[In-depth Analysis of ORM]: Mastering the Art of Interaction Between SQLAlchemy and MySQL
发布时间: 2024-09-12 14:41:48 阅读量: 34 订阅数: 38 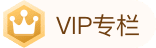
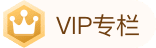
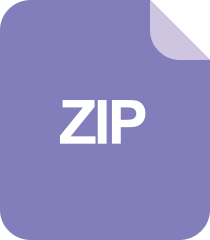
基于Flask的RESTful API实战代码:集成Flask-SQLAlchemy与MySQL
# [ORM In-depth Analysis]: Mastering the Art of Interacting with MySQL Using SQLAlchemy
## Brief Introduction to ORM Concepts and Getting Started with SQLAlchemy
### A Brief Overview of ORM Concepts
**Object-Relational Mapping (ORM)** is a technique that automates the transformation of data between relational databases and objects. It enables developers to manipulate databases using an object-oriented programming paradigm, without the need to write SQL code directly. This approach reduces the amount of code, enhancing development efficiency.
### What is SQL?
**Structured Query Language (SQL)** is the standard programming language used to access and manipulate relational databases. It consists of a series of statements that can execute operations to create, query, update, and delete data within a database.
### Getting Started with SQLAlchemy
**SQLAlchemy** is one of the most popular ORM tools in Python. It provides a rich API that allows developers to interact with databases using Pythonic code, while avoiding the complexity of writing raw SQL statements.
#### Installing SQLAlchemy
First, you need to install the SQLAlchemy library, which can be done using the following command:
```bash
pip install sqlalchemy
```
#### Basic Usage
Here is a simple example of how to use SQLAlchemy to create and operate on database tables:
```python
from sqlalchemy import create_engine, Column, Integer, String
from sqlalchemy.ext.declarative import declarative_base
from sqlalchemy.orm import sessionmaker
# Define the base class
Base = declarative_base()
# Define a model class
class User(Base):
__tablename__ = 'users'
id = Column(Integer, primary_key=True)
name = Column(String)
age = Column(Integer)
# Create a database engine
engine = create_engine('sqlite:///example.db')
# Create tables
Base.metadata.create_all(engine)
# Create a session class
Session = sessionmaker(bind=engine)
session = Session()
# Add data
session.add(User(name='Alice', age=25))
***mit()
# Query data
users = session.query(User).filter_by(name='Alice').all()
for user in users:
print(user.name, user.age)
```
This code demonstrates the basic steps of defining a model class, creating database tables, adding data, and querying data. As we delve deeper into SQLAlchemy, we will cover more advanced features to optimize data operations.
# 2. Detailed Explanation of Core Components of SQLAlchemy
## 2.1 Database Connection and Engine Configuration
### 2.1.1 Creating a Database Engine
Before using SQLAlchemy for database operations, the first step is to create a database engine. The database engine is one of the core concepts of SQLAlchemy, serving as the interface for communicating with the database, managing connection pools, and executing SQL statements.
```python
from sqlalchemy import create_engine
# Create an in-memory SQLite database engine
engine = create_engine('sqlite:///:memory:')
```
The above code creates an engine instance for an in-memory SQLite database. SQLAlchemy supports various databases, including PostgreSQL, MySQL, Oracle, etc. The type of database can be specified by modifying the URL.
**Parameter Explanation**:
- `'sqlite:///:memory:'`: Connection string to an in-memory SQLite database. If a database file path is provided as a URI parameter, a persistent database file will be created.
- `create_engine`: A function provided by SQLAlchemy to create an engine instance.
**Logical Analysis**:
- The engine instance is used to manage connection pools, optimizing database access.
- The engine can be used to build sessions, which are the context environment for executing database operations.
### 2.1.2 Connection Pooling Mechanism
Connection pooling is SQLAlchemy's mechanism for managing database connections, which can significantly improve the performance of database operations. Connection pooling is enabled by default when the engine is created.
```python
# Assuming we have a MySQL database engine
engine = create_engine('mysql+pymysql://user:password@localhost/dbname', pool_size=5)
# Use the engine to create a session
with engine.connect() as connection:
result = connection.execute('SELECT * FROM table')
for row in result:
print(row)
```
**Parameter Explanation**:
- `pool_size=5`: Specifies the number of connections that can be cached in the connection pool.
**Logical Analysis**:
- When a session is created, the engine retrieves a connection from the connection pool.
- If there are no available connections in the pool, a new database connection is opened.
- After the operation is completed, the connection is returned to the connection pool for future use.
**Scalability Explanation**:
- SQLAlchemy's connection pool supports various configuration parameters, such as timeout, maximum number of connections, etc., to meet different performance and resource constraints.
## 2.2 ORM Model Mapping
### 2.2.1 Basic Model Definition
Using SQLAlchemy to define models means creating a class that will serve as the mapping for a database table. These classes usually inherit from `Base`, which is SQLAlchemy's metadata container.
```python
from sqlalchemy.ext.declarative import declarative_base
from sqlalchemy import Column, Integer, String
Base = declarative_base()
class User(Base):
__tablename__ = 'users'
id = Column(Integer, primary_key=True)
name = Column(String)
fullname = Column(String)
nickname = Column(String)
```
**Parameter Explanation**:
- `__tablename__`: The name of the table in the database.
- `Column`: Defines a column in the table. You can specify the data type and constraints of the column.
**Logical Analysis**:
- `declarative_base` provides a base class for models, and all models inherit from this base class.
- Each class attribute corresponds to a column in the table.
**Scalability Explanation**:
- The class-based model definition is very flexible, allowing for custom validators and constructors.
- Inheritance can be used to create complex model hierarchies with shared fields.
### 2.2.2 Configuration of Attributes and Columns
When defining ORM models, attributes and columns can be configured in detail to meet the requirements of data integrity.
```python
from sqlalchemy import ForeignKey
from sqlalchemy.orm import relationship
class Address(Base):
__tablename__ = 'addresses'
id = Column(Integer, primary_key=True)
email_address = Column(String, nullable=False)
user_id = Column(Integer, ForeignKey('users.id'))
user = relationship("User", back_populates="addresses")
class User(Base):
__tablename__ = 'users'
id = Column(Integer, primary_key=True)
name = Column(String)
fullname = Column(String)
nickname = Column(String)
addresses = relationship("Address", back_populates="user")
Base.metadata.create_all(engine)
```
**Parameter Explanation**:
- `ForeignKey`: Indicates that a column is a foreign key, referencing the primary key of another table.
- `relationship`: Creates a relationship between objects.
**Logical Analysis**:
- `relationship` is used to set up relationships between two tables, and `back_populates` is used to automatically create bidirectional relationships.
- `nullable=False` indicates that the column does not allow null values, enforcing data integrity at the database level.
**Scalability Explanation**:
- `relationship` and `ForeignKey` facilitate object-relational mapping.
- By configuring these attributes and columns, complex data associations and integrity constraints can be established.
### 2.2.3 Relationship Mapping
In ORM, relationship mapping is a straightforward expression of table relationships. In SQLAlchemy, it can be one-way or bidirectional.
```python
from sqlalchemy.orm import sessionmaker
Session = sessionmaker(bind=engine)
session = Session()
```
**Logical Analysis**:
- `sessionmaker` creates a session factory that can be used to generate sessions.
- The session is a bridge between the database and ORM models, through which CRUD operations can be performed.
**Scalability Explanation**:
- SQLAlchemy supports one-to-many, many-to-one, one-to-one, and many-to-many relationship mappings.
- Additional parameters can control the loading behavior of relationships, such as lazy loading.
## 2.3 SQLAlchemy Query Language
### 2.3.1 Basic Query Construction
SQLAlchemy provides a query interface similar to SQL, making database queries very intuitive in Python.
```python
from sqlalchemy import select
# Create a query object
query = select([User]).where(User.name == 'John Doe')
# Execute the query and get the results
with engine.connect() as conn:
result = conn.execute(query)
for row in result:
print(row)
```
**Parameter Explanation**:
- `select([User])`: Creates a selection query object, specifying the table to query.
- `where(User.name == 'John Doe')`: Adds a query condition.
**Logical Analysis**:
- Query objects in SQLAlchemy are callable, meaning they can execute and return results.
- In practice, query objects can be built, modified, and combined to construct complex query logic.
**Scalability Explanation**:
- Query objects can be chained together, combining various methods to build complex queries.
- Supports dynamically constructing query conditions, making them ideal for dynamically building reports.
### 2.3.2 Aggregation and Grouping Operations
SQLAlchemy supports aggregation and grouping operations, allowing for convenient complex data statistics.
```python
from sqlalchemy import func
# Build an aggregation query
query = select([func.count(User.id), User.name]).group_by(User.name)
# Execute the query
with engine.connect() as conn:
result = conn.execute(query)
for row in result:
print(row)
```
**Parameter Explanation**:
- `func.count(User.id)`: Uses the `func` module for aggregation calculations, in this case, counting the number of users.
- `group_by(User.name)`: Specifies the grouping criterion.
**Logical Analysis**:
- The `group_by` method is used to specify the grouping criterion, and aggregation functions can be applied to grouped data.
- Aggregation queries typically combine `group_by` with filtering conditions for grouping statistics.
**Scalability Explanation**:
- Aggregation operations support various SQL functions, such as `sum()`, `avg()`, `max()`, `min()`, etc.
- Grouping queries can further be combined with `having` clauses to filter the grouping results.
### 2.3.3 Techniques for Building Complex Queries
SQLAlchemy provides a wealth of methods to build complex queries, including join operations, subqueries, and union queries.
```python
# Create a subquery
subq = session.query(Address.email_address).filter(Address.user_id == User.id).correlate(User).subquery()
# Build a union query
query = session.query(User.name, subq.c.email_address).outerjoin(subq, User.addresses)
# Execute the query and get the results
for name, email in query:
print(name, email)
```
**Parameter Explanation**:
- `filter(Address.user_id == User.id)`: Adds a filter condition to the subquery.
- `correlate(User)`: Causes the subquery to be associated with a specific parent query instance during execution.
- `subquery()`: Converts a selection query into a subquery.
- `outerjoin(subq, User.addresses)`: Creates a left join.
**Logical Analysis**:
- Subqueries can be embedded into other queries, providing support for constructing complex queries.
- Outer joins can include rows from the left table that do not match the right table.
**Scalability Explanation**:
- The `select_from` and `from_joinpoint` methods can be used to specify additional tables or joins within a query.
- Use `union`, `intersect`, and `except_` to merge multiple query result sets.
# 3. Advanced Practices in SQLAlchemy
## 3.1 Session Management and Transaction Control
In the use of ORM, session (Session) management and transaction control are key to ensuring data consistency and integrity. Understanding and correctly using them are crucial for writing robust applications.
### 3.1.1 Lifecycle of a Session
The lifecycle of a session typically includes creation, usage, committing, or rolling back stages. Here is a basic usage example:
```python
from sqlalchemy import create_engine
from sqlalchemy.orm import sessionmaker
# Create a database engine
engine = create_engine('sqlite:///example.db')
Session = sessionmaker(bind=engine)
# Create a session
session = Session()
# Use the session for data operations...
# For example, add a new object
new_user = User(name='Alice', age=25)
session.add(new_user)
# Commit the session, ***
***mit()
# Close the session, release resources
session.close()
```
### 3.1.2 Use of Transactions and Exception Handling
A transaction is a logical unit of database operations, ensuring the atomicity of a series of actions. In SQLAlchemy, transactions can be automatically managed or manually controlled. For example:
```python
from sqlalchemy.exc import SQLAlchemyError
try:
# Start a transaction
with session.begin():
# A series of operations
session.add(new_user)
session.add(another_user)
# ***
***mit()
except SQLAlchemyError as e:
# Roll back the transaction in case of an error
session.rollback()
raise e
```
In exception handling, ensure that transactions can roll back in case of errors, to avoid inconsistent data states caused by partial operations.
## 3.2 Advanced Mapping Techniques
As business complexity increases, model mapping also becomes more complex, requiring us to master some advanced mapping skills.
### 3.2.1 Inheritance Mapping Strategies
In ORM, the inheritance of models can be mapped to several strategies in database tables. The most common are the three-table strategy and the single-table strategy. For example:
```python
from sqlalchemy.ext.declarative import declarative_base
from sqlalchemy import Column, Integer, String, ForeignKey
from sqlalchemy.orm import relationship
Base = declarative_base()
class Person(Base):
__tablename__ = 'person'
id = Column(Integer, primary_key=True)
name = Column(String)
class Engineer(Person):
__tablename__ = 'engineer'
id = Column(Integer, ForeignKey('person.id'), primary_key=True)
company = Column(String)
```
### 3.2.2 Composite Primary Keys and Unique Constraints
Composite primary keys and unique constraints are key elements in ensuring data integrity. In SQLAlchemy, composite primary keys can be defined by declaring them:
```python
class User(Base):
__tablename__ = 'user'
id1 = Column(Integer, primary_key=True)
id2 = Column(Integer, primary_key=True)
email = Column(String, unique=True)
```
### 3.2.3 Mapping of Mixed Objects and Tables
In real-world applications, mixed object mapping can enhance the flexibility of database access, allowing direct manipulation of underlying tables and ordinary models:
```python
class MyTable(Base):
__tablename__ = 'my_table'
id = Column(Integer, primary_key=True)
data = Column(String)
# Using SQLAlchemy's native query method
__mapper_args__ = {
'with_polymorphic': '*',
'polymorphic_identity': 'mytable'
}
```
## 3.3 Performance Optimization and Debugging Techniques
Performance optimization and debugging are important for ensuring stable application operation.
### 3.3.1 Solving the N+1 Query Problem
The N+1 query problem is a common performance issue in ORM. It occurs when loading associated objects, resulting in a large number of SQL queries. Solutions include:
```python
# Using joinedload to load associated objects
from sqlalchemy.orm import joinedload
session.query(User).options(joinedload(User.addresses)).all()
```
### 3.3.2 Query Caching and Loading Strategies
In SQLAlchemy, query caching and lazy loading strategies can be used to optimize performance:
```python
# Using subqueryload to preload associated objects
session.query(User).options(subqueryload(User.addresses)).all()
```
### 3.3.3 Using Logs and Performance Analysis Tools
Using logs and performance analysis tools can help us understand the details of ORM operations, thereby identifying bottlenecks:
```python
# Enable logging for SQLAlchemy
import logging
logging.basicConfig()
logging.getLogger('sqlalchemy.engine').setLevel(***)
```
By utilizing these advanced practices, developers can further improve their application performance and ensure stable operation. The next chapter will combine MySQL database cases to demonstrate how to apply this knowledge in practice.
# 4. Practical Cases Combining MySQL
## 4.1 MySQL Database Features and SQLAlchemy
### 4.1.1 Support for MySQL-Specific Data Types
As a popular open-source relational database management system, MySQL has its unique data types, such as `YEAR`, `DATE`, `TIME`, `DATETIME`, `TIMESTAMP`, `CHAR`, `VARCHAR`, `BLOB`, `TEXT`, `JSON`, etc. These types must be properly handled when using SQLAlchemy to ensure that the application's data model matches the actual storage structure of the MySQL database.
When using SQLAlchemy, we need to specify the corresponding data types when defining the columns (Column) of data models to interface with MySQL's unique data types. For example, if we have a column that needs to store JSON data, we can define it in SQLAlchemy as follows:
```python
from sqlalchemy import Column, JSON
class SomeModel(Base):
__tablename__ = 'some_model'
id = Column(Integer, primary_key=True)
json_data = Column(JSON, nullable=False)
```
In this code, the `JSON` type directly maps to MySQL's `JSON` data type. The advantage of this is that SQLAlchemy will generate SQL statements that are compatible with MySQL's features, ensuring accurate storage and retrieval of data in the database.
### 4.1.2 Selection and Configuration of Storage Engines
MySQL allows users to choose different storage engines for tables, such as `InnoDB` and `MyISAM`. The storage engine determines the operational characteristics of the data table, such as transaction support, row locking, foreign keys, and index types. When using SQLAlchemy, the default storage engine can be specified by configuring database engine parameters.
When creating a SQLAlchemy database engine, the `connect_args` parameter can be used to pass storage engine configurations:
```python
from sqlalchemy import create_engine
engine = create_engine('mysql+pymysql://user:password@localhost/dbname',
connect_args={'init_command': 'SET storage_engine=InnoDB'})
```
In this code, the `init_command` in the `connect_args` dictionary is used to specify the default storage engine as `InnoDB`. This is the initialization command executed after establishing the connection, ensuring that all created tables default to using the `InnoDB` storage engine.
## 4.2 ORM Application in Complex Business Scenarios
### 4.2.1 Compound Condition Queries and Dynamic SQL Construction
In complex business scenarios, performing compound condition queries is common. SQLAlchemy provides a flexible query language and expression language to build dynamic queries.
Suppose we have a user table and an order table, and we need to query order information based on the user's name and the date range of the order. The query can be constructed as follows:
```python
from sqlalchemy.orm import Session
from models import User, Order
session = Session()
query = session.query(Order) \
.join(User, Order.user_id == User.id) \
.filter(User.name == 'John Doe',
Order.order_date.between('2023-01-01', '2023-01-31'))
orders = query.all()
```
In this code, the `join` method is used to connect two tables, and the `filter` method is used to add compound conditions. The `between` method is used to specify the date range query condition.
SQLAlchemy also supports building dynamic SQL queries that can dynamically generate query statements based on different input conditions. For example:
```python
from sqlalchemy.sql import text
name = 'John Doe'
date_range = ('2023-01-01', '2023-01-31')
query = session.query(Order) \
.join(User) \
.filter(text('User.name = :name and Order.order_date between :start and :end')) \
.params(name=name, start=date_range[0], end=date_range[1])
```
Here, the `text` function is used to construct an original SQL snippet, and parameters are dynamically passed through the `params` method. Such dynamic construction methods make queries very flexible, able to adapt to various business scenarios.
### 4.2.2 Handling Large Data Volumes and Batch Operations
For operations on large data volumes, SQLAlchemy provides the ability to batch operations, making it efficient to insert or update large amounts of data in batches. The `yield_per` method can be used to optimize performance when obtaining batch query results.
```python
from sqlalchemy import exc
batch_size = 100
try:
while True:
orders = session.query(Order).limit(batch_size).all()
if not orders:
break
for order in orders:
# Update operation
session.add(order)
***mit()
except exc SQLAlchemyError:
session.rollback()
```
This code uses the `limit` method to batch query data and operate on each batch. The `yield_per` method can replace the `limit` method to optimize performance.
When batching data insertion, the `bulk_insert_mappings` method can be used:
```python
from sqlalchemy import bulk_insert_mappings
orders_data = [
{'user_id': 1, 'amount': 100, 'status': 'pending'},
# ...other order data
]
bulk_insert_mappings(Order, orders_data)
***mit()
```
Here, the `bulk_insert_mappings` method accepts a model and a list of data, then inserts the data into the database all at once, which is more efficient.
### 4.2.3 Data Migration and Version Control
During the development process of an application, the database structure may change. To handle these changes, SQLAlchemy provides the Alembic data migration tool for version control.
Here are the basic steps for using Alembic for data migration:
1. Initialize the migration environment:
```bash
alembic init myapp
```
2. After modifying the model, generate a migration script:
```bash
alembic revision --autogenerate -m 'Add new column to user table'
```
3. Apply the migration:
```bash
alembic upgrade head
```
In this way, the database will be updated to the latest structure, keeping it in sync with the application code.
## 4.3 Security and Best Practices
### 4.3.1 Preventing SQL Injection and Secure Queries
SQL injection is a common security threat, and SQLAlchemy effectively prevents this risk by using parameterized queries. When using SQLAlchemy, parameterized queries should always be used, and user input should be avoided from being directly concatenated into SQL statements.
For example:
```python
from sqlalchemy.sql import select
# A secure query
query = session.query(User).filter(User.name == bindparam('name'))
result = query.params(name='John Doe').all()
```
In this example, the `bindparam` method is used to create a parameter placeholder, and the `params` method is used to pass parameter values securely.
### 4.3.2 ORM Code Architecture and Project Integration
When integrating SQLAlchemy into a project, it should follow architecture patterns such as MVC (Model-View-Controller) or MVVM (Model-View-ViewModel). Models usually correspond to database tables, while views or view models are responsible for presentation logic.
To maintain code clarity and maintainability, it is recommended to use packages (packages) to organize models, views, and controllers, as shown below:
```
project/
│
├── app/
│ ├── models/
│ ├── views/
│ ├── controllers/
│ └── ...
│
├── tests/
│ └── ...
│
└── main.py
```
In this example, the `models` folder contains all database model definitions, the `views` folder contains view layer code, and the `controllers` folder contains business logic control code. This makes the project structure clear and responsibilities distinct.
### 4.3.3 Community Best Practices and Pattern References
In actual development, following community best practices can greatly improve development efficiency and code quality. The SQLAlchemy community provides extensive documentation and examples, such as using the `Declarative` base class to define models.
```python
from sqlalchemy.ext.declarative import declarative_base
Base = declarative_base()
class User(Base):
__tablename__ = 'user'
id = Column(Integer, primary_key=True)
name = Column(String)
# Other fields...
```
By inheriting from `declarative_base()`, table mappings and fields can be quickly defined.
Additionally, you can refer to other developers' shared project structures and coding patterns, such as:
- One-to-Many/Many-to-Many relationship mapping.
- Using `hybrid_property` to implement custom behavior methods.
- Utilizing `Association Table` to map many-to-many relationships.
- Using session hooks and event listeners to handle data change events.
By learning and practicing these best practices, developers can build more robust, maintainable, and scalable ORM applications.
# 5. Future Trends and Development of ORM
## 5.1 Evolution of ORM Technology
As software development continues to evolve, Object-Relational Mapping (ORM) technology is also constantly developing and improving. This section will explore the latest developments in current ORM technology and possible future directions.
### 5.1.1 Comparative Analysis of New-Generation ORM Frameworks
The new generation of ORM frameworks, such as Django ORM, Hibernate 5, Entity Framework Core, etc., have significantly improved in performance, flexibility, and ease of use. They typically have the following characteristics:
- **More Efficient Query Generators**: Modern ORM frameworks provide more complex and efficient query builders, supporting native SQL queries to reduce unnecessary database interactions and improve data retrieval efficiency.
- **Support for Asynchronous Operations**: Many modern ORM frameworks support asynchronous programming models to meet the needs of modern high-concurrency and reactive Web applications.
- **More Complete Metadata and Migration Tools**: For example, Entity Framework Core's Code First migration allows database structures to change with code, maintaining consistency in data models.
- **High Integration**: New-generation ORMs often integrate more closely with other parts of the framework, such as authentication and authorization.
### 5.1.2 Exploring the Integration of ORM and NoSQL
NoSQL databases are gradually becoming an important part of modern application architectures due to their horizontal scalability and flexible data models. As needs diversify, ORM technology is also beginning to explore integration with NoSQL databases.
- **Support for Multiple Databases**: ORM frameworks like SQLAlchemy have started to support multiple databases, including both relational and non-relational databases, providing developers with the ability to operate on various databases through a single query interface.
- **Mapping of Document Storage Models**: The rise of document databases has created a demand for new data structures. ORM frameworks need to adapt to this change, providing mapping solutions from traditional relational tables to document structures.
- **ORM Frameworks as a Data Access Layer**: ORM is not just a mapping tool for relational databases but is gradually becoming a universal data access layer for applications and data storage, whether the storage form is SQL or NoSQL.
## 5.2 Integration of Deep Learning and ORM
Deep learning has begun to impact various aspects of software development, including database query optimization and data processing.
### 5.2.1 Using Machine Learning to Optimize Query Performance
Query optimization is an important part of database management, and deep learning can play a significant role here:
- **Intelligent Query Optimization**: By collecting a large amount of query execution data, machine learning models can learn the optimal query execution plan, automatically optimize SQL queries, and reduce the response time of the database.
- **Predictive Maintenance**: Machine learning can predict database performance bottlenecks and take measures in advance to avoid potential performance degradation.
- **Anomaly Detection**: After learning the normal behavior patterns of the database, the model can detect abnormal patterns and promptly identify potential security threats.
### 5.2.2 The Application of ORM in Data Analysis and Processing
Modern business applications not only need to store data but also need to analyze and process data. ORM frameworks also play a role in data processing:
- **Data Model Analysis**: Deep learning can analyze the usage of data models and provide data support for designing more efficient data models.
- **Automated Reporting and Analysis**: By combining ORM frameworks, information can be automatically extracted from the database and analyzed through machine learning models, automatically generating reports and recommendations.
## 5.3 Community Dynamics and Future Outlook
### 5.3.1 Current Active Projects and Contributors in the Community
The open-source community's contribution to ORM technology is significant. Active projects include:
- **Active Projects**: Projects like Hibernate Validator and Entity Framework Code First Migrations are continuously updated and improved, providing strong tool support for developers.
- **Contributors**: On platforms like GitHub, many developers participate in contributing to ORM frameworks, some focusing on performance optimization, others providing new features.
### 5.3.2 Predicting the Future Development Trends of ORM Technology
Looking ahead, ORM technology development may move in the following directions:
- **Better Integration with Cloud Services**: The popularization of cloud services makes database management more reliant on services provided by cloud platforms. ORM frameworks need to integrate with cloud services, providing native support.
- **No Code/Low Code Development**: To meet the needs of rapid development, ORM may move towards providing higher-level abstractions, allowing developers to build complex data operation logic without writing code.
- **Cross-Platform and Cross-Database Consistency**: As technology converges, developers expect to interact with different types of databases using a unified approach. Future ORM technology will pay more attention to cross-platform and cross-database consistency.
Through this in-depth discussion, we not only look forward to the future trends of ORM technology but also gain insights into the possible combinations with cutting-edge technologies such as deep learning. As technology continues to evolve, we have reason to believe that future ORM frameworks will become more intelligent and efficient, better serving developers and end-users.
0
0
相关推荐
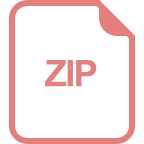
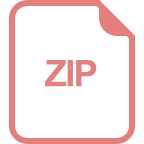
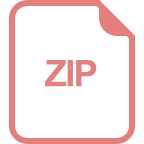
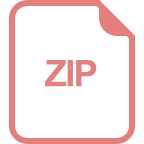
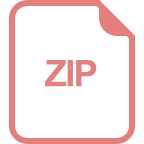
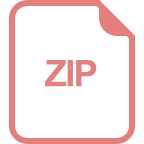
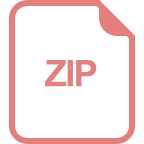
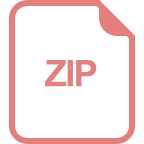