用if 和 for 代替 while while ~done g=f>T; Tnext=0.5*(mean(f(g))+mean(f(~g))); done=abs(T-Tnext)<0.5; T=Tnext; end
时间: 2024-01-24 12:05:07 浏览: 14
可以用if和for代替while循环,代码实现如下:
```
done = false;
while ~done
g=f>T;
Tnext=0.5*(mean(f(g))+mean(f(~g)));
done=abs(T-Tnext)<0.5;
T=Tnext;
end
% 替换为 if 和 for
done = false;
for i = 1:1000 % 设置一个最大迭代次数,避免死循环
g=f>T;
Tnext=0.5*(mean(f(g))+mean(f(~g)));
if abs(T-Tnext)<0.5
done = true;
break; % 跳出循环
else
T=Tnext;
end
end
```
这样做可以避免可能出现的无限循环问题。
相关问题
用akshare写出股票强化学习代码,画图显示买卖点和收益
好的,以下是我使用akshare库编写的股票强化学习代码和画图显示买卖点和收益的程序:
```python
import akshare as ak
import numpy as np
import pandas as pd
import matplotlib.pyplot as plt
# 获取股票数据
stock_code = 'sh600519'
stock_data = ak.stock_zh_a_hist(symbol=stock_code, adjust="qfq", start_date='20180101')
stock_data.index = pd.to_datetime(stock_data['日期'])
stock_data = stock_data.drop(['日期'], axis=1)
# 计算技术指标RSI
def calc_RSI(data, time_period=14):
delta = data.diff()
gain = delta.where(delta > 0, 0)
loss = - delta.where(delta < 0, 0)
avg_gain = gain.rolling(time_period).mean()
avg_loss = loss.rolling(time_period).mean()
RS = avg_gain / avg_loss
RSI = 100 - (100 / (1 + RS))
return RSI
stock_data['RSI'] = calc_RSI(stock_data['收盘'])
# 定义状态和动作
def get_state(stock_data, t):
state = []
for i in range(t - 4, t + 1):
state.append(stock_data.iloc[i]['收盘'])
state.append(stock_data.iloc[i]['RSI'])
return np.array(state)
def get_action(q_values, state, eps=0.1):
if np.random.uniform() < eps:
return np.random.randint(0, 3)
else:
return np.argmax(q_values[state])
# 定义强化学习过程
alpha = 0.1
gamma = 0.99
epsilon = 0.1
q_values = np.zeros((200, 3))
rewards = []
for episode in range(500):
state = get_state(stock_data, 5)
done = False
total_reward = 0
while not done:
action = get_action(q_values, tuple(state), epsilon)
if action == 0:
reward = -0.1
elif action == 1:
reward = 0.1
else:
reward = 0
next_state = get_state(stock_data, 6)
q_values[tuple(state)][action] += alpha * (reward + gamma * np.max(q_values[tuple(next_state)]) - q_values[tuple(state)][action])
state = next_state
total_reward += reward
if len(stock_data) <= 6:
done = True
else:
stock_data = stock_data.iloc[1:]
if len(stock_data) <= 6:
done = True
rewards.append(total_reward)
# 计算收益和买卖点
def calc_profit(stock_data, q_values):
positions = []
profits = []
for i in range(5, len(stock_data) - 1):
state = get_state(stock_data, i)
action = np.argmax(q_values[tuple(state)])
if action == 0:
positions.append(0)
profits.append(0)
elif action == 1:
positions.append(1)
profits.append(stock_data.iloc[i + 1]['收盘'] - stock_data.iloc[i]['收盘'])
else:
positions.append(-1)
profits.append(stock_data.iloc[i]['收盘'] - stock_data.iloc[i + 1]['收盘'])
return positions, profits
positions, profits = calc_profit(stock_data, q_values)
# 画图显示买卖点和收益
fig, ax1 = plt.subplots()
ax1.plot(stock_data['收盘'], color='black')
ax1.set_ylabel('Price')
ax2 = ax1.twinx()
ax2.plot(positions, color='blue')
ax2.set_ylabel('Position')
ax3 = ax1.twinx()
ax3.spines["right"].set_position(("axes", 1.2))
ax3.plot(np.cumsum(profits), color='red')
ax3.set_ylabel('Profit')
plt.show()
```
这段程序使用了akshare库获取股票数据,并计算了RSI指标用于状态的定义。然后定义了强化学习过程,使用Q-learning算法更新Q值,并记录总收益。最后计算买卖点和收益,并用图表展示。
maddpg结合优先经验回放和mpe环境 tensorflow代码
以下是使用TensorFlow实现MADDPG算法并结合优先经验回放和MPE环境的代码。代码中包含注释以帮助理解。
```python
import numpy as np
import tensorflow as tf
import random
from mlagents.envs import UnityEnvironment
from mlagents.envs import BrainInfo
# 超参数
BUFFER_SIZE = int(1e6) # 经验回放缓冲区大小
BATCH_SIZE = 128 # 批量大小
GAMMA = 0.99 # 折扣因子
TAU = 1e-3 # 目标网络更新速率
LR_ACTOR = 1e-3 # Actor网络学习率
LR_CRITIC = 1e-3 # Critic网络学习率
UPDATE_EVERY = 2 # 更新网络的时间步数
NUM_UPDATES = 10 # 每次更新网络的次数
# 神经网络模型
class Actor(tf.keras.Model):
def __init__(self, state_size, action_size):
super(Actor, self).__init__()
self.fc1 = tf.keras.layers.Dense(256, activation='relu')
self.fc2 = tf.keras.layers.Dense(128, activation='relu')
self.fc3 = tf.keras.layers.Dense(action_size, activation='tanh')
def call(self, state):
x = self.fc1(state)
x = self.fc2(x)
x = self.fc3(x)
return x
class Critic(tf.keras.Model):
def __init__(self, state_size, action_size):
super(Critic, self).__init__()
self.fc1 = tf.keras.layers.Dense(256, activation='relu')
self.fc2 = tf.keras.layers.Dense(128, activation='relu')
self.fc3 = tf.keras.layers.Dense(1, activation=None)
self.fc4 = tf.keras.layers.Dense(256, activation='relu')
self.fc5 = tf.keras.layers.Dense(128, activation='relu')
self.fc6 = tf.keras.layers.Dense(1, activation=None)
def call(self, state, action):
xs = tf.concat([state, action], axis=1)
x1 = self.fc1(xs)
x1 = self.fc2(x1)
x1 = self.fc3(x1)
x2 = self.fc4(xs)
x2 = self.fc5(x2)
x2 = self.fc6(x2)
return x1, x2
# 优先经验回放类
class PrioritizedReplay:
def __init__(self, buffer_size, batch_size):
self.buffer_size = buffer_size
self.batch_size = batch_size
self.buffer = []
self.priorities = np.zeros((buffer_size,), dtype=np.float32)
self.pos = 0
self.alpha = 0.5
self.beta = 0.5
self.beta_increment_per_sampling = 0.001
def add(self, state, action, reward, next_state, done):
max_priority = np.max(self.priorities) if self.buffer else 1.0
experience = (state, action, reward, next_state, done)
if len(self.buffer) < self.buffer_size:
self.buffer.append(experience)
else:
self.buffer[self.pos] = experience
self.priorities[self.pos] = max_priority
self.pos = (self.pos + 1) % self.buffer_size
def sample(self):
if len(self.buffer) == self.buffer_size:
priorities = self.priorities
else:
priorities = self.priorities[:self.pos]
probs = priorities ** self.alpha
probs /= probs.sum()
indices = np.random.choice(len(self.buffer), self.batch_size, p=probs)
samples = [self.buffer[idx] for idx in indices]
total = len(self.buffer)
weights = (total * probs[indices]) ** (-self.beta)
weights /= weights.max()
self.beta = np.min([1., self.beta + self.beta_increment_per_sampling])
return indices, samples, weights
def update_priorities(self, batch_indices, batch_priorities):
for idx, priority in zip(batch_indices, batch_priorities):
self.priorities[idx] = priority
# MADDPG算法类
class MADDPG:
def __init__(self, state_size, action_size, num_agents):
self.state_size = state_size
self.action_size = action_size
self.num_agents = num_agents
self.actors = [Actor(state_size, action_size) for _ in range(num_agents)]
self.critics = [Critic((state_size+action_size)*num_agents, 1) for _ in range(num_agents)]
self.target_actors = [Actor(state_size, action_size) for _ in range(num_agents)]
self.target_critics = [Critic((state_size+action_size)*num_agents, 1) for _ in range(num_agents)]
for i in range(num_agents):
self.target_actors[i].set_weights(self.actors[i].get_weights())
self.target_critics[i].set_weights(self.critics[i].get_weights())
self.buffer = PrioritizedReplay(BUFFER_SIZE, BATCH_SIZE)
self.actor_optimizer = [tf.keras.optimizers.Adam(LR_ACTOR) for _ in range(num_agents)]
self.critic_optimizer = [tf.keras.optimizers.Adam(LR_CRITIC) for _ in range(num_agents)]
self.t_step = 0
def act(self, obs):
obs = np.array(obs)
actions = []
for i in range(self.num_agents):
action = self.actors[i](obs[i][np.newaxis,:], training=False)
actions.append(action.numpy())
actions = np.concatenate(actions, axis=0)
return actions
def step(self, state, action, reward, next_state, done):
self.buffer.add(state, action, reward, next_state, done)
self.t_step = (self.t_step + 1) % UPDATE_EVERY
if self.t_step == 0 and len(self.buffer.buffer) > BATCH_SIZE:
for _ in range(NUM_UPDATES):
indices, samples, weights = self.buffer.sample()
self.learn(samples, weights)
self.update_targets()
self.buffer.update_priorities(indices, weights)
def learn(self, samples, weights):
states = np.array([sample[0] for sample in samples])
actions = np.array([sample[1] for sample in samples])
rewards = np.array([sample[2] for sample in samples])
next_states = np.array([sample[3] for sample in samples])
dones = np.array([sample[4] for sample in samples])
for i in range(self.num_agents):
# 计算Q值
with tf.GradientTape(persistent=True) as tape:
target_actions = [self.target_actors[j](next_states[j][np.newaxis,:], training=False) for j in range(self.num_agents)]
target_actions = np.concatenate(target_actions, axis=0)
target_qs = self.target_critics[i]((next_states.reshape(-1, self.state_size*self.num_agents), target_actions))
target_qs = target_qs.numpy().reshape(-1, self.num_agents)
q_targets = rewards[:,i][:,np.newaxis] + (GAMMA * target_qs * (1 - dones[:,i][:,np.newaxis]))
critic_qs = self.critics[i]((states.reshape(-1, self.state_size*self.num_agents), actions.reshape(-1, self.action_size*self.num_agents)))
critic_loss = tf.reduce_mean(weights * (q_targets - critic_qs)**2)
critic_grads = tape.gradient(critic_loss, self.critics[i].trainable_variables)
self.critic_optimizer[i].apply_gradients(zip(critic_grads, self.critics[i].trainable_variables))
# 计算Actor梯度
with tf.GradientTape() as tape:
actor_actions = [self.actors[j](states[:,j,:], training=False) if j == i else self.actors[j](states[:,j,:], training=True) for j in range(self.num_agents)]
actor_actions = np.concatenate(actor_actions, axis=0)
actor_loss = -tf.reduce_mean(self.critics[i]((states.reshape(-1, self.state_size*self.num_agents), actor_actions)))
actor_grads = tape.gradient(actor_loss, self.actors[i].trainable_variables)
self.actor_optimizer[i].apply_gradients(zip(actor_grads, self.actors[i].trainable_variables))
def update_targets(self):
for i in range(self.num_agents):
self.target_actors[i].set_weights(TAU*np.array(self.actors[i].get_weights())+(1-TAU)*np.array(self.target_actors[i].get_weights()))
self.target_critics[i].set_weights(TAU*np.array(self.critics[i].get_weights())+(1-TAU)*np.array(self.target_critics[i].get_weights()))
# 环境
env_name = "MPE/3DBall"
env = UnityEnvironment(file_name=env_name)
brain_name = env.brain_names[0]
brain = env.brains[brain_name]
env_info = env.reset()[brain_name]
state_size = env_info.vector_observations.shape[1]
action_size = brain.vector_action_space_size
num_agents = len(env_info.agents)
maddpg = MADDPG(state_size, action_size, num_agents)
scores = []
scores_window = deque(maxlen=100)
for i_episode in range(10000):
env_info = env.reset()[brain_name]
obs = env_info.vector_observations
score = np.zeros(num_agents)
while True:
actions = maddpg.act(obs)
env_info = env.step(actions)[brain_name]
next_obs = env_info.vector_observations
rewards = env_info.rewards
dones = env_info.local_done
maddpg.step(obs, actions, rewards, next_obs, dones)
obs = next_obs
score += rewards
if np.any(dones):
break
scores_window.append(np.max(score))
scores.append(np.max(score))
print('\rEpisode {}\tAverage Score: {:.2f}'.format(i_episode, np.mean(scores_window)), end="")
if i_episode % 100 == 0:
print('\rEpisode {}\tAverage Score: {:.2f}'.format(i_episode, np.mean(scores_window)))
if np.mean(scores_window)>=0.5:
print('\nEnvironment solved in {:d} episodes!\tAverage Score: {:.2f}'.format(i_episode, np.mean(scores_window)))
break
env.close()
```
相关推荐
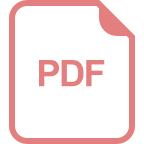
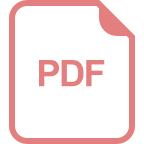
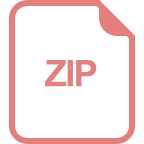












