MATLAB Curve Interpolation: Predicting Unknown Points and Expanding Data Scope
发布时间: 2024-09-14 08:28:03 阅读量: 29 订阅数: 33 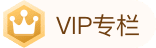
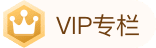
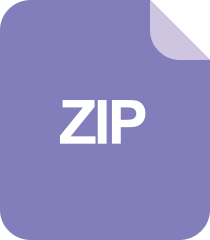
Cubic Convolution Interpolation:三次卷积插值-matlab开发
# 1. Overview of MATLAB Curve Interpolation**
Curve interpolation is an approximation technique that creates smooth curves through known data points. In MATLAB, curve interpolation is used in various applications including data visualization, prediction, and data processing.
Curve interpolation algorithms calculate the value of new points based on known data points. MATLAB provides a range of curve interpolation functions, including linear interpolation, polynomial interpolation, and spline interpolation. These functions allow users to specify the interpolation method, data points, and interpolation points.
# 2. Theoretical Foundation of Curve Interpolation
### 2.1 Linear Interpolation
Linear interpolation is the simplest interpolation method, which assumes that the function values between adjacent data points change linearly. Given a set of data points $(x_0, y_0), (x_1, y_1), \dots, (x_n, y_n)$, where $x_0 < x_1 < \dots < x_n$, the interpolation function within the interval $[x_i, x_{i+1}]$ is:
```
f(x) = y_i + (x - x_i) * (y_{i+1} - y_i) / (x_{i+1} - x_i)
```
**Code Block:**
```matlab
% Given data points
x = [0, 1, 2, 3];
y = [0, 1, 4, 9];
% Linearly interpolate within the interval [1.5, 2.5]
xi = 1.5:0.1:2.5;
yi = interp1(x, y, xi, 'linear');
% Plot the interpolation curve
plot(x, y, 'o', xi, yi, '-');
xlabel('x');
ylabel('y');
legend('Data Points', 'Interpolation Curve');
```
**Logical Analysis:**
* The `interp1` function is used for linear interpolation, with parameters being: data point x-coordinates `x`, data point y-coordinates `y`, interpolation point x-coordinates `xi`, and interpolation method `'linear'`.
* The `plot` function is used to draw the interpolation curve, where `'o'` represents data points and `'-'` represents the interpolation curve.
* The `xlabel` and `ylabel` functions are used to set the axis labels.
* The `legend` function is used to add a legend.
### 2.2 Polynomial Interpolation
Polynomial interpolation assumes that the function values between adjacent data points can be approximated by a polynomial. Given a set of data points $(x_0, y_0), (x_1, y_1), \dots, (x_n, y_n)$, where $x_0 < x_1 < \dots < x_n$, the n-th degree polynomial interpolation function is:
```
f(x) = a_0 + a_1(x - x_0) + a_2(x - x_0)(x - x_1) + \dots + a_n(x - x_0)(x - x_1) \dots (x - x_{n-1})
```
where $a_0, a_1, \dots, a_n$ are the polynomial coefficients.
**Code Block:**
```matlab
% Given data points
x = [0, 1, 2, 3];
y = [0, 1, 4, 9];
% Perform 3rd degree polynomial interpolation within the interval [1.5, 2.5]
xi = 1.5:0.1:2.5;
yi = interp1(x, y, xi, 'spline');
% Plot the interpolation curve
plot(x, y, 'o', xi, yi, '-');
xlabel('x');
ylabel('y');
legend('Data Points', 'Interpolation Curve');
```
**Logical Analysis:**
* The `interp1` function is used for polynomial interpolation, with parameters similar to linear interpolation, but the interpolation method is changed to `'spline'`.
* The `spline` method uses piecewise cubic spline interpolation, which generates smooth curves between adjacent data points.
### 2.3 Spline Interpolation
Spline interpolation is a piecewise polynomial interpolation method that generates smooth curves between adjacent data points while ensuring the interpolation function is continuously differentiable throughout the interval. The spline interpolation function consists of multiple piecewise polynomials, each valid within its own interval.
**Code Block:**
```matlab
% Given data points
x = [0, 1, 2, 3];
y = [0, 1, 4, 9];
% Perform spline interpolation within the interval [1.5, 2.5]
xi = 1.5:0.1:2.5;
yi = interp1(x, y, xi, 'spline');
% Plot the interpolation curve
plot(x, y, 'o', xi, yi, '-');
xlabel('x');
ylabel('y');
legend('Data Points', 'Interpolation Curve');
```
**Logical Analysis:**
* The `interp1` function is used for spline interpolation, with parameters similar to polynomial interpolation, but the interpolation method is changed to `'spline'`.
* The `spline` method uses piecewise cubic spline interpolation, which generates smooth curves between adjacent data points.
# 3.1 Linear Interpolation Function interp1()
Linear interpolation is the simplest interpolation method, which estimates the value of unknown points by connecting the straight lines between adjacent data points. The `interp1()` function in MATLAB is used to perform linear interpolation.
#### Parameter Explanation
The syntax of the `interp1()` function is as follows:
```matlab
y = interp1(x, y, xi, method)
```
where:
* `x`: x-coor
0
0
相关推荐







