翻译一下这串代码 class TAttention(nn.Module): def __init__(self, dim, heads=8, dim_head=64, dropout=0.): super().__init__() inner_dim = dim_head * heads project_out = not (heads == 1 and dim_head == dim) self.heads = heads self.scale = dim_head ** -0.5 self.attend = nn.Softmax(dim=-1) self.to_qkv = nn.Linear(dim, inner_dim * 3, bias=False) self.to_out = nn.Sequential( nn.Linear(inner_dim, dim), nn.Dropout(dropout) ) if project_out else nn.Identity()
时间: 2024-04-02 09:35:58 浏览: 149
这段代码定义了一个名为 TAttention 的类,它继承自 nn.Module。构造函数中有四个参数,分别是 dim、heads、dim_head 和 dropout。其中 dim 表示输入的特征维度,heads 表示注意力头数,dim_head 表示每个注意力头的维度,dropout 表示 dropout 的概率。
在构造函数中,首先根据 heads 和 dim_head 计算得到内部维度 inner_dim,并判断是否需要通过投影将内部维度变为 dim。然后定义了 heads 和缩放因子 scale,同时定义了使用 Softmax 计算注意力分布的层 attend,以及将输入转化为查询、键、值三个部分的线性层 to_qkv。最后定义了一个线性层 to_out 用于将注意力计算结果转换为最终输出,如果需要投影则使用 nn.Linear,否则使用 nn.Identity。其中线性层 to_out 的结构为:先通过 nn.Linear 将内部维度的特征转换为 dim 维,然后通过一个 dropout 层进行正则化。
相关问题
class MultiHeadGraphAttention(torch.nn.Module): def __init__(self, num_heads, dim_in, dim_k, dim_v): super(MultiHeadGraphAttention, self).__init__() #"dim_k and dim_v must be multiple of num_heads" assert dim_k % num_heads == 0 and dim_v % num_heads == 0 self.num_heads = num_heads self.dim_in = dim_in self.dim_k = dim_k self.dim_v = dim_v self.linear_q = torch.nn.Linear(dim_in, dim_k, bias=False) self.linear_k = torch.nn.Linear(dim_in, dim_k, bias=False) self.linear_v = torch.nn.Linear(dim_in, dim_v, bias=False) self.leaky_relu = torch.nn.LeakyReLU(negative_slope=0.2) self._nor_fact = 1 / sqrt(dim_k // num_heads)
这是一个实现多头图注意力机制的 PyTorch 模块。该模块将输入的节点特征矩阵作为 Q(查询)、K(键)和 V(值)三个线性变换的输入,并将其分别映射为 dim_k、dim_k 和 dim_v 维的特征矩阵。然后,将这些特征矩阵按照 num_heads 头进行切分,每个头的维度为 dim_k/num_heads 和 dim_v/num_heads,然后进行注意力计算。最后将每个头的结果拼接在一起,经过一次线性变换输出。其中,_nor_fact 是一个归一化因子,用于控制注意力的大小。
需要注意的是,这个模块只处理了节点之间的注意力计算,如果要考虑边上的权重信息,还需要在输入特征矩阵中加入边的特征信息,并在计算注意力时将其考虑进去。
class DoubleFastRCNNOutputLayers(nn.Module): def __init__( self, cfg, input_size, num_classes, cls_agnostic_bbox_reg, box_dim=4 ): super(DoubleFastRCNNOutputLayers, self).__init__() if not isinstance(input_size, int): input_size = np.prod(input_size) self.cls_score = nn.Linear(input_size, num_classes + 1) num_bbox_reg_classes = 1 if cls_agnostic_bbox_reg else num_classes self.bbox_pred = nn.Linear(input_size, num_bbox_reg_classes * box_dim) nn.init.normal_(self.cls_score.weight, std=0.01) nn.init.normal_(self.bbox_pred.weight, std=0.001) for l in [self.cls_score, self.bbox_pred]: nn.init.constant_(l.bias, 0) self._do_cls_dropout = cfg.MODEL.ROI_HEADS.CLS_DROPOUT self._dropout_ratio = cfg.MODEL.ROI_HEADS.DROPOUT_RATIO def forward(self, x_s, x_l): if x_s.dim() > 2: x_s = torch.flatten(x_s, start_dim=1) if x_l.dim() > 2: x_l = torch.flatten(x_l, start_dim=1) proposal_deltas = self.bbox_pred(x_l) if self._do_cls_dropout: x_s = F.dropout(x_s, self._dropout_ratio, training=self.training) scores = self.cls_score(x_s) return scores, proposal_deltas
这段代码是一个双输入的Fast R-CNN输出层的实现,其中包括一个分类得分层和一个边界框回归层。它接受两个输入x_s和x_l,分别代表短边和长边的特征。在前向传播时,它首先对输入进行扁平化处理,然后通过bbox_pred层获得边界框预测值,通过cls_score层获得分类得分。在进行分类得分的计算时,可以进行dropout操作来防止过拟合。最终,返回分类得分和边界框预测值。
阅读全文
相关推荐
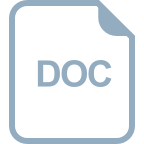
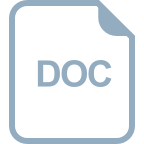
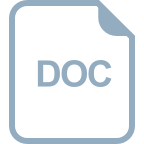














