MATLAB Global Optimization Algorithms: An Advanced Journey of Exploration and Practice
发布时间: 2024-09-14 21:05:31 阅读量: 7 订阅数: 18 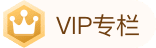
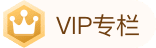
# MATLAB Global Optimization Algorithm: The Journey of Advanced Exploration and Practice
## Introduction
In the fields of IT and engineering, optimization problems are omnipresent, ranging from improving algorithm efficiency to designing new products; optimization techniques are always needed. MATLAB, as a high-performance numerical computing environment, offers a series of powerful tools for solving optimization problems. It is a valuable asset for engineers and researchers.
## Definition of Optimization Problems
Optimization problems generally involve maximizing or minimizing one or more objective functions while satisfying certain constraints. These can range from simple linear optimization problems to extremely complex nonlinear problems.
## MATLAB's Role in Optimization Problems
MATLAB provides a series of built-in functions and toolboxes to solve these optimization problems. From simple linear programming to complex global optimization, MATLAB has a complete set of solutions. These tools can help users quickly build models, verify assumptions, implement algorithms, and ultimately achieve optimization goals.
In this chapter, we will briefly introduce MATLAB's optimization toolbox and how to use MATLAB to solve some basic optimization problems. After studying this chapter, readers will be able to understand the application value of MATLAB in solving optimization problems and how to start using MATLAB for optimization problem solving. The following chapters will further explore the theoretical basis and practical guide of MATLAB optimization algorithms.
# 2. Theoretical Basis of MATLAB Global Optimization Algorithms
## 2.1 Mathematical Modeling of Optimization Problems
### 2.1.1 Objective Functions and Constraints
When modeling global optimization problems, the objective function and constraints are the basic elements of the problem. The objective function can be represented as a mathematical expression, the purpose of which is to measure the performance or benefit of certain variable combinations, usually to be maximized or minimized. In MATLAB, the objective function can be linear or nonlinear, smooth or nonsmooth, continuous or discrete*
***mon types of constraints include equality constraints and inequality constraints. Equality constraints are usually represented in the form of `Ax = b`, while inequality constraints are represented in the form of `C*x <= d`. In MATLAB, the objective function and constraints are defined through function handles, allowing users to flexibly describe complex problems.
When modeling, careful consideration must be given to the mathematical properties of each objective function and constraint because they directly affect the choice and implementation of the global optimization algorithm used.
### 2.1.2 Classification of Optimization Problems
Optimization problems can be classified into various types based on their characteristics and the nature of the objective function. For example:
- **Linear Programming Problem**: Both the objective function and constraints are linear.
- **Nonlinear Programming Problem**: At least one of the objective functions or constraints is nonlinear.
- **Integer Programming Problem**: The problem contains integer variables, usually divided into mixed integer linear programming and mixed integer nonlinear programming.
- **Multi-Objective Optimization Problem**: There are multiple objective functions that need to be optimized simultaneously.
Furthermore, optimization problems can be classified into continuous optimization problems and discrete optimization problems based on the type of variables. MATLAB optimization toolbox provides a rich set of functions to handle different types of problems, allowing users to choose the most appropriate tool to solve specific problems.
## 2.2 Theoretical Framework of Global Optimization Algorithms
### 2.2.1 Deterministic Global Optimization and Stochastic Global Optimization
Global optimization algorithms can be divided into two major categories: deterministic global optimization and stochastic global optimization. Deterministic global optimization algorithms attempt to find the global optimal solution of the problem and ensure the quality of the solution. These algorithms usually require the mathematical properties of the objective function to guarantee the accuracy of the optimization process, such as branch and bound methods and interval methods.
Stochastic global optimization algorithms (also known as metaheuristic algorithms) simulate the heuristic mechanisms of nature to explore the solution space. These algorithms do not guarantee to find the global optimal solution but can usually find a good approximation within a reasonable time, ***mon stochastic global optimization algorithms include genetic algorithms, simulated annealing algorithms, and particle swarm optimization algorithms.
### 2.2.2 Applicability and Selection Criteria
When selecting a global optimization algorithm, several factors need to be considered, including the scale of the problem, complexity, the nature of the objective function, and requirements for the quality of the solution and computation time. For small-scale or well-behaved mathematical problems, deterministic global optimization algorithms may be more appropriate; for large-scale or mathematically difficult-to-obtain problems, stochastic global optimization algorithms are more suitable.
MATLAB optimization toolbox provides a wide range of algorithm choices, allowing users to select the most appropriate global optimization algorithm based on the characteristics and requirements of the problem. In practice, it may be necessary to try multiple algorithms and determine the optimal algorithm choice by comparing their performance.
## 2.3 Optimization Function Library in MATLAB
### 2.3.1 Introduction to Built-in Functions like fmincon, fminsearch, etc.
MATLAB provides several built-in optimization functions to support the solution of various optimization problems. For example:
- `fmincon`: Used to solve nonlinear programming problems with linear and nonlinear constraints.
- `fminsearch`: Used to solve unconstrained multivariable problems, using the simplex method.
- `ga`: Genetic algorithm optimizer, used to find the global optimal solution.
- `simulannealbnd`: Simulated annealing algorithm, suitable for large-scale global optimization problems.
These functions usually require users to provide handles to the objective function and constraints, making them flexible for various problems.
### 2.3.2 Other Functions of the Optimization Toolbox
In addition to providing a series of optimization functions, MATLAB optimization toolbox includes some auxiliary functions, such as:
- Numerical solving environment for optimization problems (e.g., `optimoptions`, `optimset`);
- Visualization tools (e.g., `optimtool`, `contour`, `surface`, etc.);
- Flexible algorithm options settings, which can be customized through `optimoptions`.
These functions help users better set up optimization problems, interpret results, and adjust algorithm parameters to achieve better performance.
Through the introduction of the above chapters, we have outlined the mathematical modeling basis of MATLAB optimization problems and explored different types of global optimization algorithms, as well as the composition and characteristics of MATLAB's optimization function library. This lays the theoretical foundation for the practical guides and specific application cases in subsequent chapters.
# 3. Practical Guide to MATLAB Global Optimization Algorithms
## 3.1 Algorithm Selection and Parameter Adjustment
### 3.1.1 How to Choose Optimization Algorithms Based on Problem Characteristics
When solving real-world problems, choosing the appropriate global optimization algorithm is crucial as it directly affects optimization efficiency and the accuracy of the results. When facing an optimization problem, it is necessary to first clarify the scale of the problem (the number of variables), complexity (the degree of nonlinearity of the objective function and constraints), and whether gradient information is available.
1. For small-scale, low-complexity, and smooth objective functions and constraints optimization problems, traditional gradient descent or quasi-Newton methods, etc., are often more effective.
2. For large-scale or highly nonlinear optimization problems, especially when there are multiple local minima, using stochastic global optimization algorithms such as simulated annealing, genetic algorithms, or particle swarm optimization may be a better choice.
3. When there is insufficient gradient information for the problem, derivative-based global optimization methods such as GlobalSearch or PatternSearch can be considered. These methods do not require gradient information and are suitable for black-box optimization problems.
### 3.1.2 Strategies and Techniques for Parameter Settings
The performance of optimization algorithms largely depends on parameter settings. Taking genetic algorithms as an example, their main parameters include population size, crossover probability, mutation probability, etc. Choosing the correct parameter combination is crucial for the convergence speed and the quality of the final solution of the algorithm. Here are some strategies for setting parameters:
1. **Population Size**: A larger population helps maintain diversity but increases computational costs. Usually, the optimal value needs to be determined through experiments.
2. **Crossover Probability**: A higher crossover probability can promote the exploration of the solution space, but too high a crossover probability may make the algorithm too random.
3. **Mutation Probability**: An appropriate mutation probability can prevent the algorithm from converging too early to local minima, but too high
0
0
相关推荐
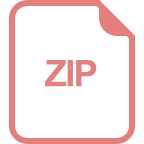
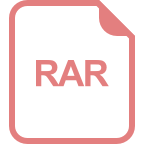
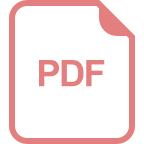





