MATLAB's Optimization Applications in Finance: A Detailed Case Study on Portfolio Optimization
发布时间: 2024-09-14 20:54:04 阅读量: 23 订阅数: 42 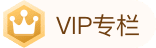
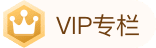
# 1. An Overview of MATLAB's Application in Financial Optimization
MATLAB, as a powerful numerical computing and scientific computing software, plays a crucial role in solving optimization problems in the financial industry. In the field of financial optimization, the scope of MATLAB's applications spans from theoretical research to the formulation of actual investment strategies. Its robust matrix computation capabilities, rich financial libraries, and built-in optimization algorithms enable financial analysts and engineers to quickly implement complex mathematical models and conduct efficient simulations and optimizations.
The application of MATLAB in financial optimization is not limited to the construction of models and simulation of results; it also includes real-time testing of strategies, risk assessment, and predictive analysis. With MATLAB's Financial Toolbox, users can access a vast amount of financial data and use advanced mathematical methods for analysis, which is greatly beneficial for the design of financial products, the management of investment portfolios, and market analysis.
Furthermore, MATLAB's openness and flexibility allow it to be integrated with other programming languages and software platforms, providing greater room for development in financial optimization. For instance, MATLAB can be combined with databases, Excel, C++, and other programming languages to achieve data import and export, algorithm extension, and integration with other systems, thereby offering a comprehensive solution for financial optimization.
# 2.
## 2.1 Basic Operations in MATLAB and Financial Mathematical Theories
### 2.1.1 Introduction to MATLAB's Working Environment
MATLAB (Matrix Laboratory) is a high-performance numerical computing environment and fourth-generation programming language. It integrates data visualization, data analysis, and numerical computing, and is widely used in areas such as engineering computation, control design, signal processing, and financial modeling. The MATLAB working environment includes four main windows: the Command Window, the Editor/Debugger, the Workspace, and the Path. The Command Window is used for inputting and executing commands, the Editor/Debugger is used for writing and debugging scripts and functions, the Workspace displays the variables in the current working environment, and the Path shows the current search path as well as the setting of toolboxes.
```matlab
% A simple example of a MATLAB command
a = 1;
b = 2;
sum = a + b;
disp(sum); % The output result is 3
```
In the code above, we defined two variables `a` and `b`, assigned them values of 1 and 2, respectively, calculated their sum and stored it in the variable `sum`, and finally used the `disp` function to output the result.
### 2.1.2 Basic Operations on Matrices and Arrays
The core of MATLAB is matrices and arrays; it uses matrices as the basic unit for data operations, so the creation and manipulation of matrices are fundamental to MATLAB programming. MATLAB supports a variety of operations on matrices and arrays, such as addition, subtraction, multiplication, division, transposition, and matrix multiplication.
```matlab
% An example of matrix creation and basic operations
A = [1 2; 3 4]; % Create a 2x2 matrix A
B = [5 6; 7 8]; % Create a 2x2 matrix B
% Matrix addition
C = A + B;
% Matrix multiplication
D = A * B;
% Matrix transposition
E = A';
disp(C); % Output the result of matrix C
disp(D); % Output the result of matrix D
disp(E); % Output the result of matrix E
```
In MATLAB, matrix multiplication is performed using the `*` operator, and the transpose operation uses a single quote `'`. After executing the above operations, the results of the two matrices being added, multiplied, and the matrix transposition will be outputted.
## 2.2 Financial Mathematical Basics and Models
### 2.2.1 Theoretical Basis of Investment Portfolios
The theory of portfolio is one of the core contents of modern finance, with the goal of studying how to effectively allocate assets while considering risk and expected returns. Harry Markowitz proposed the Modern Portfolio Theory (MPT) in 1952, which assumes that investors are risk-averse and seek the optimal balance between the expected return of the portfolio and risk.
```matlab
% A simple calculation of the expected return and risk of a portfolio
weights = [0.5, 0.5]; % Assume the weight of two assets is 50% each
expected_returns = [0.1, 0.12]; % The expected returns of two assets
cov_matrix = [0.01, 0; 0, 0.02]; % The return covariance matrix of two assets
% Calculate the expected return of the portfolio
port_return = weights' * expected_returns;
% Calculate the risk of the portfolio (standard deviation)
port_risk = sqrt(weights' * cov_matrix * weights);
disp(['Expected return: ', num2str(port_return)]);
disp(['Portfolio risk: ', num2str(port_risk)]);
```
In the code above, we defined the weights, expected returns, and covariance matrix of two assets and calculated the expected return and risk of the portfolio. This demonstrates the application of MATLAB in simple portfolio analysis.
### 2.2.2 Quantitative Models for Risk and Return
In financial mathematics, ***mon indicators for quantifying risk and return include standard deviation, variance, beta coefficient, and the Sharpe ratio. Investors can use these indicators to assess the risk-return characteristics of assets and make investment decisions.
```matlab
% Calculate the Sharpe ratio using MATLAB
risk_free_rate = 0.03; % The risk-free interest rate
sharp_ratio = (port_return - risk_free_rate) / port_risk;
disp(['Sharpe ratio: ', num2str(sharp_ratio)]);
```
In the code block above, we defined the risk-free interest rate and calculated the Sharpe ratio of the portfolio, which reflects the ratio of the portfolio's excess return to risk and is an important indicator for evaluating investment performance.
### 2.2.3 An Overview of the Markowitz Model
The Markowitz model is the cornerstone of portfolio theory, providing a framework for quantifying investment risk and returns. The model assumes that investors construct optimal portfolios based on the mean-variance criterion and solve for the optimal weight allocation based on this.
```matlab
% Implementing the Markowitz model optimization in MATLAB
% Assume the expected returns and covariance matrix of a set of assets are known
expected_returns = [0.1; 0.12; 0.11; 0.13; 0.15]; % The expected returns of five assets
cov_matrix = [0.01, 0.002, 0.001, 0.0015, 0.001; ... % The return covariance matrix of five assets
0.002, 0.015, 0.0025, 0.0028, 0.0023;
0.001, 0.0025, 0.012, 0.0022, 0.0018;
0.0015, 0.0028, 0.0022, 0.013, 0.0016;
0.001, 0.0023, 0.0018, 0.0016, 0.011];
% Use quadprog to solve the quadratic programming problem, calculating the optimal portfolio for the Markowitz model
options = optimoptions('quadprog','Display','off'); %
```
0
0
相关推荐







