);
c = x(3);
% Visualize the fitting results
figure;
scatter(data, a * exp(-(data - b)^2 / (2 * c^2)));
xlabel('x');
ylabel('y');
title('Gaussian Fit with Optimization');
```
0
0