【MATLAB Curve Drawing Guide】: From Beginner to Expert, Creating Professional-Level Charts
发布时间: 2024-09-14 08:13:22 阅读量: 23 订阅数: 22 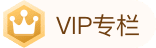
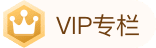
# **MATLAB Curve Plotting Guide**: From Novice to Expert, Crafting Professional Charts
MATLAB is a powerful technical computing language widely used in the fields of science, engineering, and finance. Curve plotting is an essential function in MATLAB that visualizes data, aiding users in analyzing and understanding it.
This chapter introduces the basics of MATLAB curve plotting, including the syntax, functions, styles, and color settings for curve plotting in MATLAB, as well as the usage of legends and labels. With these basics, users can quickly get started with MATLAB curve plotting and create clear and aesthetically pleasing graphs.
# 2. Theory and Practice of Curve Plotting
### 2.1 Mathematical Principles of Curve Plotting
#### 2.1.1 Functions and Equations
A curve is a term in mathematics that denotes the graphical representation of a function. A function is a rule that maps an input (independent variable) to an output (dependent variable). Functions can be represented by algebraic equations, such as:
```
y = f(x)
```
Here, `y` is the dependent variable, `x` is the independent variable, and `f` is the function.
#### 2.1.2 Coordinate Systems and Transformations
Curve plotting is typically performed in the Cartesian coordinate system, which consists of two perpendicular axes (the x-axis and the y-axis). Each point is represented by its x and y coordinates.
Coor***mon coordinate transformations include translation, rotation, and scaling.
### 2.2 MATLAB Curve Plotting Syntax and Functions
#### 2.2.1 plot() and Related Functions
MATLAB offers various functions for plotting curves, with the most basic being the `plot()` function. The syntax for `plot()` is:
```
plot(x, y)
```
Here, `x` and `y` are vectors containing the x and y coordinates.
In addition to the `plot()` function, MATLAB also provides other functions for plotting special types of curves, such as:
- `stem()`: Plots a stem graph
- `bar()`: Plots a bar graph
- `scatter()`: Plots a scatter plot
#### 2.2.2 Style and Color Settings
MATLAB allows users to customize the style and color of curves. The following properties can be used to set the style and color:
- `LineStyle`: Sets the line style of the curve, such as solid, dashed, or dash-dot
- `LineWidth`: Sets the width of the curve
- `Color`: Sets the color of the curve
For example, the following code plots a red dashed line:
```
plot(x, y, 'r--')
```
#### 2.2.3 Legends and Labels
Legends and labels are crucial for explaining curves. MATLAB provides the following functions to add legends and labels:
- `legend()`: Adds a legend
- `xlabel()`: Sets the x-axis label
- `ylabel()`: Sets the y-axis label
- `title()`: Sets the graph's title
For example, the following code adds a legend and axis labels:
```
plot(x, y, 'r--')
legend('Curve 1')
xlabel('x')
ylabel('y')
title('Example of Curve Plotting')
```
# 3. Advanced Techniques for Curve Plotting
### 3.1 Data Processing and Preprocessing
#### 3.1.1 Data Import and Export
MATLAB offers various methods for importing and exporting data, including:
- The `load()` and `save()` functions: Used for importing and exporting data from files.
- The `importdata()` and `exportdata()` functions: Used for importing and exporting data from various file formats.
- The `readtable()` and `writetable()` functions: Used for importing and exporting data from tabular data formats.
**Code block:**
```matlab
% Import data from a CSV file
data = importdata('data.csv');
% Export data to a MAT file
save('data.mat', 'data');
% Import data from tabular data
data = readtable('data.xlsx');
% Export data to a text file
writetable(data, 'data.txt');
```
**Logical Analysis:**
- The `importdata()` function reads the specified file and returns a data structure containing the data.
- The `save()` function saves the specified variable to a MAT file.
- The `readtable()` function imports data from tabular data formats (such as Excel files) and returns a table object.
- The `writetable()` function exports a table object to a text file or other supported formats.
#### 3.1.2 Data Filtering and Interpolation
Data filtering and interpolation techniques are used to handle missing values, outliers, and irregularly sampled data.
***Data Filtering:**
- `movmean()` function: Used for smoothing data.
- `medfilt1()` function: Used for noise removal.
- `butter()` function: Used for designing and applying filters.
***Data Interpolation:**
- `interp1()` function: Used for linear interpolation.
- `interp2()` function: Used for two-dimensional interpolation.
- `griddata()` function: Used for interpolation based on grid data.
**Code block:**
```matlab
% Smooth data
smoothed_data = movmean(data, 5);
% Remove noise
filtered_data = medfilt1(data, 5);
% Linear interpolation
interpolated_data = interp1(x, y, new_x);
```
**Logical Analysis:**
- The `movmean()` function calculates the moving average within a specified window, smoothing the data.
- The `medfilt1()` function uses a median filter to remove noise while preserving the main features of the data.
- The `interp1()` function performs one-dimensional linear interpolation, generating new data points.
### 3.2 Multiple Curves and Subplots
#### 3.2.1 Multiple Curve Plotting
MATLAB allows plotting multiple curves on the same graph to compare different datasets or to show relationships between different variables.
* The `hold on` and `hold off` commands: Used for plotting multiple curves on the same graph.
* The `legend()` function: Used for adding legends to identify each curve.
**Code block:**
```matlab
% Plot multiple curves
figure;
hold on;
plot(x1, y1, 'r');
plot(x2, y2, 'b');
hold off;
% Add legends
legend('Curve 1', 'Curve 2');
```
**Logical Analysis:**
- The `hold on` command allows plotting multiple curves on the same graph.
- The `plot()` function draws each curve, specifying the color and line type.
- The `hold off` command disables the mode for plotting multiple curves.
- The `legend()` function adds a legend displaying the labels for each curve.
#### 3.2.2 Subplot Layout and Management
MATLAB provides functionality for creating subplot layouts, allowing multiple subplots to be displayed within a single figure window.
* The `subplot()` function: Used for creating subplots.
* The `title()` function: Used for setting the title of a subplot.
* The `xlabel()` and `ylabel()` functions: Used for setting the x and y-axis labels of a subplot.
**Code block:**
```matlab
% Create subplot layout
figure;
subplot(2, 1, 1);
plot(x, y1);
title('Subplot 1');
xlabel('x');
ylabel('y1');
subplot(2, 1, 2);
plot(x, y2);
title('Subplot 2');
xlabel('x');
ylabel('y2');
```
**Logical Analysis:**
- The `subplot(2, 1, 1)` command creates a layout with two subplots, with the first subplot at the top.
- The `plot()` function is used to draw the data.
- The `title()`, `xlabel()`, and `ylabel()` functions set the titles and axis labels for the subplots.
### 3.3 Interactive Curve Plotting
#### 3.3.1 Data Point Selection and Modification
MATLAB allows users to interactively select and modify data points.
* The `ginput()` function: Used for obtaining points selected by the user.
* The `datacursormode()` function: Used for enabling data cursor mode, which displays information about data points.
**Code block:**
```matlab
% Get points selected by the user
points = ginput(2);
% Modify data points
data(points(1, 1), points(1, 2)) = 10;
```
**Logical Analysis:**
- The `ginput()` function obtains points selected by the user and returns a matrix containing the x and y coordinates.
- Data points can be modified by directly indexing the data array.
#### 3.3.2 Graph Zooming and Rotation
MATLAB provides interactive zooming and rotating functionality for graphs.
* The `zoom()` function: Used for zooming in and out of a graph.
* The `rotate3d()` function: Used for rotating three-dimensional graphs.
**Code block:**
```matlab
% Zoom in on a graph
zoom on;
% Rotate a three-dimensional graph
rotate3d on;
```
**Logical Analysis:**
- `zoom on` enables zoom mode, allowing users to zoom in and out of a graph using a mouse.
- `rotate3d on` enables rotate mode, allowing users to rotate three-dimensional graphs using a mouse.
# 4. Applications of Curve Plotting
### 4.1 Scientific Data Visualization
#### 4.1.1 Experimental Data Analysis
Curve plotting is essential in scientific data visualization as it allows researchers to graphically represent and analyze experimental data. By plotting data points, trends, patterns, and outliers can be identified.
For example, in a physics experiment, curves can be used to plot the velocity or acceleration of an object over time. Analyzing these curves can determine the object's state of motion, such as constant velocity, acceleration, or deceleration.
```matlab
% Experimental data: time and speed
time = [0, 1, 2, 3, 4, 5];
speed = [0, 10, 20, 30, 40, 50];
% Plot the curve
plot(time, speed, 'b-o');
xlabel('Time (s)');
ylabel('Speed (m/s)');
title('Object Motion Speed vs. Time Curve');
grid on;
% Analyze the curve
% Speed increases linearly with time
disp('Curve analysis: Speed increases linearly with time.');
```
#### 4.1.2 Model Fitting and Prediction
Curve plotting is also used for model fitting and prediction. By fitting experimental data to a mathematical model, the underlying relationships can be inferred, and future behavior can be predicted.
For instance, in a chemical reaction, curves can be used to plot the concentration of reactants over time. By fitting the data to an exponential decay model, the reaction rate constant can be determined, and the time for the reaction to complete can be predicted.
```matlab
% Experimental data: time and reactant concentration
time = [0, 10, 20, 30, 40, 50];
concentration = [100, 80, 60, 40, 20, 10];
% Fit to an exponential decay model
model = fit(time', concentration', 'exp1');
% Plot the fitted curve
plot(time, concentration, 'b-o');
hold on;
plot(time, model(time), 'r--');
xlabel('Time (s)');
ylabel('Concentration (%)');
title('Reactant Concentration vs. Time Curve');
legend('Experimental Data', 'Fitted Curve');
grid on;
% Analyze the curve
% Reactant concentration decreases exponentially over time
disp('Curve analysis: Reactant concentration decreases exponentially over time.');
```
### 4.2 Engineering Design and Simulation
#### 4.2.1 Mechanics and Thermodynamics Curves
Curve plotting is widely used in engineering design and simulation to represent mechanical and thermodynamic behaviors. For example, in mechanical engineering, curves can be used to plot stress-strain curves to determine the strength and elasticity of materials.
```matlab
% Stress-strain curve data
stress = [0, 100, 200, 300, 400, 500];
strain = [0, 0.002, 0.004, 0.006, 0.008, 0.01];
% Plot the curve
plot(stress, strain, 'g-o');
xlabel('Stress (MPa)');
ylabel('Strain');
title('Stress-Strain Curve');
grid on;
% Analyze the curve
% In the elastic deformation phase, stress and strain are linearly related
disp('Curve analysis: In the elastic deformation phase, stress and strain are linearly related.');
```
#### 4.2.2 Signal Processing and Control Systems
In signal processing and control systems, curve plotting is used to represent signals and system behaviors. For example, in communication systems, curves can be used to plot the spectrum of a modulated signal.
```matlab
% Modulated signal data
t = linspace(0, 1, 1000);
carrier = 100 * cos(2 * pi * 1000 * t);
modulatedSignal = carrier .* sin(2 * pi * 100 * t);
% Plot the spectrum
Fs = 1000; % Sampling frequency
N = length(modulatedSignal); % Number of data points
Y = fft(modulatedSignal);
f = (0:N-1) * (Fs/N); % Frequency vector
figure;
plot(f, abs(Y), 'r-');
xlabel('Frequency (Hz)');
ylabel('Amplitude');
title('Modulated Signal Spectrum');
grid on;
% Analyze the curve
% The modulated signal has a distinct spectral peak at 100Hz
disp('Curve analysis: The modulated signal has a distinct spectral peak at 100Hz.');
```
### 4.3 Finance and Economic Analysis
#### 4.3.1 Stock Price Trends
Curve plotting is crucial in finance and economic analysis for representing stock price trends and other economic indicators. By analyzing curves, trends can be identified, future trends can be predicted, and investment decisions can be made.
```matlab
% Stock price data
date = {'2023-01-01', '2023-02-01', '2023-03-01', '2023-04-01', '2023-05-01'};
price = [100, 110, 120, 130, 140];
% Plot a line graph
figure;
plot(date, price, 'b-o');
xlabel('Date');
ylabel('Stock Price');
title('Stock Price Trend');
grid on;
% Analyze the curve
% The stock price shows a steady upward trend
disp('Curve analysis: The stock price shows a steady upward trend.');
```
#### 4.3.2 Economic Indicator Charts
Curve plotting is also used to represent economic indicators such as unemployment rates, inflation rates, and GDP. By analyzing these curves, the economic situation can be understood, and policy decisions can be made.
```matlab
% Economic indicator data
year = [2018, 2019, 2020, 2021, 2022];
unemploymentRate = [4.1, 3.5, 6.2, 5.4, 3.9];
inflationRate = [1.9, 2.3, 1.2, 2.9, 4.7];
gdpGrowth = [2.9, 2.3, -3.5, 5.7, 2.6];
% Plot line graphs
figure;
subplot(3, 1, 1);
plot(year, unemploymentRate, 'r-o');
xlabel('Year');
ylabel('Unemployment Rate (%)');
title('Unemployment Rate Trend');
grid on;
subplot(3, 1, 2);
plot(year, inflationRate, 'g-o');
xlabel('Year');
ylabel('Inflation Rate (%)');
title('Inflation Rate Trend');
grid on;
subplot(3, 1, 3);
plot(year, gdpGrowth, 'b-o');
xlabel('Year');
ylabel('GDP Growth Rate (%)');
title('GDP Growth Rate Trend');
grid on;
% Analyze the curves
% The unemployment rate spiked significantly in 2020 and then decreased
% The inflation rate spiked significantly in 2022
% The GDP growth rate dropped significantly in 2020 and then rebounded
disp('Curve analysis:');
disp(' - The unemployment rate spiked significantly in 2020 and then decreased.');
disp(' - The inflation rate spiked significantly in 2022.');
disp(' - The GDP growth rate dropped significantly in 2020 and then rebounded.');
```
# 5. Best Practices for MATLAB Curve Plotting**
**5.1 Code Optimization and Readability**
To ensure the efficiency and maintainability of MATLAB curve plotting code, it is essential to follow best practices.
**5.1.1 Use of Functions and Scripts**
Organizing code into functions and scripts can improve readability, reusability, and maintainability. Functions can encapsulate specific tasks, while scripts can contain a series of commands to perform more complex analyses. For example:
```matlab
% Define a function to plot a sine curve
function plot_sine(amplitude, frequency, phase)
t = linspace(0, 2*pi, 100);
y = amplitude * sin(frequency * t + phase);
plot(t, y);
end
% Call this function in a script
amplitude = 1;
frequency = 2;
phase = pi/2;
plot_sine(amplitude, frequency, phase);
```
**5.1.2 Comments and Documentation**
***ments explain the purpose and behavior of the code, while documentation provides more comprehensive information, such as the input and output parameters of a function.
```matlab
% Plot a sine curve
%
% Inputs:
% amplitude: The amplitude of the curve
% frequency: The frequency of the curve
% phase: The phase of the curve
%
% Outputs:
% None
function plot_sine(amplitude, frequency, phase)
% ...
end
```
**5.2 Principles of Graphic Design**
In addition to code optimization, following principles of graphic design is crucial for creating clear and effective charts.
**5.2.1 Color Selection and Contrast**
Choosing colors with high contrast improves the readability of a chart. Avoid using similar colors or light colors, as they may be difficult to distinguish.
**5.2.2 Graphic Layout and Clarity**
Carefully arranging chart elements, such as titles, labels, and legends, can improve the comprehensibility of the chart. Ensure that the chart has sufficient whitespace to avoid clutter and confusion.
0
0
相关推荐
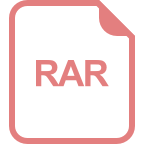
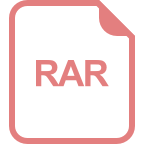
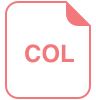
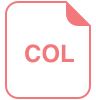
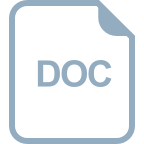
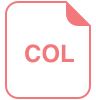
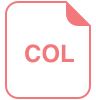
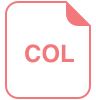