Unveiling the Application of MATLAB Gaussian Fitting in Data Analysis: Uncovering Data Intrinsic Patterns to Assist Decision Making
发布时间: 2024-09-14 19:30:17 阅读量: 30 订阅数: 35 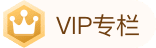
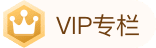
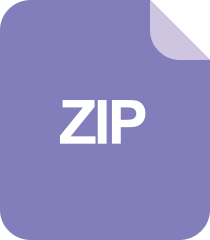
《COMSOL顺层钻孔瓦斯抽采实践案例分析与技术探讨》,COMSOL模拟技术在顺层钻孔瓦斯抽采案例中的应用研究与实践,comsol顺层钻孔瓦斯抽采案例 ,comsol;顺层钻孔;瓦斯抽采;案例,COM
# 1. Overview of MATLAB Gaussian Fitting
Gaussian fitting is a powerful statistical technique used to fit data points to a Gaussian distribution curve. In MATLAB, Gaussian fitting provides an effective method for modeling, analyzing, and predicting data.
The Gaussian distribution, also known as the normal distribution, is a bell-shaped curve defined by two parameters: the mean and the standard deviation. Gaussian fitting involves finding a set of parameters that bring the Gaussian distribution curve closest to a given set of data points.
Through Gaussian fitting, key features can be extracted from the data, such as central tendency, dispersion, and peak location. This makes Gaussian fitting a valuable tool for data analysis, pattern recognition, and predictive modeling.
# 2. Theoretical Foundations of MATLAB Gaussian Fitting
### 2.1 Mathematical Model of the Gaussian Distribution
The Gaussian distribution, also known as the normal distribution, is a continuous probability distribution whose probability density function is defined as:
```
f(x) = (1 / (σ√(2π))) * e^(-(x - μ)² / (2σ²))
```
Where:
* μ: the mean, indicating the central position of the distribution
* σ: the standard deviation, indicating the degree of dispersion of the distribution
The Gaussian distribution has the following characteristics:
* Symmetry: The distribution is symmetrical on both sides of the mean
* Bell-shaped curve: The probability density function takes the shape of a bell curve
* 95% probability interval: Within the mean ±2σ range, 95% of the probability is included
* Asymptotic behavior: As the number of samples increases, the distribution of sample means approaches the Gaussian distribution
### 2.2 Principles and Methods of Gaussian Fitting
Gaussian fitting is a statistical modeling technique aimed at finding a set of parameters (μ, σ) to make the probability density function of a given dataset as close as possible to the probability density function of the Gaussian distribution.
The fitting process involves the following steps:
**1. Data Preprocessing**
* Remove outliers and noise
* Normalize or standardize data
**2. Parameter Estimation**
***Maximum Likelihood Estimation (MLE):** Parameters are estimated by maximizing the likelihood function of the given dataset
***Least Squares Method:** Parameters are estimated by minimizing the squared error between the fitted curve and the data points
**3. Model Evaluation**
***Residual Analysis:** Check if the fitted residuals are randomly distributed to assess the goodness of fit
***Goodness of Fit Metrics:** Use metrics like R², AIC, or BIC to quantify the goodness of fit
**4. Parameter Interpretation**
* Mean (μ): Indicates the central location of the data
* Standard Deviation (σ): Indicates the degree of dispersion of the data
**5. Applications**
* Data feature extraction and pattern recognition
* Trend prediction and anomaly detection
* Parameter estimation and uncertainty analysis
# 3.1 Data Import and Preprocessing
Before performing Gaussian fitting, data needs to be imported into MATLAB and preprocessed. Data import can be done using the `importdata` function, which can import data from various file formats.
```
data = importdata('data.csv');
```
After importing the data, preprocessing is required to ensure the data is suitable for Gaussian fitting. Preprocessing typically includes:
***Data Cleaning:** Deleting or replacing outliers and missing values.
***Data Standardization:** Converting data to a standard normal distribution with a mean of 0 and a standard deviation of 1.
***Data Transformation:** If the data does not conform to a normal distribution, transformations such as logarithmic or square root can be used to make it closer to a normal distribution.
### 3.2 Establishing the Gaussian Fitting Model
The Gaussian fitting model can be established using MATLAB's `fitgmdist` function. This function
0
0
相关推荐





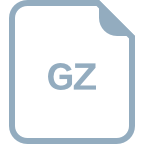
