Application of OpenCV and Python Versions in Machine Learning: Version Selection and Performance Optimization, Enhancing Model Effectiveness
发布时间: 2024-09-14 16:56:25 阅读量: 30 订阅数: 42 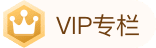
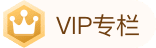
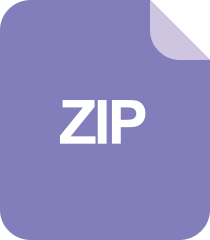
python-for-computer-vision-with-opencv-and-deep-learning
# 1. Overview of OpenCV and Python Applications in Machine Learning
OpenCV (Open Source Computer Vision Library) is an open-source computer vision library, and Python is a popular high-level programming language. Their combination provides powerful tools for image processing and computer vision tasks in machine learning.
OpenCV offers a wide range of image processing and computer vision algorithms, including image reading, transformation, resizing, rotating, segmentation, object detection, feature extraction, and matching. These algorithms can be used for image preprocessing, feature extraction and representation, providing useful input for machine learning models.
Python offers easy-to-use syntax, a wealth of libraries, and extensive community support. It enables developers to rapidly prototype and deploy machine learning models and access its powerful image processing features through the OpenCV API.
# ***parative Analysis of OpenCV and Python Versions
### 2.1 Differences in Features and Functionality
#### 2.1.1 Image Processing and Computer Vision Algorithms
Both OpenCV and Python versions provide a rich set of image processing and computer vision algorithms, ranging from basic operations (such as image reading, transformation, and display) to advanced techniques (such as image segmentation, object detection, and feature matching). However, there are some differences in the implementation and support of these algorithms:
- **OpenCV:** Focuses on the low-level implementation of image processing and computer vision algorithms, providing efficient C++ libraries. Its algorithm library is very extensive, covering various fields from image processing to machine learning.
- **Python Version:** Provides access to the OpenCV library via the OpenCV-Python bindings, allowing its use in a Python environment. While it offers similar functionality to OpenCV, its algorithm implementations may rely on underlying C++ libraries, which could affect performance and flexibility.
#### 2.1.2 Support for Machine Learning and Deep Learning
Both OpenCV and Python versions support machine learning and deep learning tasks, but they differ in focus and functionality:
- **OpenCV:** Primarily focuses on computer vision tasks and offers limited support for machine learning and deep learning. It includes some basic machine learning algorithms, such as Support Vector Machines and Decision Trees, but lacks native support for deep learning models.
- **Python Version:** Provides more comprehensive support for machine learning and deep learning by integrating third-party libraries such as Scikit-learn, TensorFlow, and PyTorch. This allows the Python version to handle a broader range of machine learning and deep learning tasks, including image classification, object detection, and natural language processing.
### 2.2 Strategies for Performance Optimization
#### 2.2.1 Code Optimization Techniques
- **OpenCV:** Offers various code optimization techniques, including the use of OpenMP for multi-threaded parallel processing, SIMD instruction sets for vectorized computations, and optimized memory management.
- **Python Version:** Can optimize code performance by using libraries like NumPy and SciPy for vectorized computations, employing multi-threading and multiprocessing for parallel processing, and optimizing data structures.
#### 2.2.2 Hardware Acceleration and Parallel Computing
- **OpenCV:** Supports hardware accelerators such as GPUs and FPGAs for parallel computing, significantly enhancing the performance of image processing and computer vision tasks.
- **Python Version:** Also supports hardware acceleration by integrating libraries like CUDA and OpenCL. However, its parallel computing capabilities may be limited by the underlying Python interpreter and the implementation of third-party libraries.
# 3. Guideli
0
0
相关推荐






