MATLAB Matrix Indexing Tips: 7 Methods for Flexible Access to Matrix Elements
发布时间: 2024-09-15 01:21:23 阅读量: 42 订阅数: 39 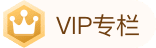
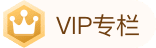
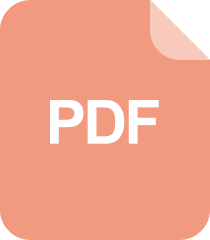
Tutorial on matrix indexing in MATLAB
# 1. Overview of MATLAB Matrix Indexing
MATLAB matrix indexing is a powerful tool for accessing and manipulating elements within a matrix. It offers a variety of indexing methods to extract, replace, or operate on data within a matrix based on specific conditions or patterns. These methods include single-element indexing, multi-element indexing, advanced indexing, and logical indexing. They can flexibly handle matrices of different dimensions and perform a wide range of data manipulation tasks.
# 2. Single-Element Indexing
Single-element indexing is used to access individual elements within a matrix. It employs a pair of parentheses `()`, within which one or more index values are placed.
### 2.1 Linear Indexing
Linear indexing uses a single numeric index to access elements within a matrix. Index values start at 1, representing the first row and first column of the matrix. For example, the following code accesses the first element of matrix `A`:
```matlab
A = [1 2 3; 4 5 6; 7 8 9];
element = A(1, 1); % element = 1
```
### 2.2 Logical Indexing
Logical indexing uses boolean values (`true` or `false`) to select elements within a matrix. Boolean values can be generated by relational operators (such as `>`, `<`, `==`). For instance, the following code uses logical indexing to select elements in matrix `A` that are greater than 5:
```matlab
A = [1 2 3; 4 5 6; 7 8 9];
B = A > 5; % B = [false false false; false true true; true true true]
selected_elements = A(B); % selected_elements = [6 7 8 9]
```
**Line-by-line code logic explanation:**
1. `A > 5`: Compares each element in matrix `A` to 5, resulting in a boolean matrix `B`. The `true` elements in `B` indicate elements greater than 5.
2. `A(B)`: Uses the boolean matrix `B` as an index to select elements from matrix `A` that satisfy the condition.
# 3. Multi-Element Indexing
### 3.1 Colon Indexing
Colon indexing uses the colon (`:`) symbol to specify a range of elements. It can be used to index rows, columns, or the entire matrix.
**Syntax:**
```
matrix(start:end)
matrix(start:step:end)
```
**Parameters:**
* `start`: Starting index
* `end`: Ending index
* `step`: Step size (optional)
**Examples:**
```matlab
% Extract the first two rows of the matrix
matrix(1:2, :)
% Extract the first two rows and the first three columns
matrix(1:2, 1:3)
% Extract the even-numbered rows of the matrix
matrix(2:2:end, :)
% Extract the odd-numbered columns of the matrix
matrix(:, 1:2:end)
```
### 3.2 Comma Indexing
Comma indexing uses the comma (`,`) symbol to specify multiple elements or ranges of elements. It can be used to index rows, columns, or the entire matrix.
**Syntax:**
```
matrix(index1, index2, ..., indexN)
```
**Parameters:**
* `index1`, `index2`, ..., `indexN`: Elements or ranges of elements to be indexed
**Examples:**
```matlab
% Extract the 1st, 3rd, and 5th rows of the matrix
matrix([1, 3, 5], :)
% Extract the 2nd, 4th, and 6th columns of the matrix
matrix(:, [2, 4, 6])
% Extract elements from the 1st row, 2nd column and the 3rd row, 4th column of the matrix
matrix([1, 3], [2, 4])
```
### 3.3 Individual Indexing
Individual indexing uses a single index value to access a single element. It can be used to index rows, columns, or the entire matrix.
**Syntax:**
```
matrix(index)
```
**Parameters:**
* `index`: Index value of the element to be indexed
**Examples:**
```matlab
% Extract the element from the 2nd row, 3rd column of the matrix
matrix(2, 3)
% Extract the first element from the 4th row of the matrix
matrix(4, 1)
% Extract the last element of the matrix
matrix(end)
```
### Logical Indexing
Logical indexing uses logical expressions to choose elements that satisfy the condition. It can be used to index rows, columns, or the entire matrix.
**Syntax:**
```
matrix(logicalExpression)
```
**Parameters:**
* `logicalExpression`: Logical expression used to select elements
**Examples:**
```matlab
% Extract elements greater than 5 from the matrix
matrix(matrix > 5)
% Extract even elements from the matrix
matrix(mod(matrix, 2) == 0)
% Extract elements from the first two rows of the matrix
matrix(1:2, :)
```
### Cell Indexing
Cell indexing uses cell arrays to specify elements to be indexed. It can be used to index rows, columns, or the entire matrix.
**Syntax:**
```
matrix{index1, index2, ..., indexN}
```
**Parameters:**
* `index1`, `index2`, ..., `indexN`: Cell indices of the elements to be indexed
**Examples:**
```matlab
% Extract the element from the 1st row, 2nd column of the matrix
matrix{1, 2}
% Extract elements from the 2nd, 4th, and 6th rows of the matrix
matrix{2:2:6, :}
% Extract elements from the 1st, 3rd, and 5th columns of the matrix
matrix{:, [1, 3, 5]}
```
### Structure Indexing
Structure indexing uses structure field names to specify elements to be indexed. It can be used to index field values within a structure array.
**Syntax:**
```
structure.(fieldName)
```
**Parameters:**
* `fieldName`: Name of the structure field to be indexed
**Examples:**
```matlab
% Extract the values of all 'name' fields from the structure array
names = {structure.name};
% Extract the 'age' field value from the 2nd element of the structure array
age = structure(2).age;
% Extract the values of all 'address' fields from the structure array
addresses = {structure.address};
```
# 4. Advanced Indexing
### 4.1 Boolean Indexing
Boolean indexing uses logical values (true or false) to select elements within a matrix. It allows for the extraction of specific elements from a matrix based on conditions.
**Syntax:**
```matlab
logical_index = logical_expression;
indexed_matrix = matrix(logical_index);
```
**Parameters explanation:**
* `logical_expression`: A logical expression that returns boolean values, used to determine which elements to extract.
* `matrix`: The matrix to be indexed.
* `indexed_matrix`: A new matrix containing elements that satisfy the logical expression.
**Examples:**
```matlab
% Create a matrix
A = [1 2 3; 4 5 6; 7 8 9];
% Use boolean indexing to extract elements greater than 5
logical_index = A > 5;
indexed_matrix = A(logical_index);
% Print the matrix after indexing
disp(indexed_matrix)
```
**Output:**
```
6
7
8
9
```
### 4.2 Cell Indexing
Cell indexing uses cell arrays to choose elements within a matrix. A cell array is an array that contains other arrays.
**Syntax:**
```matlab
cell_index = {row_index, column_index};
indexed_matrix = matrix(cell_index);
```
**Parameters explanation:**
* `row_index`: A vector specifying the row indices to be extracted.
* `column_index`: A vector specifying the column indices to be extracted.
* `matrix`: The matrix to be indexed.
* `indexed_matrix`: A new matrix containing the specified cell elements.
**Examples:**
```matlab
% Create a matrix
A = [1 2 3; 4 5 6; 7 8 9];
% Use cell indexing to extract the element from the 2nd row and 3rd column
cell_index = {2, 3};
indexed_matrix = A(cell_index);
% Print the matrix after indexing
disp(indexed_matrix)
```
**Output:**
```
6
```
### 4.3 Structure Indexing
Structure indexing uses structure field names to select elements within a matrix. A structure is a collection of different types of data.
**Syntax:**
```matlab
struct_index = struct_field_name;
indexed_matrix = matrix.(struct_index);
```
**Parameters explanation:**
* `struct_field_name`: The name of the structure field to be extracted.
* `matrix`: The matrix to be indexed.
* `indexed_matrix`: A new matrix containing the specified structure field elements.
**Examples:**
```matlab
% Create a structure
my_struct = struct('name', {'John', 'Mary', 'Bob'}, 'age', [25, 30, 35]);
% Use structure indexing to extract all ages
age_index = 'age';
age_data = my_struct.(age_index);
% Print the data after indexing
disp(age_data)
```
**Output:**
```
25
30
35
```
# 5.1 Extraction and Replacement of Matrix Elements
Indexing in MATLAB can be used not only to retrieve matrix elements but also to modify them.
**Element Extraction**
Using linear indexing or logical indexing, one or multiple matrix elements can be extracted. For example:
```
% Create a matrix
A = [1 2 3; 4 5 6; 7 8 9];
% Use linear indexing to extract the element from the 2nd row, 3rd column
element = A(2, 3);
% Use logical indexing to extract all elements greater than 5
elements = A(A > 5);
```
**Element Replacement**
Similarly, linear indexing or logical indexing can be used to replace matrix elements. For example:
```
% Use linear indexing to replace the element in the 1st row, 2nd column with 10
A(1, 2) = 10;
% Use logical indexing to replace all elements greater than 5 with 0
A(A > 5) = 0;
```
**注意事项:**
* Indexing beyond the matrix's range will result in an error.
* When replacing elements, the number of new elements must match the number of elements being replaced.
* When replacing elements using logical indexing, the value of the new elements will be applied to all elements that satisfy the condition.
0
0
相关推荐







