Application of MATLAB in Engineering Optimization: In-depth Case Studies
发布时间: 2024-09-14 20:41:34 阅读量: 31 订阅数: 24 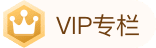
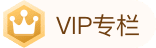
# 1. Introduction to MATLAB and Overview of Engineering Optimization
MATLAB (an abbreviation for Matrix Laboratory) is a high-performance numerical computing environment that integrates numerical analysis, matrix operations, signal processing, and graph display, particularly prominent in the field of engineering optimization. It provides an easy-to-use programming environment for algorithm development, data visualization, data analysis, and numerical computing, enabling engineers and scientists to implement complex numerical computations and scientific graphing by writing scripts or functions.
## 1.1 The Importance of MATLAB in Engineering Optimization
Engineering optimization is an interdisciplinary field that involves mathematics, computer science, engineering, and other knowledge areas. Its purpose is to improve or optimize the design or performance of engineering systems. MATLAB offers powerful tools for engineering optimization, such as built-in algorithms and function libraries, allowing engineers and researchers to quickly solve linear, nonlinear, integer, and constrained optimization problems. The goal of optimization is to find the optimal solution or a set of feasible solutions that, under certain constraints, minimize or maximize the objective function.
## 1.2 Applications of MATLAB in Optimization Problems
In engineering practice, optimization problems can be divided into various types, such as parameter optimization, multi-objective optimization, and global optimization. MATLAB provides rich tools and functions to solve these problems. For example, MATLAB's `fmincon` function can solve optimization problems with linear and nonlinear constraints, while the `ga` function is suitable for solving genetic algorithm optimization problems with global search characteristics. The widespread application of these tools makes MATLAB an indispensable auxiliary tool in the field of engineering optimization.
# 2. Application of Optimization Toolbox in MATLAB
## 2.1 Theoretical Foundation of the Optimization Toolbox
### 2.1.1 Mathematical Modeling of Optimization Problems
In engineering and scientific fields, optimization problems are ubiquitous. To solve these problems using MATLAB's optimization toolbox, a mathematical model must first be established. Mathematical modeling involves transforming real-world problems into mathematical language, including defining the objective function, design variables, and constraints. The objective function is the quantity to be optimized, which can be either maximized or minimized. Design variables are the variables that affect the value of the objective function. Constraints define the feasible region for the design variables.
```plaintext
Objective function: Minimize or Maximize f(x)
Design variables: x = [x1, x2, ..., xn]
Constraints: g(x) <= 0, h(x) = 0
```
By appropriately setting these elements, we can transform various engineering problems into optimization problems. For example, in mechanical design, it may be necessary to minimize material usage (objective function) while satisfying constraints on strength and cost.
### 2.1.2 Basics of Linear and Nonlinear Programming
Linear programming (LP) is one of the most common types of optimization problems, with both the objective function and constraints being linear. Linear programming problems are typically solved using methods such as the simplex method or the interior-point method.
```plaintext
Objective function: Minimize c^T * x
Constraints: A * x <= b
x >= 0
```
Nonlinear programming (NLP), on the other hand, has no such restrictions; the objective function or constraints can be any mathematical function. These problems are generally more complex and require specialized algorithms, such as gradient descent, Newton's method, or quasi-Newton methods, to solve.
```plaintext
Objective function: Minimize f(x)
Constraints: g_i(x) <= 0 (i = 1, ..., m)
h_j(x) = 0 (j = 1, ..., p)
```
MATLAB's optimization toolbox provides various functions to solve these problems, helping users quickly and conveniently find the optimal solutions to problems.
## 2.2 MATLAB Optimization Toolbox Functions
### 2.2.1 fmincon: Nonlinear Constrained Optimization
The `fmincon` function in MATLAB is used to solve nonlinear optimization problems with linear and nonlinear constraints. This function is very powerful, capable of handling both equality and inequality constraints, and supports boundary limits.
```matlab
[x, fval] = fmincon(fun, x0, A, b, Aeq, beq, lb, ub, nonlcon, options)
```
When using `fmincon`, you need to specify the objective function `fun`, the initial point `x0`, the linear equality and inequality constraints `Aeq`, `beq`, `A`, `b`, the lower and upper bounds for the variables `lb` and `ub`, and the nonlinear constraint function `nonlcon`.
#### Example: Solving a Constrained Optimization Problem
Suppose we need to minimize the function `f(x) = x1^2 + x2^2`, subject to the constraints `x1 + x2 >= 1`, `x1^2 + x2 <= 1`, `x1 >= 0`.
```matlab
function f = objfun(x)
f = x(1)^2 + x(2)^2;
end
function [c, ceq] = nonlcon(x)
c = -(x(1) + x(2) - 1); % c <= 0
ceq = x(1)^2 + x(2)^2 - 1; % ceq = 0
end
% Initial point and options setup
x0 = [0, 0];
options = optimoptions('fmincon','Display','iter','Algorithm','sqp');
% Execute optimization
[x, fval] = fmincon(@objfun, x0, [], [], [], [], [], [], @nonlcon, options);
```
In the above code, `objfun` defines the objective function, and `nonlcon` defines the nonlinear constraints. `x0` is the initial point for the optimization, and `options` sets the optimization parameters, such as displaying the iteration process and selecting the algorithm. After executing `fmincon`, `x` and `fval` respectively give the optimal solution and its objective function value.
### 2.2.2 linprog: Solving Linear Programming Problems
The `linprog` function is used to solve linear programming problems. It is also a powerful tool that can solve standard or relaxed forms of linear programming problems.
```matlab
[x, fval] = linprog(f, A, b, Aeq, beq, lb, ub, options)
```
Here, `f` is the coefficient vector of the objective function, `A` and `b` are the coefficients of the inequality constraints, `Aeq` and `beq` are the coefficients of the equality constraints, and `lb` and `ub` are the lower and upper bounds of the variables.
#### Example: Solving a Linear Programming Problem
Assume we need to minimize the function `f(x) = c1*x1 + c2*x2`, subject to the constraints `a11*x1 + a12*x2 <= b1`, `a21*x1 + a22*x2 <= b2`, and `x1 >= 0`, `x2 >= 0`.
```matlab
c = [c1, c2];
A = [a11, a12; a21, a22];
b = [b1; b2];
lb = [0; 0]; % No upper bound
[x, fval] = linprog(c, A, b, [], [], lb);
```
In the above code, `c` is the objective function coefficient vector, and `A` and `b` constitute the inequality constraints. `lb` sets the lower bound of the variables, and there is no upper bound set here, indicating no upper bound. After executing `linprog`, `x` gives the optimal solution to the problem, and `fval` is the corresponding optimal objective function value.
### 2.2.3 ga: Application of Genetic Algorithm in Optimization
The Genetic Algorithm (GA) is a search heuristic algorithm based on the principles of natural selection and genetics. GA solves optimization problems by simulating the process of biological evolution in nature. In MATLAB, the `ga` function implements the genetic algorithm.
```matlab
[x, fval] = ga(fun, nvars, A, b, Aeq, beq, lb, ub, nonlcon, options)
```
When using `ga`, `fun` is the objective function to be minimized, `nvars` is the number of variables, and the other parameters are similar to those of `fmincon`.
#### Example: Solving an Optimization Problem with Genetic Algorithm
Suppose we need to minimize the function `f(x) = x1^2 + x2^2`, and the variables `x1` and `x2` can take values between `[-100, 100]`.
```matlab
function f = objfun(x)
f = x(1)^2 + x(2)^2;
end
nvars = 2;
lb = [-100, -100];
ub = [100, 100];
[x, fval] = ga(@objfun, nvars, [], [], [], [], lb, ub);
```
In the above code, `objfun` defines the objective function, `nvars` specifies the number of variables, and `lb` and `ub` limit the range of variables. The `ga` function will use the genetic algorithm to solve for the optimal solution `x` and the objective function value `fval`.
## 2.3 Practical Case: Using Optimization Toolbox for Design Optimization
### 2.3.1 Case Study of Mechanical Design Optimization
In mechanical design, the optimization toolbox can be used to find the optimal design scheme. For example, we may need to optimize the design parameters of a gearbox to ensure its volume is minimized while meeting the requirements for torque and structural strength.
#### Case Description
Assume we have a gearbox design problem where the goal is to minimize the volume, and the gearbox must be able to transmit a specific torque and meet safety standards for structural strength. The design variables include the size of the gears, the number of teeth, and material properties. The constraints include torque transmission requirements and strength limits.
```plaintext
Objective function: Minimize V(x)
Constraints: g(x) <= 0 (Torque transmission requirements)
h(x) = 0 (Strength limits)
x_min <= x <= x_max
```
#### Solution
Use the `fmincon` function to solve this problem. First, define the objective function and constraint functions, then set the optimization options and start the optimization algorithm.
```matlab
% Define the objective function
function f = volumeFun(x)
% Calculate the volume based on the design parameters of the gearbox
f = ...; % Expression for calculating the volume
end
% Define the nonlinear constraint function
function [c, ceq] = constraintsFun(x)
% Calculate torque transmission and strength limits based on design parameters
c = ...; % Expression for calculating torque transmission limits
ceq = ...; % Expression for calculating strength limits
end
% Optimization parameters
x0 = ...; % Initial values of design variables
options = optimoptions('fmincon','Display','iter','Algorithm','sqp');
% Execute optimization
[x_opt, fval] = fmincon(@volumeFun, x0, [], [], [], [], x_min, x_max, @constraintsFun, options);
```
### 2.3.2 Case Study of Circuit Design Optimization
The optimization toolbox is also applicable in circuit design. For example, assume we need to design a circuit to find the optimal solution that minimizes the total resistance of the circuit while meeting current and voltage requirements.
#### Case Description
Assume we have a circuit design problem where the goal is to minimize the total resistance of the circuit, while ensuring that the circuit can support a specified range of current and voltage. The design variables include the resistance values of the resistors, and the constraints include current and voltage requirements.
```plaintext
Objective function: Minimize R_total(x)
Constraints: I_min <= I(x) <= I_max
V_min <= V(x) <= V_max
```
#### Solution
Use `fmincon` to solve this optimization problem. First, def
0
0