Application of MATLAB Optimization Algorithms in Transportation Logistics: Complete Analysis of Cases and Strategies
发布时间: 2024-09-14 21:07:44 阅读量: 6 订阅数: 18 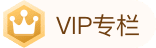
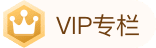
# 1. Basic Concepts of MATLAB Optimization Algorithms
Optimization algorithms are at the core of modern computing technology, involving the search for a set of parameters that optimizes a given performance metric. MATLAB, as a high-performance numerical computing and visualization software, offers a range of toolboxes to support the development and application of optimization algorithms. This chapter will introduce the basic knowledge and applications of MATLAB in optimization problems, laying the foundation for subsequent in-depth analyses on topics such as transportation logistics optimization, path optimization, inventory management, and supply chain network optimization.
## 1.1 Linear Programming and MATLAB
Linear programming is one of the most common types of optimization problems, involving the maximization or minimization of a linear objective function subject to a set of linear inequalities or equalities. The `linprog` function in MATLAB is a key tool for solving linear programming problems, providing a simple and efficient method for their solution.
```matlab
% Example: Linear Programming Problem
c = [-1; -2]; % Coefficients of the objective function
A = [1, 2; 1, -1; -2, 1]; % Coefficients matrix for inequality constraints
b = [2; 2; 3]; % Constants for inequality constraints
lb = zeros(2,1); % Lower bounds for the variables
[x, fval] = linprog(c, A, b, [], [], lb); % Solving the linear programming problem
```
## 1.2 Nonlinear Optimization and MATLAB
Compared to linear problems, solving nonlinear optimization problems is more complex, involving functions that may contain nonlinear terms. MATLAB's `fmincon` function can be used to solve constrained nonlinear optimization problems, employing interior-point methods or sequential quadratic programming to find local optima.
```matlab
% Example: Nonlinear Optimization Problem
x0 = [0.5, 0.5]; % Initial guess values
A = []; b = [];
Aeq = []; beq = [];
lb = [0, 0]; ub = [];
nonlcon = @nonlconfun; % Define the nonlinear constraint function
options = optimoptions('fmincon','Display','iter','Algorithm','sqp');
[x, fval] = fmincon(@objfun, x0, A, b, Aeq, beq, lb, ub, nonlcon, options);
% Define the objective function
function f = objfun(x)
f = (x(1) - 1)^2 + (x(2) - 2)^2;
end
% Define the nonlinear constraint function
function [c, ceq] = nonlconfun(x)
c = x(1)^2 + x(2)^2 - 1;
ceq = [];
end
```
In this chapter, we have introduced the basic concepts of linear and nonlinear optimization problems and their corresponding solving methods in MATLAB, ranging from simple to complex. In the next chapter, we will delve into the issue of transportation logistics optimization, demonstrating MATLAB's significant potential in solving specific industry problems.
# 2. Analysis of Transportation Logistics Optimization Problems
## 2.1 Overview of Logistics Optimization Problems
### 2.1.1 Problem Definition and Importance
Logistics optimization problems focus on how to effectively transport, store, and distribute goods while meeting customer demands and maintaining service quality. These problems usually involve cost minimization, efficiency maximization, and customer satisfaction maximization. In the logistics process, many factors need to be considered, such as transportation costs, warehousing expenses, delivery times, transportation routes, and inventory levels. Logistics optimization can not only save costs for companies but also improve service quality and customer satisfaction, playing a significant role in maintaining competitive advantages in fierce market competition.
### 2.1.2 Limitations of Traditional Logistics Optimization Methods
Traditional logistics optimization methods rely on empirical judgments and simple mathematical models, often unable to solve complex, variable real-world problems. These methods have limitations when dealing with large-scale, multi-constraint problems, for example, inefficiently processing large amounts of data and difficulty in responding to rapidly changing market demands and supply conditions. In addition, traditional optimization methods lack flexibility; when the external environment changes, the cost of readjusting the optimization plan is high and difficult. Therefore, adopting more advanced technologies and algorithms, such as MATLAB optimization toolboxes, for logistics optimization becomes an inevitable trend.
## 2.2 Introduction to MATLAB Optimization Toolbox
### 2.2.1 Overview of Key Functions in the Toolbox
The MATLAB optimization toolbox provides a powerful function library for solving various linear and nonlinear programming problems. Key functions in the toolbox include:
- `linprog`: Used for solving linear programming problems.
- `intlinprog`: Used for solving integer linear programming problems.
- `quadprog`: Used for solving quadratic programming problems.
- `fmincon`: Used for solving constrained nonlinear optimization problems.
- `ga`: A global optimization solver based on genetic algorithms.
These functions are flexible in parameter configuration and can adapt to different types of optimization problems, providing reliable mathematical model support for solving logistics optimization problems.
### 2.2.2 Mathematical Models of Optimization Problems in MATLAB
The MATLAB optimization toolbox abstracts optimization problems into mathematical models, which can be represented as:
```
minimize f(x)
subject to g(x) ≤ 0
A*x = b
Aeq*x = beq
lb ≤ x ≤ ub
x in {ints}
```
Where `f(x)` is the objective function to be minimized, `g(x)` is a series of inequality constraint conditions, `A*x = b` and `Aeq*x = beq` are equality constraint conditions. The range of variable `x` is constrained by the lower bound `lb`, the upper bound `ub`, and the integer set `ints`.
These mathematical models can clearly express various problems in logistics optimization and be solved through MATLAB's optimization functions.
## 2.3 Advantages of Using MATLAB to Solve Transportation Logistics Problems
### 2.3.1 Comparison with Other Programming Languages
Compared with traditional programming languages (such as C/C++ and Java), MATLAB has obvious advantages in numerical computing and visualization. MATLAB comes with a large number of advanced numerical computing functions, allowing users to quickly build prototype models without having to implement complex mathematical algorithms from scratch. At the same time, MATLAB provides rich graphics and visualization tools that can intuitively display optimization results and processes, facilitating analysis and interpretation.
### 2.3.2 Application of MATLAB in Real-World Cases
In actual logistics optimization projects, MATLAB has been widely used. For example, in transportation route optimization, warehouse location selection, and inventory level control, MATLAB can provide accurate mathematical models and efficient algorithm solutions. Through these advantages, companies can adjust strategies in real-time, optimize logistics networks, and improve competitiveness.
In the following chapters, we will delve into how MATLAB solves practical problems in specific areas, such as path optimization and inventory management, as well as the specific applications in supply chain network design.
# 3. Application of MATLAB in Transportation Route Optimization
## 3.1 Theoretical Basis of Route Optimization
Route optimization is a key step in solving transportation logistics problems, with the main goal of finding the lowest cost path under a series of constraint conditions.
### 3.1.1 Shortest Path Problem (SPP)
The Shortest Path Problem (SPP) is one of the classic route optimization problems. It aims to find the shortest path between two nodes and is widely used in road network planning and network communication.
#### Theoretical Analysis
In SPP, there are usually three key elements:
- Node: A point in the network representing a location or a transfer station.
- Edge: A line or curve connecting two nodes, representing a transportation route, which may be weighted to indicate distance or cost.
- Weight: A numerical value on an edge indicating the cost of traveling from one node to another, such as distance, time, or money.
In MATLAB, we can use algorithms from graph theory, such as Dijkstra's algorithm or the Bellman-Ford algorithm, to solve the SPP.
### 3.1.2 Vehicle Routing Problem (VRP)
The Vehicle Routing Problem (VRP) is another more complex route optimization problem. It expands on the concept of the SPP, considering not only the routing of a single vehicle but also the paths and load distribution for multiple vehicles.
#### Theoretical Analysis
The core challenge of VRP lies in:
- Reasonable allocation of distribution centers and customer points.
- Optimizing vehicle transportation routes to reduce travel distance or costs.
- Considering actual constraints such as vehicle capacity and delivery time windows.
Solving VRP usually involves heuristic algorithms, such as genetic algorithms, simulated annealing algorithms, and ant colony algorithms, which can find approximate optimal solutions within acceptable time frames.
## 3.2 MATLAB Implementation of Route Optimiz
0
0