def calculate_birds_eye_view_parameters(x_bounds, y_bounds, z_bounds): """ Parameters ---------- x_bounds: Forward direction in the ego-car. y_bounds: Sides z_bounds: Height Returns ------- bev_resolution: Bird's-eye view bev_resolution bev_start_position Bird's-eye view first element bev_dimension Bird's-eye view tensor spatial dimension """ bev_resolution = torch.tensor([row[2] for row in [x_bounds, y_bounds, z_bounds]]) bev_start_position = torch.tensor([row[0] + row[2] / 2.0 for row in [x_bounds, y_bounds, z_bounds]]) bev_dimension = torch.tensor([(row[1] - row[0]) / row[2] for row in [x_bounds, y_bounds, z_bounds]], dtype=torch.long) return bev_resolution, bev_start_position, bev_dimension这段代码完成的事情
时间: 2024-04-02 11:34:46 浏览: 200
这段代码是定义了一个名为calculate_birds_eye_view_parameters的函数,该函数的目的是计算鸟瞰图的参数。其中,x_bounds、y_bounds和z_bounds分别表示鸟瞰图的前、左、右、上和下边界,bev_resolution表示鸟瞰图的分辨率,bev_start_position表示鸟瞰图的起始位置,bev_dimension表示鸟瞰图的空间维度。具体而言,bev_resolution是一个包含三个元素的张量,分别表示在x、y和z方向上的分辨率;bev_start_position是一个包含三个元素的张量,分别表示在x、y和z方向上的起始位置;bev_dimension是一个包含三个元素的张量,分别表示在x、y和z方向上的鸟瞰图空间维度。
相关问题
(defun screenshot-callback () (let ((x (mouse-x)) (y (mouse-y))) (setf (text-value x-field) x) (setf (text-value y-field) y) (let ((img (screenshot x y))) (setf (image-value img-field) img)))) (defun screenshot (x y) ;; Get the current view and window size (let* ((view (cds-current-view)) (win (cds-view-window view)) (w (window-width win)) (h (window-height win))) ;; Calculate the bounds of the screenshot (let* ((left (- x (* w 0.1))) (right (+ x (* w 0.1))) (top (- y (* h 0.1))) (bottom (+ y (* h 0.1)))) ;; Take the screenshot (cds-take-screenshot view left top right bottom)))))
这段代码是一个用于截图的回调函数。当用户触发截图事件时,该函数会获取当前鼠标的位置,然后计算出需要截取的区域,并使用CDS(Cadence Design Systems)的API来进行截图操作。具体来说,该函数会获取当前视图和窗口的大小,然后根据鼠标位置和窗口大小计算出截图区域的左上角和右下角坐标,最后调用CDS的take-screenshot函数来进行截图操作。截图结果将被显示在界面上。
该代码中给出报错numpy.AxisError: axis 1 is out of bounds for array of dimension 1该如何修改
报错 "numpy.AxisError: axis 1 is out of bounds for array of dimension 1" 是因为在代码的第 27 行使用了 `axis=(1,2)` 参数,但是 `features` 数组的维度不足以支持这个参数。
为了解决这个问题,可以使用以下代码将 `features` 变量的维度从 `(n, c, 1, 1)` 转换为 `(c, 1, 1)`:
```python
# Reshape features to remove single-dimensional axes
features = features.squeeze()
if len(features.shape) == 3:
features = features.unsqueeze(-1)
if len(features.shape) == 2:
features = features.unsqueeze(-1).unsqueeze(-1)
```
这段代码会检查 `features` 数组的维度是否为 `(n, c, 1, 1)`,如果是,会先使用 `squeeze()` 方法删除所有大小为 1 的维度。如果删除后的维度为 `(c, 1, 1)`,则不需要进一步操作。否则,会使用 `unsqueeze()` 方法添加缺少的维度。
这样处理之后,就可以在第 27 行使用 `axis=(0,1)` 参数,而不会出现维度错误。
完整修改后的代码如下:
```python
import torch
import torch.nn as nn
from torchvision import models, transforms
from PIL import Image
import numpy as np
import matplotlib.pyplot as plt
# Load pre-trained ResNet-18 model
model = models.resnet18(pretrained=True)
# Remove the fully connected layer from the model
model = nn.Sequential(*list(model.children())[:-1])
# Set model to evaluation mode
model.eval()
# Define image transformation to match the pre-processing used during training
transform = transforms.Compose([
transforms.Resize((224, 224)),
transforms.ToTensor(),
transforms.Normalize([0.485, 0.456, 0.406], [0.229, 0.224, 0.225])
])
# Load sample image
img = Image.open('sample_image.jpg')
# Apply transformation and convert to tensor
img_tensor = transform(img).unsqueeze(0)
# Pass image tensor through ResNet-18 to get feature maps
with torch.no_grad():
features = model(img_tensor)
# Reshape features to remove single-dimensional axes
features = features.squeeze()
if len(features.shape) == 3:
features = features.unsqueeze(-1)
if len(features.shape) == 2:
features = features.unsqueeze(-1).unsqueeze(-1)
# Calculate global average pooling of feature maps
pooled_features = np.mean(features, axis=(1,2))
# Reshape pooled features to match spatial dimensions of feature maps
pooled_features = np.repeat(pooled_features, features.shape[1]*features.shape[2]).reshape(features.shape)
# Calculate importance of each feature map by comparing it to the global average
heatmap = np.abs(features - pooled_features)
# Normalize heatmap to range between 0 and 1
heatmap = (heatmap - np.min(heatmap)) / (np.max(heatmap) - np.min(heatmap))
# Plot heatmap on top of original image
plt.imshow(img)
plt.imshow(heatmap.sum(axis=0), alpha=0.5, cmap='jet')
plt.axis('off')
plt.show()
```
希望能对您有所帮助!
阅读全文
相关推荐
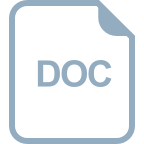
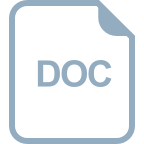
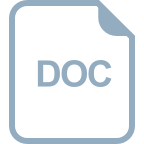
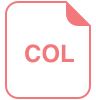
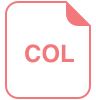
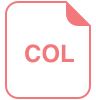
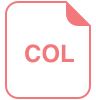
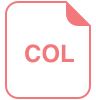
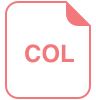
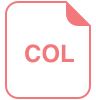
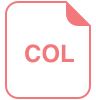
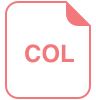
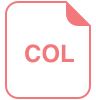



