如果我想要一个最大池化操作,输入为3x3, 池化的窗口为2x2,输出为2x2,求pytorch代码
时间: 2023-04-06 09:03:18 浏览: 96
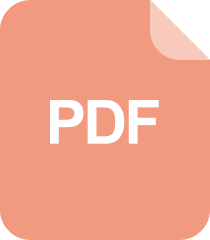
MaxPooling 最大池化简单实现
可以使用torch.nn.MaxPool2d函数实现最大池化操作,代码如下:
import torch
input_tensor = torch.randn(1, 1, 3, 3) # 输入为1个通道,大小为3x3的张量
max_pool = torch.nn.MaxPool2d(kernel_size=2, stride=2) # 池化窗口为2x2,步长为2
output_tensor = max_pool(input_tensor) # 输出为1个通道,大小为2x2的张量
print(output_tensor) # 输出结果为大小为2x2的张量
阅读全文
相关推荐
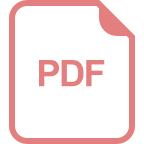

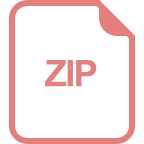
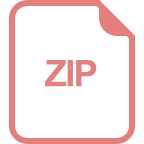
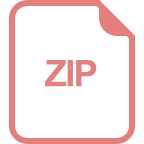
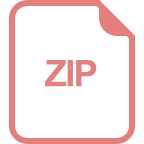
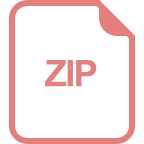
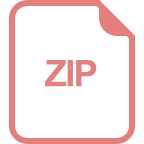








